SATR: Semantics-Aware Triadic Refinement network for referring image segmentation
KNOWLEDGE-BASED SYSTEMS(2024)
摘要
Referring image segmentation (RIS) is a fundamental cross-modal task that aims to predict the pixel-level segmentation mask of the target referred by a natural language expression. Existing methods usually focus on leveraging word-to-pixel interaction mechanisms to directly generate final masks, while they ignore semantic alignment between the query and visual context, as well as rich fine-grained spatial details of the referred object, resulting in inaccurate identification, blurry boundary, and missing small objects.To address these issues, we propose a Semantics-Aware Triadic Refinement (SATR) network for referring image segmentation. Specifically, to bridge the gap between visual and linguistic modalities, we propose a Language-Guided Pixel Modulation (LGPM), which utilizes word-and sentence-level features to facilitate word -to-pixel interaction and sentence-to-object alignment, respectively. Meanwhile, the LGPM is plugged into an off-the-shelf pre-trained visual backbone to jointly learn and extract multi-modal features, which avoids the tedious learning phase of low-level features from scratch. In addition, without any post-processing for refining the final mask, we design a triadic refinement decoder to selectively extract and aggregate salient object features, pixel-level details, and boundary information, which preserves the rich spatial features to generate a high-quality mask. Further, we use a multi-task strategy to capture the target-specific context during training. Experimental results demonstrate the proposed method performs favorably against the previous approaches on the challenging RefCOCO, RefCOCO+, and RefCOCOg datasets.
更多查看译文
关键词
Referring image segmentation,Triadic Refinement network,Language-guided pixel modulation,Multi-task learning,Boundary enhancement
AI 理解论文
溯源树
样例
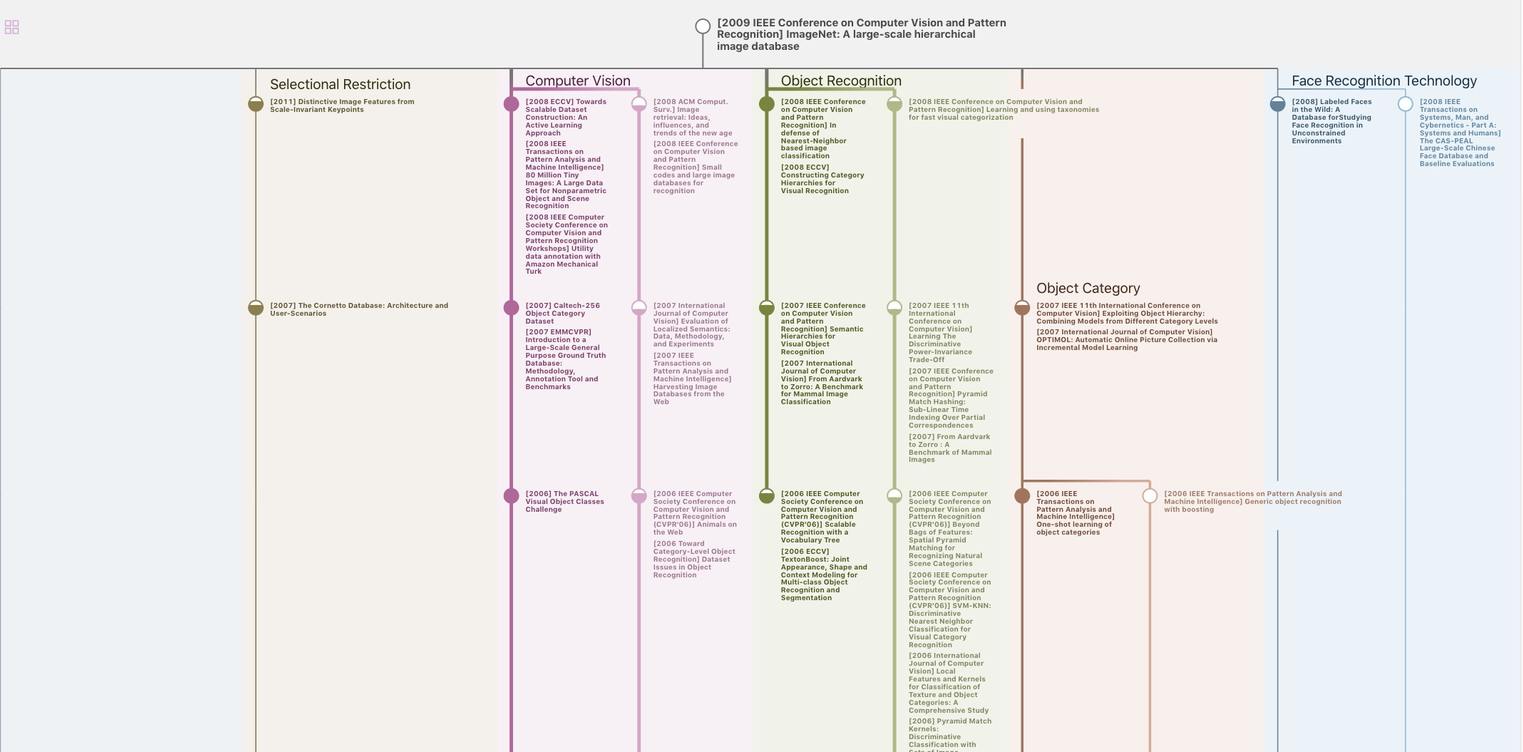
生成溯源树,研究论文发展脉络
Chat Paper
正在生成论文摘要