FlexNet: A warm start method for deep reinforcement learning in hybrid electric vehicle energy management applications
ENERGY(2024)
摘要
Deep reinforcement learning (DRL) has been widely studied in the energy management of hybrid electric vehicles (HEVs) for its remarkable energy efficiency improvement compared to conventional methods. However, how to alleviate the time consumption of training a stable reinforcement learning agent still needs to be solved in real -world implementation. This study presents a human expert knowledge encoded 'warm start' method with the flexibility to change the neural network architecture. The expert knowledge is encoded in a decision tree which then initializes the weights and bias of the DRL neural network. Compared with another fixed architecture warm start method, the proposed FlexNet exhibits improved learning speed by 60.8 % and 88.8 % in action space 50 and 100, respectively. The energy consumption by the proposed FlexNet EMS method is 12.2 % and 6.4 % better than rule-based and equivalent consumption minimization strategy, respectively. This proposed warm start method can reduce learning time and increase energy efficiency in various energy management applications.
更多查看译文
关键词
Hybrid electric vehicle,Deep reinforcement learning,Warm start,Expert knowledge,Energy management strategy
AI 理解论文
溯源树
样例
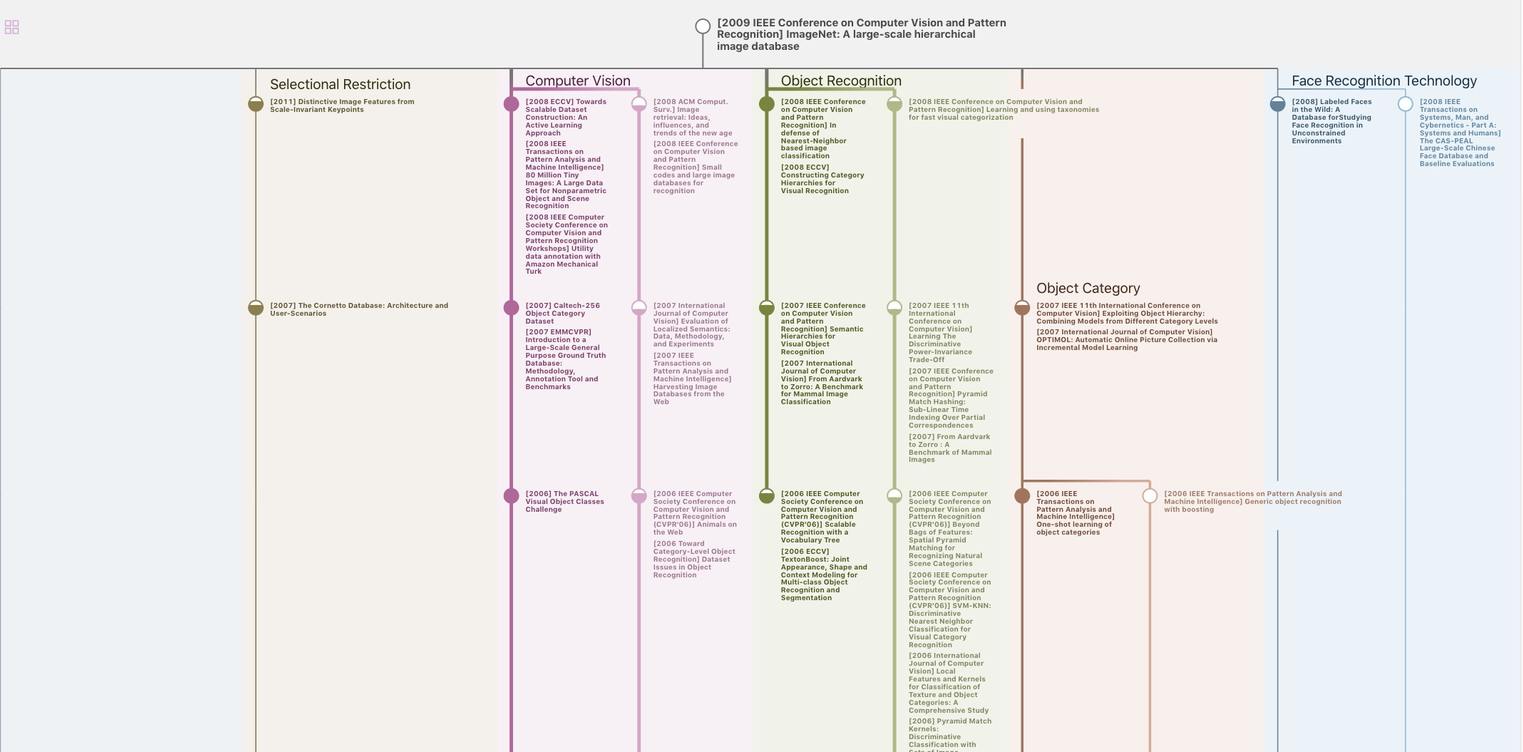
生成溯源树,研究论文发展脉络
Chat Paper
正在生成论文摘要