SVD-AE: An asymmetric autoencoder with SVD regularization for multivariate time series anomaly detection
NEURAL NETWORKS(2024)
摘要
Anomaly detection in multivariate time series is of critical importance in many real-world applications, such as system maintenance and Internet monitoring. In this article, we propose a novel unsupervised framework called SVD-AE to conduct anomaly detection in multivariate time series. The core idea is to fuse the strengths of both SVD and autoencoder to fully capture complex normal patterns in multivariate time series. An asymmetric autoencoder architecture is proposed, where two encoders are used to capture features in time and variable dimensions and a shared decoder is used to generate reconstructions based on latent representations from both dimensions. A new regularization based on singular value decomposition theory is designed to force each encoder to learn features in the corresponding axis with mathematical supports delivered. A specific loss component is further proposed to align Fourier coefficients of inputs and reconstructions. It can preserve details of original inputs, leading to enhanced feature learning capability of the model. Extensive experiments on three real world datasets demonstrate the proposed algorithm can achieve better performance on multivariate time series anomaly detection tasks under highly unbalanced scenarios compared with baseline algorithms.
更多查看译文
关键词
Anomaly detection,Multivariate time series,Singular value decomposition,Autoencoder regularization,Fourier coefficients alignment
AI 理解论文
溯源树
样例
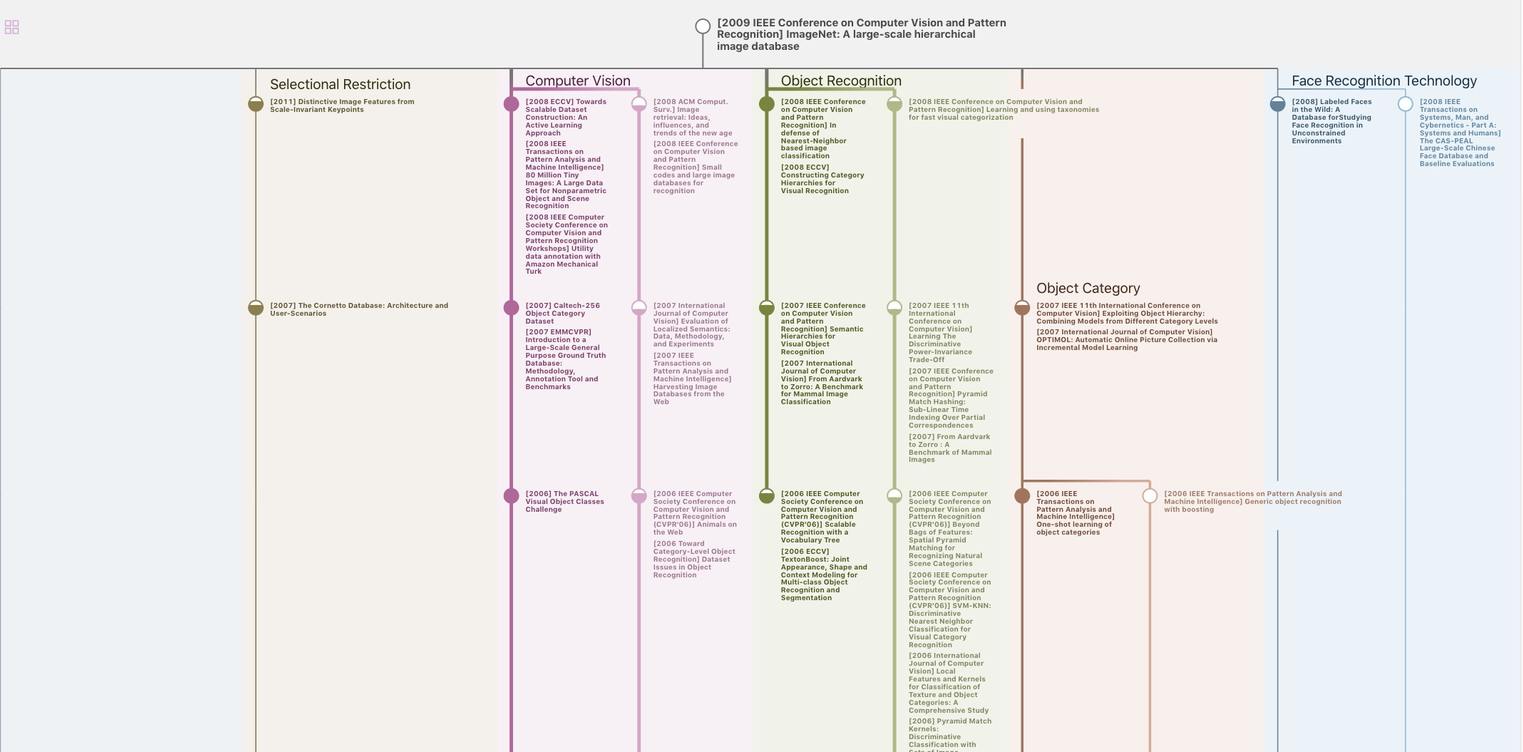
生成溯源树,研究论文发展脉络
Chat Paper
正在生成论文摘要