LoRa-based outdoor localization and tracking using unsupervised symbolization
INTERNET OF THINGS(2024)
Abstract
This paper proposes a long-range (LoRa)-based outdoor localization and tracking method. Our method presents an unsupervised localization approach that utilizes symbolized LoRa received signal features, such as RSSI, SNR, and path loss, where each symbol represents a system state. To identify the partitioning boundaries between the symbols in time series, we employ maximum entropy partitioning. The D-Markov machine is used to construct nondeterministic finite-state automata for extracting temporal patterns. We incorporate the Chinese restaurant process for online estimation, especially in scenarios with an unbounded number of probable areas around each LoRa gateway. An adaptive trilateration approach is then used to localize the target node from the estimated ranged radii of areas. The point-wise localization data was used for time-series continuous tracking. We collected a dataset using three LoRaWAN gateways, sensor nodes powered by single-use batteries, and a Chirpstack server on a sports oval. We thoroughly evaluated the proposed method from the perspectives of localization accuracy and tracking capability. Our method outperformed state-of-the-art machine learning-driven range-based and fingerprint-based localization techniques.
MoreTranslated text
Key words
LoRa localization,RSSI,Path loss,D-Markov machine,Chinese restaurant process,Maximum entropy partitioning
AI Read Science
Must-Reading Tree
Example
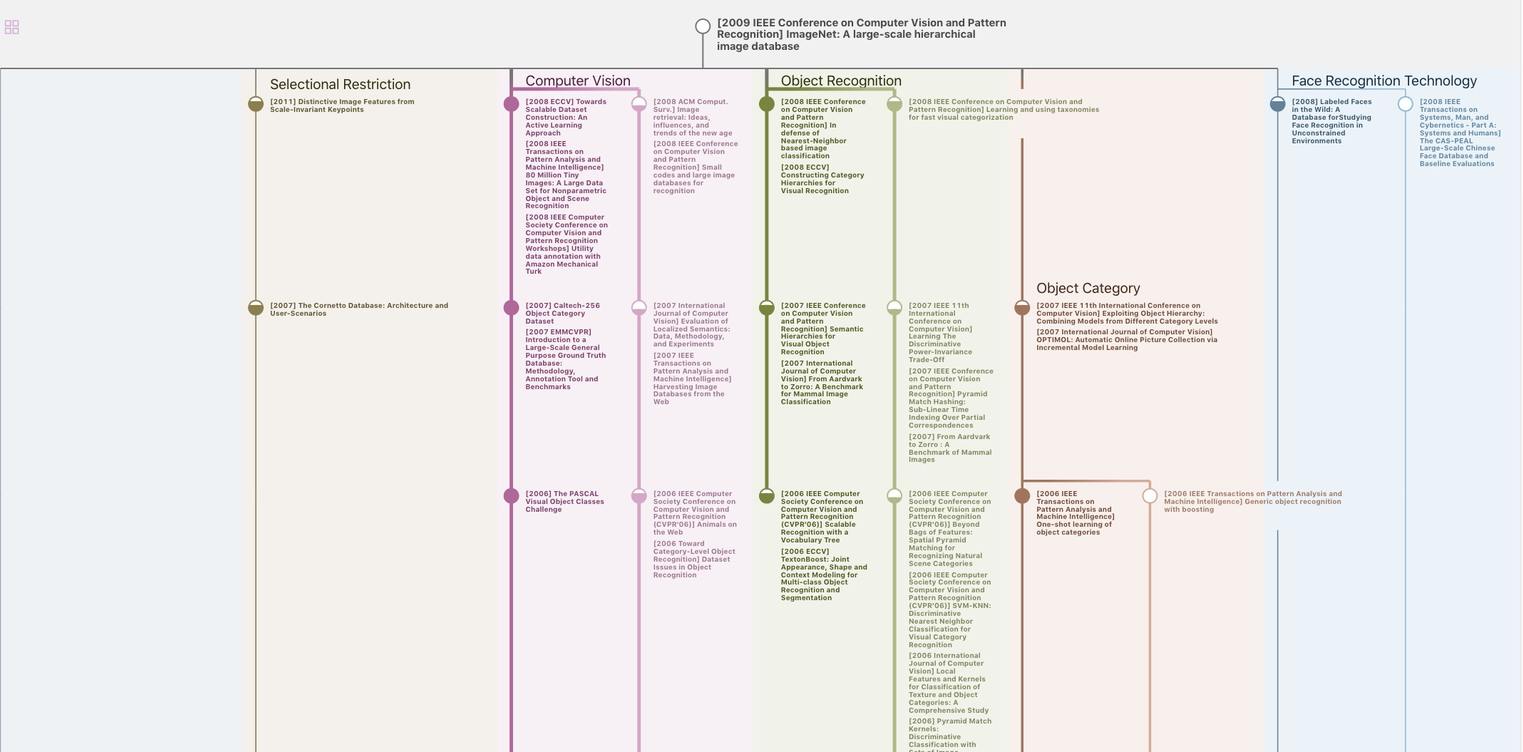
Generate MRT to find the research sequence of this paper
Chat Paper
Summary is being generated by the instructions you defined