A coarse-to-fine pattern parser for mitigating the issue of drastic imbalance in pixel distribution
PATTERN RECOGNITION(2024)
Abstract
The significance of minute semantic components such as eyes and eyebrows tends to be overshadowed by larger components like skin and background, leading to inadequate graph attention from the model. Recent croppingand-segmenting strategies comprise distinct stages and do not involve extensive interactions, thereby preventing joint optimization for collaborative perception. To mitigate this flaw, a coarse-to-fine pattern parsing network (CtFPPN) is proposed based on the capsule network (CapsNet). The CtFPPN incorporates a coarse-grained parser module, which generates binary coarse-scaled masks for larger components, and a fine-grained parser module, which performs fine-scaled parsing for smaller components using the coarse contexts as references. To establish a connection between two parsers, the discretization attention fragmentation mechanism (DAFM) is customized. The fine-grained parser has the option to aggregate projections from non-spatially-fixed collections of the coarsegrained parser, thereby embodying the principle of "coarse-to-fine" of CtFPPN. Under the premise of the existence of imbalanced pixel distribution, quantitative and ablation experiments of face and human parsing demonstrate the superiority of CtFPPN over the state-of-the-arts. Notably, CtFPPN excels in mitigating the pixel imbalance issues and accurately defining fine-scaled semantic boundaries of minuscule components.
MoreTranslated text
Key words
Pattern parsing,Unbalanced distribution of pixels,Graph attention,Capsule network
AI Read Science
Must-Reading Tree
Example
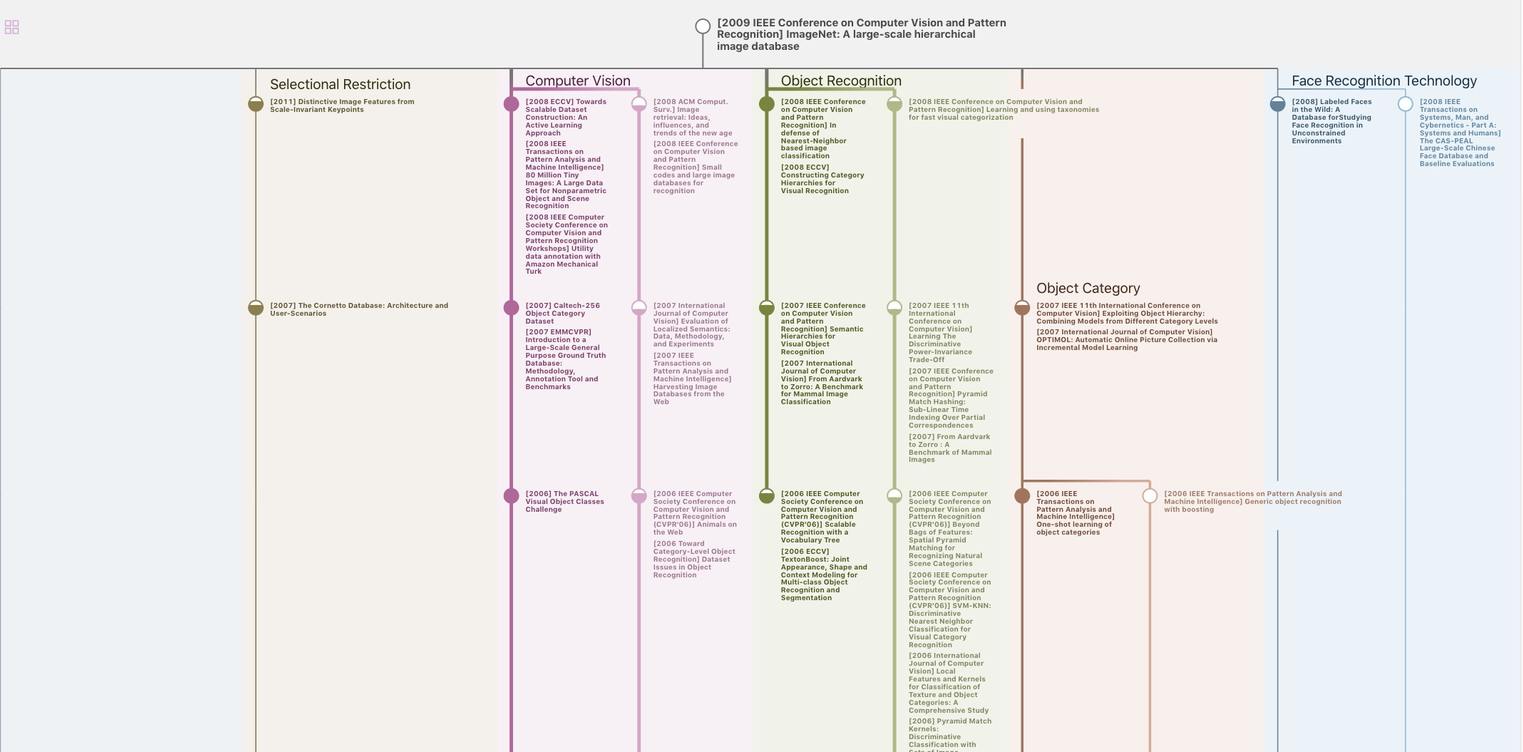
Generate MRT to find the research sequence of this paper
Chat Paper
Summary is being generated by the instructions you defined