Tail behavior of ACD models and consequences for likelihood-based estimation
JOURNAL OF ECONOMETRICS(2024)
摘要
We establish new results for estimation and inference in financial durations models, where events are observed over a given time span, such as a trading day, or a week. For the classical autoregressive conditional duration (ACD) models by Engle and Russell (1998), we show that the large sample behavior of likelihood estimators is highly sensitive to the tail behavior of the financial durations. In particular, even under stationarity, asymptotic normality breaks down for tail indices smaller than one or, equivalently, when the clustering behavior of the observed events is such that the unconditional distribution of the durations has no finite mean. Instead, we find that estimators are mixed Gaussian and have non-standard rates of convergence. The results are based on exploiting the crucial fact that for duration data the number of observations within any given time span is random. Our results apply to general econometric models where the number of observed events is random.
更多查看译文
关键词
Financial durations,Autoregressive conditional duration (ACD),Tail index,Quasi maximum likelihood,Mixed normality
AI 理解论文
溯源树
样例
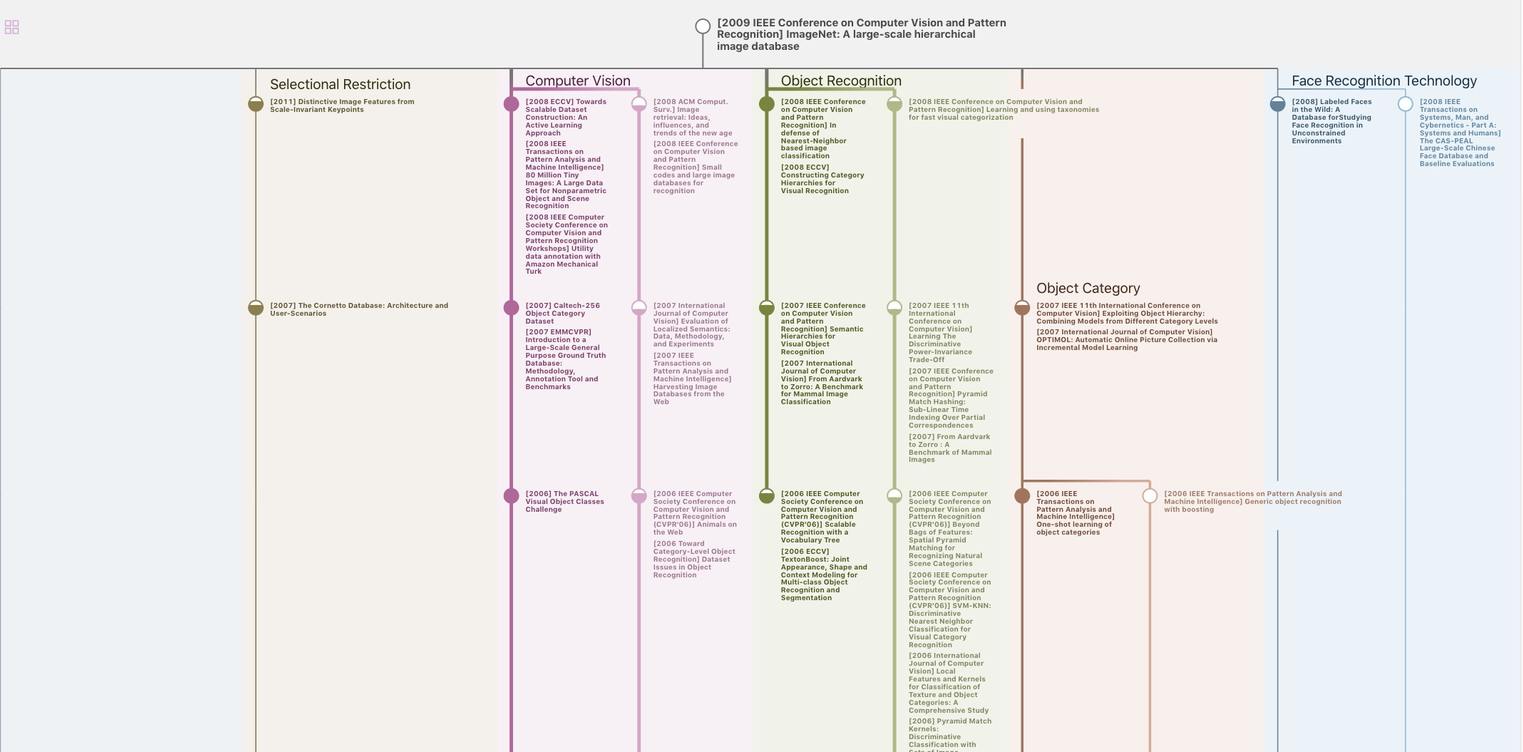
生成溯源树,研究论文发展脉络
Chat Paper
正在生成论文摘要