Stable approximate Q-learning under discounted cost for data-based adaptive tracking control
NEUROCOMPUTING(2024)
摘要
In this paper, the stability of tracking error dynamics under the data-based discounted iterative Q-learning is investigated. First, a novel performance index with a discount factor is introduced into the iterative Q-learning based tracking control. Then, considering the approximation errors caused by the Q-function approximator, the finite error bound between the iterative and optimal Q-functions is established. Moreover, based on the new stability analysis, the selection rule of the discount factor is developed, which ensures that the corresponding optimal control policy is admissible. Next, to ensure the stability of the tracking error dynamics under iterative control policies, the stability condition about the approximate Q-function is established. It is guaranteed that iterative control policies derived from the critic network drive the tracking error to zero. Additionally, considering the adopted policy function approximator, the upper bound function of the approximation errors is developed. It is ensured that the trained action network stabilizes the tracking error dynamics. Finally, a simulation example is utilized to implement the data-based discounted iterative Q-learning and verify the present theoretical results.
更多查看译文
关键词
Adaptive dynamic programming,Discrete-time nonlinear systems,Discounted value iteration,Q-learning,Stability analysis,Tracking control
AI 理解论文
溯源树
样例
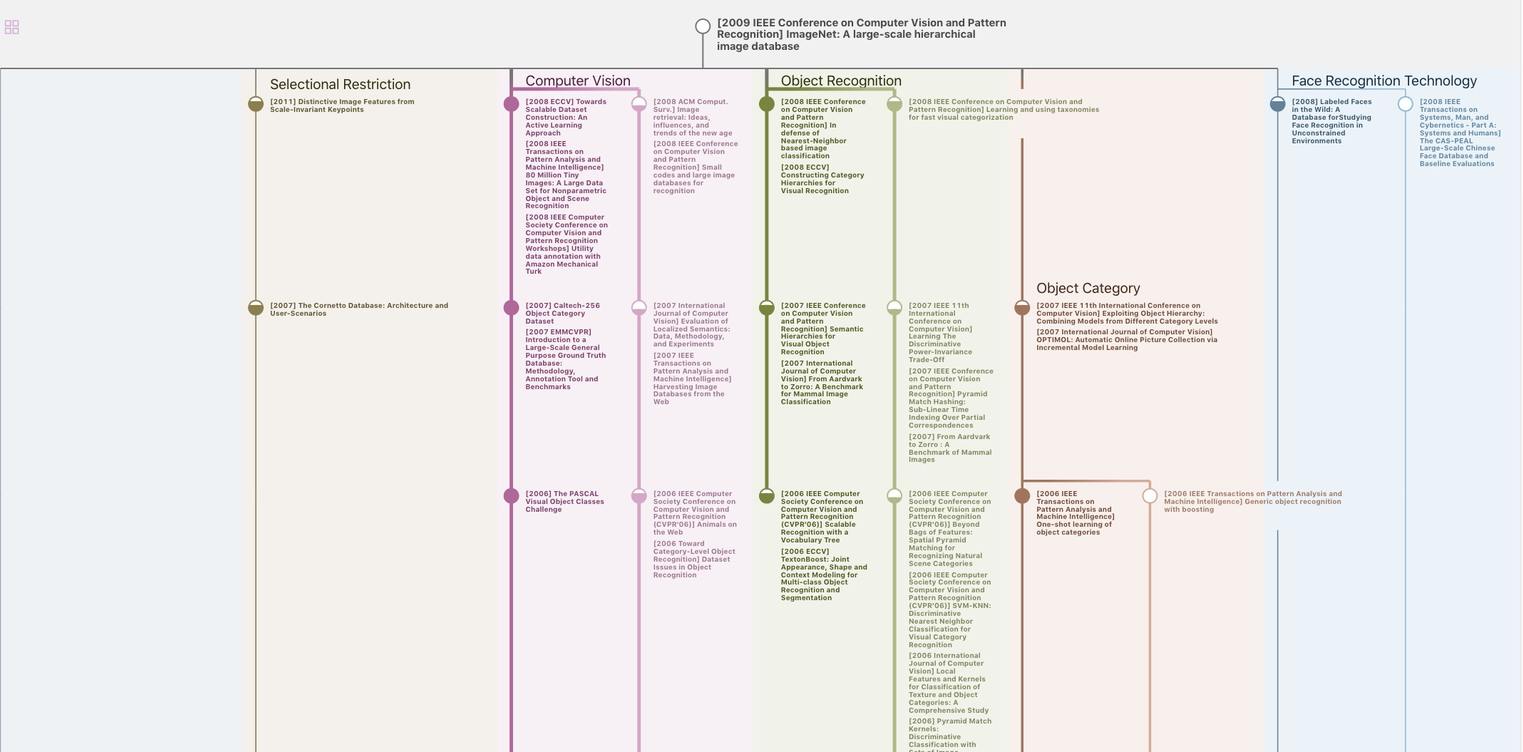
生成溯源树,研究论文发展脉络
Chat Paper
正在生成论文摘要