LDD-Net: Lightweight printed circuit board defect detection network fusing multi-scale features
ENGINEERING APPLICATIONS OF ARTIFICIAL INTELLIGENCE(2024)
摘要
The current printed circuit board (PCB) defect detection model is difficult to balance accuracy and compu-tational cost and cannot satisfy the requirements of practical applications. In this regard, a lightweight PCB image defect detection network (LDD-Net) with high accuracy is proposed in this study. First, LDD-Net designs a novel lightweight feature extraction network (LFEN), which adds convolutional layers for extracting large-scale feature maps and uses an efficient downsampling method to extract richer defect features. LFEN not only greatly reduces resource requirements, but also improves detection accuracy. Second, LDD-Net devises multi-scale aggregation network (MAN) to facilitate information sharing among feature maps at different scales. In addition, MAN incorporates an efficient attention module to suppress similar background interference. Finally, LDD-Net uses the lightweight decoupling head to detect three groups of shallow feature maps with rich information of the small object. Experimental results on the PCB defect dataset ,and VisDrone public dataset show that the mean average precision (mAP), recall, and precision of LDD-Net outperform state-of-the-art lightweight object detection models. The mAP, computation, number of parameters, and detection speed of LDD-Net are 95.90%, 21.48 G, 5.13 M, and 78.13 frames per second, respectively. LDD-Net effectively balances accuracy, computational cost, and detection speed for applications in edge systems or resource-constrained embedded devices.
更多查看译文
关键词
Deep learning,Lightweight detection network,PCB defect detection,Small object detection
AI 理解论文
溯源树
样例
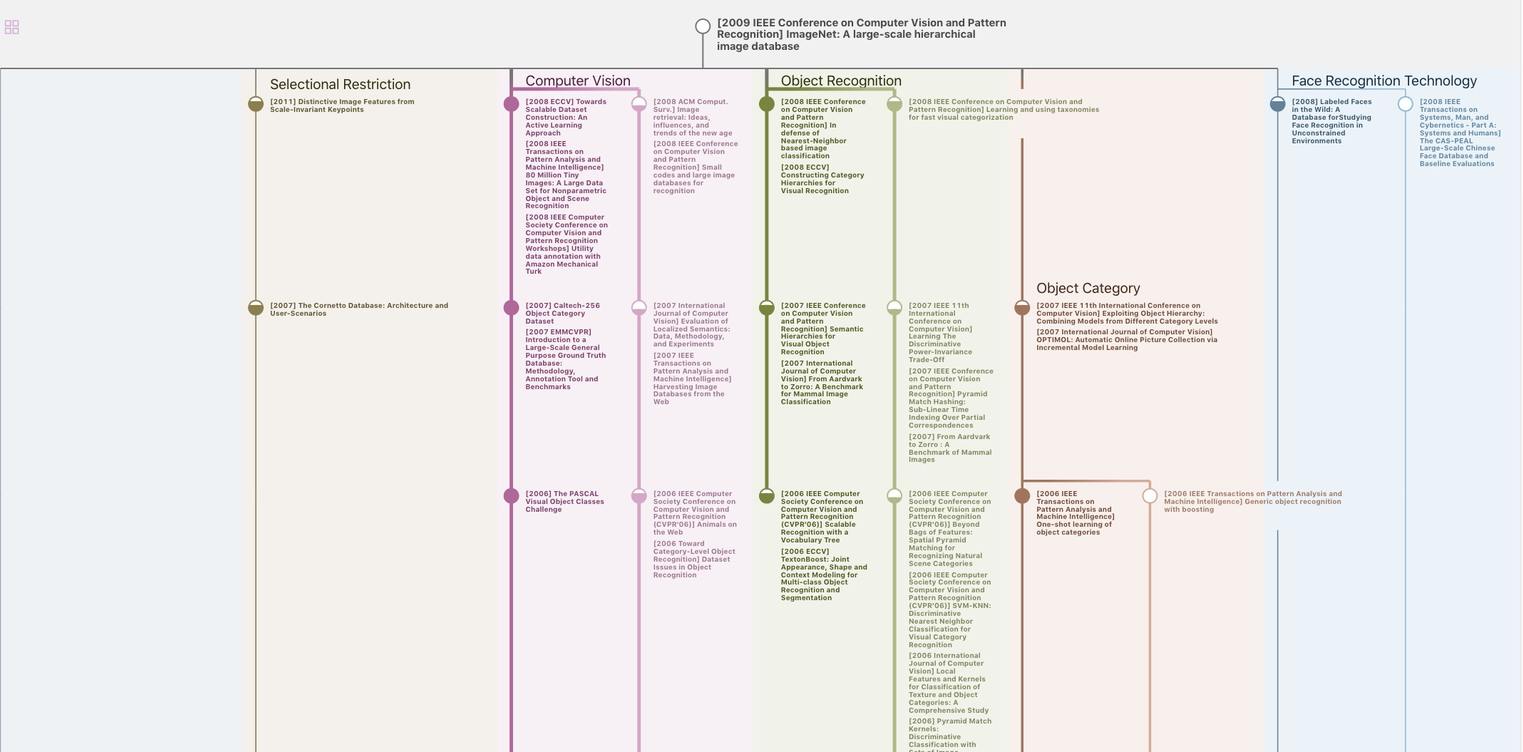
生成溯源树,研究论文发展脉络
Chat Paper
正在生成论文摘要