Multi-label arrhythmia classification using 12-lead ECG based on lead feature guide network
ENGINEERING APPLICATIONS OF ARTIFICIAL INTELLIGENCE(2024)
摘要
Background: Multi-label arrhythmia classification plays a crucial role in the prevention and diagnosis of cardiac diseases. Intelligent analysis of multi-lead electrocardiogram (ECG) signals is the main method of classification. Existing methods have shown that different lead ECG signals contribute differently to the classification network. Methods: In this paper, we propose a Lead Feature Guide Network (LFG-Net) to improve the feature representation capability of the network by focusing on the lead features with high contribution. Firstly, the lead features with high contributions are selected by the Shapley Additive exPlanations (SHAP) method. Secondly, the lead features are utilized to guide the classification model in bridging the gap between the selected features and the classification model by minimizing the difference in their losses. Finally, the network improves the accuracy of arrhythmia classification due to the extraction of more valid feature information.Conclusion: The results demonstrated that the F1-score reached 0.842 for classification on the China Physiological Signal Challenge (CPSC) 2018 dataset, which indicated the network outperformed other state-of-the-art methods. The parameters of the network during prediction are 1.02 M. Through experiments, it can be verified that the effectiveness of the proposed method on arrhythmia classification with multi-label, and it can also be demonstrated that the lead features with high contributions can improve the network classification accuracy.
更多查看译文
关键词
A rrhythmia classification,Deep learning,12-Lead ECG,Multi-label
AI 理解论文
溯源树
样例
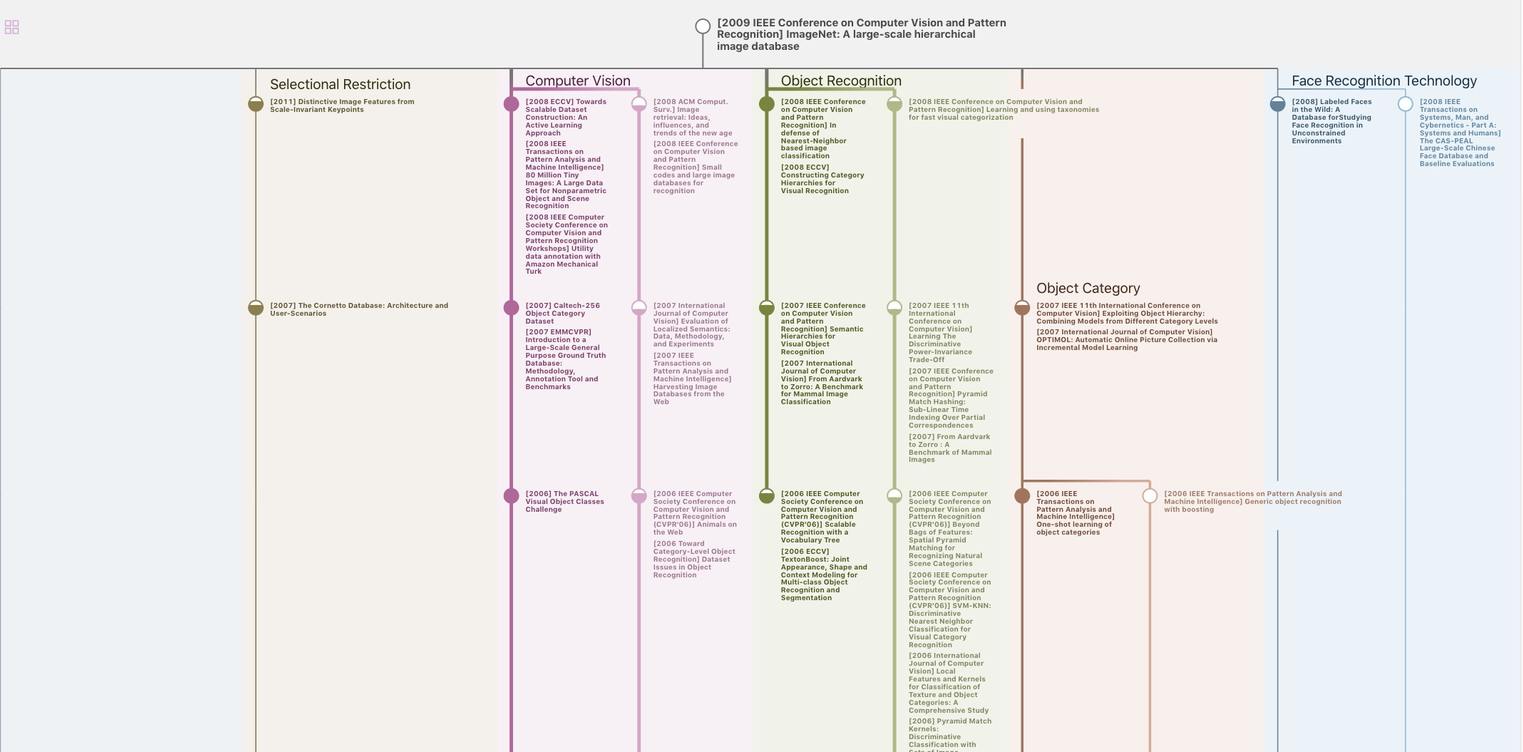
生成溯源树,研究论文发展脉络
Chat Paper
正在生成论文摘要