Machine learning and copula-based analysis of past changes in global droughts and socioeconomic exposures
JOURNAL OF HYDROLOGY(2024)
摘要
The intensification of the hydrological cycle has altered the spatiotemporal redistribution of terrestrial water storage (TWS). The long-term evolutionary mechanism controlling global TWS droughts and their bivariate risks (i.e., drought and severity) remain uncertain. Using machine learning-based TWS reconstructions, we explored the drivers, changes, and impacts of TWS droughts during 1940-2022 at the global scale. We developed a machine learning framework to detect the dominant climate/vegetation factors governing TWS. During 1940-1970, precipitation and vapor pressure deficit were the primary factors influencing TWS; however, the leaf area index was the dominant factor during 1992-2022. We evaluated past changes in drought frequency, duration, severity, and intensity, and found a substantial intensification tendency in most land areas. Subsequently, we evaluated the bivariate risks by combing a copula-based modeling approach and the most likely realization method, revealing a fivefold intensification over most regions. Changes in the marginal distributions of duration and severity accounted for 40-60% of the overall changes in bivariate drought risk, while the contribution from their dependence varied globally. Approximately 80-90% of the global population and gross domestic product were exposed to increasing bivariate drought risk, indicating the need to improve the adaptivity of society and ecosystems to climate change.
更多查看译文
关键词
Droughts,Terrestrial water storage,Machine learning,Socioeconomic exposure,Climate change
AI 理解论文
溯源树
样例
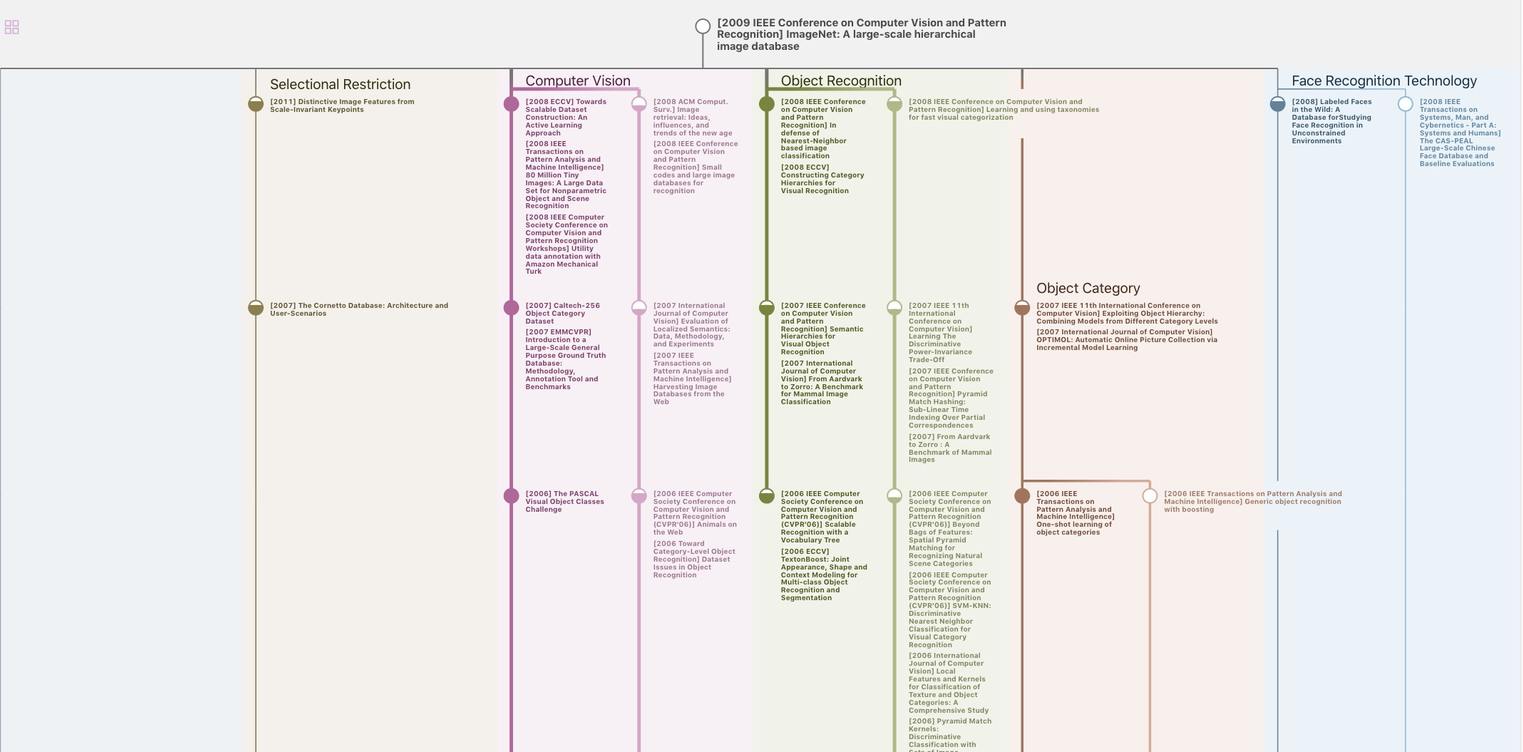
生成溯源树,研究论文发展脉络
Chat Paper
正在生成论文摘要