Class-specific dictionary-based cross-domain scene classification method with reliable target pseudo labels for remote sensing images
REMOTE SENSING APPLICATIONS-SOCIETY AND ENVIRONMENT(2024)
摘要
It is usually costly or even impossible to obtain required training labels of remote sensing images for traditional supervised classification method. Domain adaptation approaches can reduce the effort on obtaining training labels by transferring the knowledge in previously labeled data. How-ever, it may deliver an unsatisfactory performance resulted from diverse surrounding environ-ments, image sensors, geolocations and so on. Therefore, a class-specific dictionary-based cross-domain scene classification (CDCSC) method with reliable target pseudo labels is proposed for scene classification without labeled target data. First of all, a progressive target pseudo-labeled data selection strategy is proposed so as to augment the training dataset and increase the adaptive ability of the training dataset to the target domain. Then a class-specific constraint is proposed to learn class-specific transitional dictionaries that can draw data from the same class close and make data belonging to diverse types far away. Finally, the domain-invariant representations that concatenates the source, transitional, and target representations can make features discrimina-tive while decreasing the feature distribution difference. The CDCSC approach is experimented on three target images located in different cities, with two publicly available scene classification datasets serving as the training and validation datasets. The experiments confirm that the CDCSC can achieve an average accuracy over 85% without target labeled data.
更多查看译文
关键词
Scene classification Progressive target pseudo-labeled data tion Transitional dictionaries Class-specific dictionary Domain-invariant representations
AI 理解论文
溯源树
样例
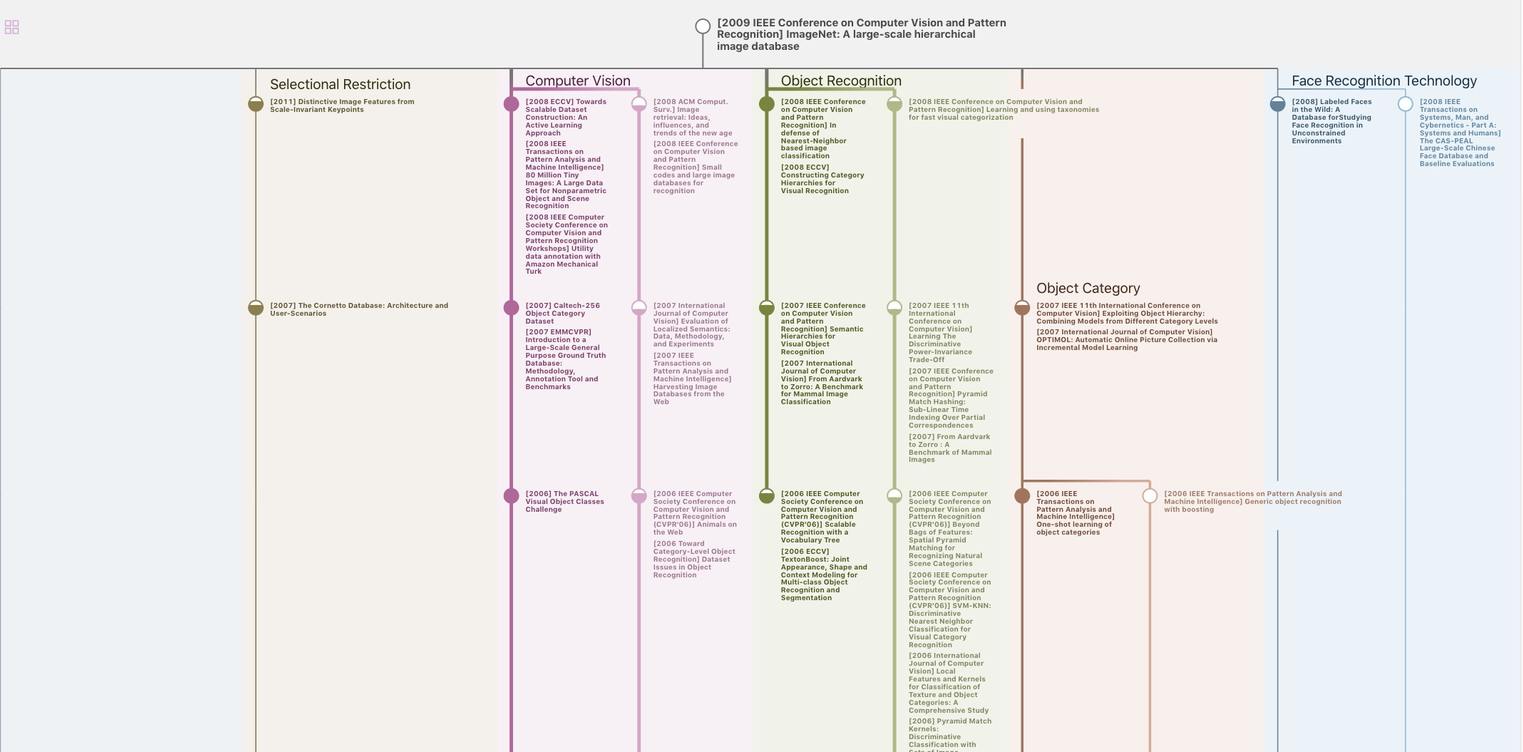
生成溯源树,研究论文发展脉络
Chat Paper
正在生成论文摘要