Dynamic Graph Contrastive Learning via Maximize Temporal Consistency
PATTERN RECOGNITION(2024)
摘要
Graph contrastive learning (GCL) is one of the most powerful self-supervised representation learning frame-works. Existing GCL methods have achieved impressive performance. However, it is still challenging to capture the evolution of nodes or edges, where the interaction of nodes or edges is stable in a short time but changeable at long time intervals. Therefore, it is crucial to capture the temporal consistency in dynamic graph. In this paper, we propose a novel Dynamic Graph Contrastive Learning framework, DyGCL, which learns node representation by maximizing the temporal consistency in a short time and discriminating the non-consistency in a long term. More specifically, DyGCL consists of two parts: GCL Trainer and Auxiliary Trainer. GCL Trainer focus on distinguishing temporal consistency and non-consistency. And the Auxiliary Trainer aims to improve the generalization ability with less labeled data as auxiliary supervision. Finally, we demonstrate the effectiveness and superiority of DyGCL by applying it to three datasets.
更多查看译文
关键词
Contrastive learning,Dynamic graph,Temporal information
AI 理解论文
溯源树
样例
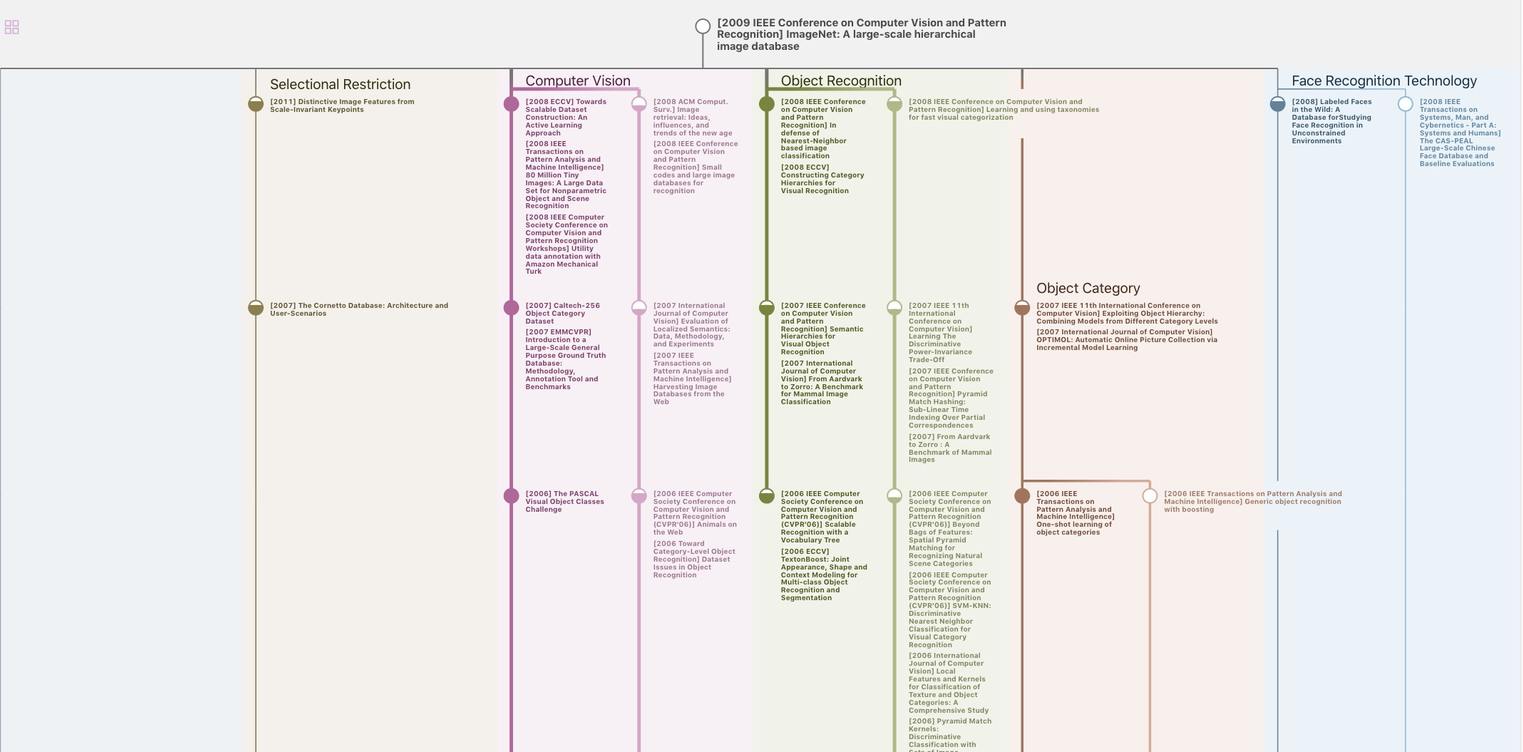
生成溯源树,研究论文发展脉络
Chat Paper
正在生成论文摘要