Robust peer-to-peer learning via secure multi-party computation
Journal of Information and Intelligence(2023)
摘要
To solve the data island problem, federated learning (FL) provides a solution paradigm where each client sends the model parameters but not the data to a server for model aggregation. Peer-to-peer (P2P) federated learning further improves the robustness of the system, in which there is no server and each client communicates directly with the other. For secure aggregation, secure multi-party computing (SMPC) protocols have been utilized in peer-to-peer manner. However, the ideal SMPC protocols could fail when some clients drop out. In this paper, we propose a robust peer-to-peer learning (RP2PL) algorithm via SMPC to resist clients dropping out. We improve the segment-based SMPC protocol by adding a check and designing the generation method of random segments. In RP2PL, each client aggregates their models by the improved robust secure multi-part computation protocol when finishes the local training. Experimental results demonstrate that the RP2PL paradigm can mitigate clients dropping out with no significant degradation in performance.
更多查看译文
关键词
Federated learning,Swarm learning,Secure multi-party computation,Peer-to-peer learning
AI 理解论文
溯源树
样例
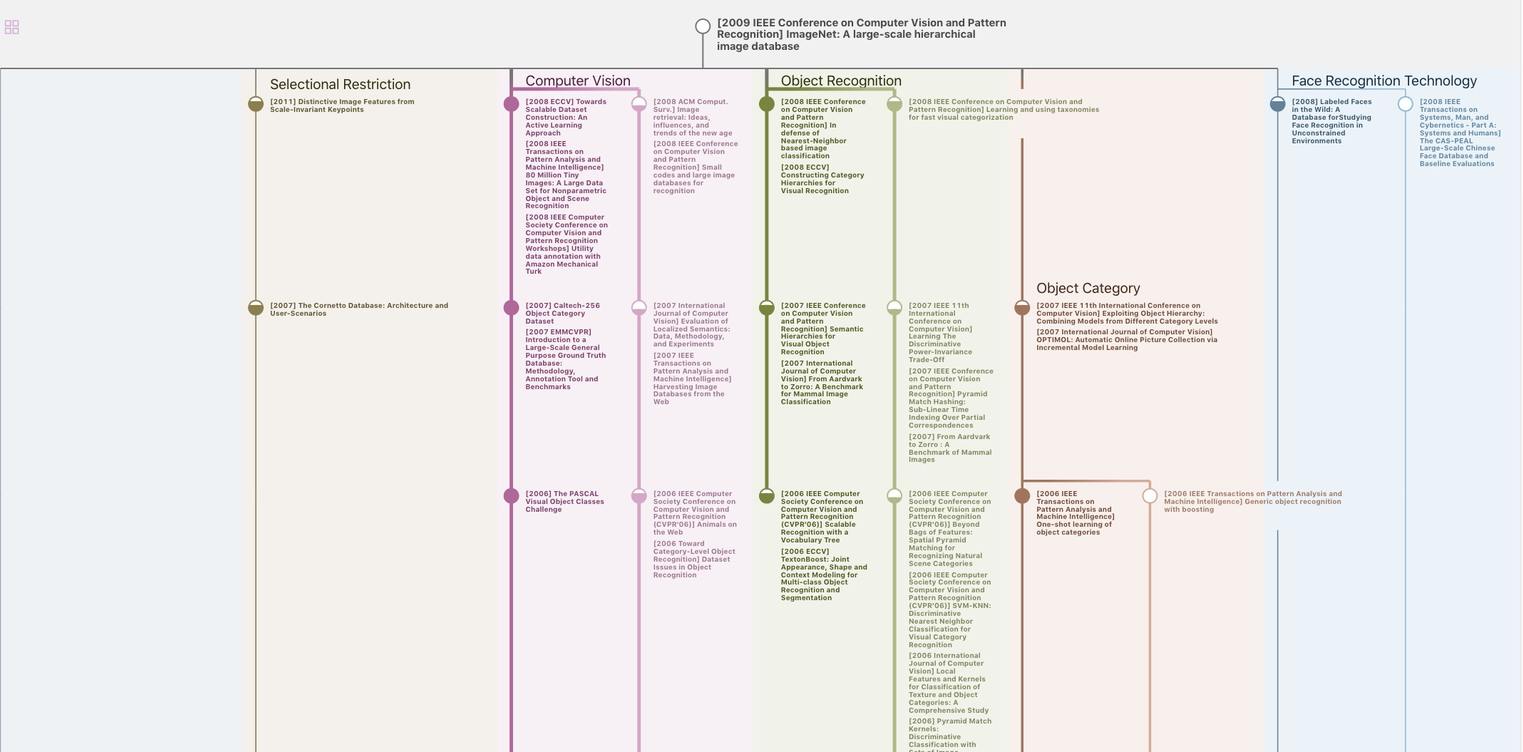
生成溯源树,研究论文发展脉络
Chat Paper
正在生成论文摘要