Mixformer: An improved self-attention architecture applied to multivariate chaotic time series
EXPERT SYSTEMS WITH APPLICATIONS(2024)
Abstract
Multivariate chaotic time series prediction has been a challenging problem, especially the coupling relationship between multiple variables need to be carefully considered. We propose a self-attention architecture with an information interaction module, called mixformer, applied to the multivariate chaotic time series prediction task. First, Based on rethinking the multivariate data structure, we compensate the deficiency of phase space reconstruction with a group of cross-convolution operator that can automatically update parameters, and propose an explicitly designed feature reconstruction module. Then, to address the problem of interactive fusion of series information and channel information, we propose an information interaction module that enables the feature communication by expanding and contracting dimensions. By breaking the communication barrier between series information and channel information, the feature representation capability is enhanced. Finally, we construct mixformer that combines locally sparse features and global context features. And notably, mixformer integrates the information interaction module and the feature reconstruction module to form a continuous solution. By comparing the results with existing models using multiple simulated data (Lorenz, Chen, and Rossler systems) and real-world application (power consumption), it is verified that our proposed model achieves surprising performance in practice.
MoreTranslated text
Key words
Chaotic time series,Multivariate prediction,Information interaction,Global context modeling,Self-attention architecture
AI Read Science
Must-Reading Tree
Example
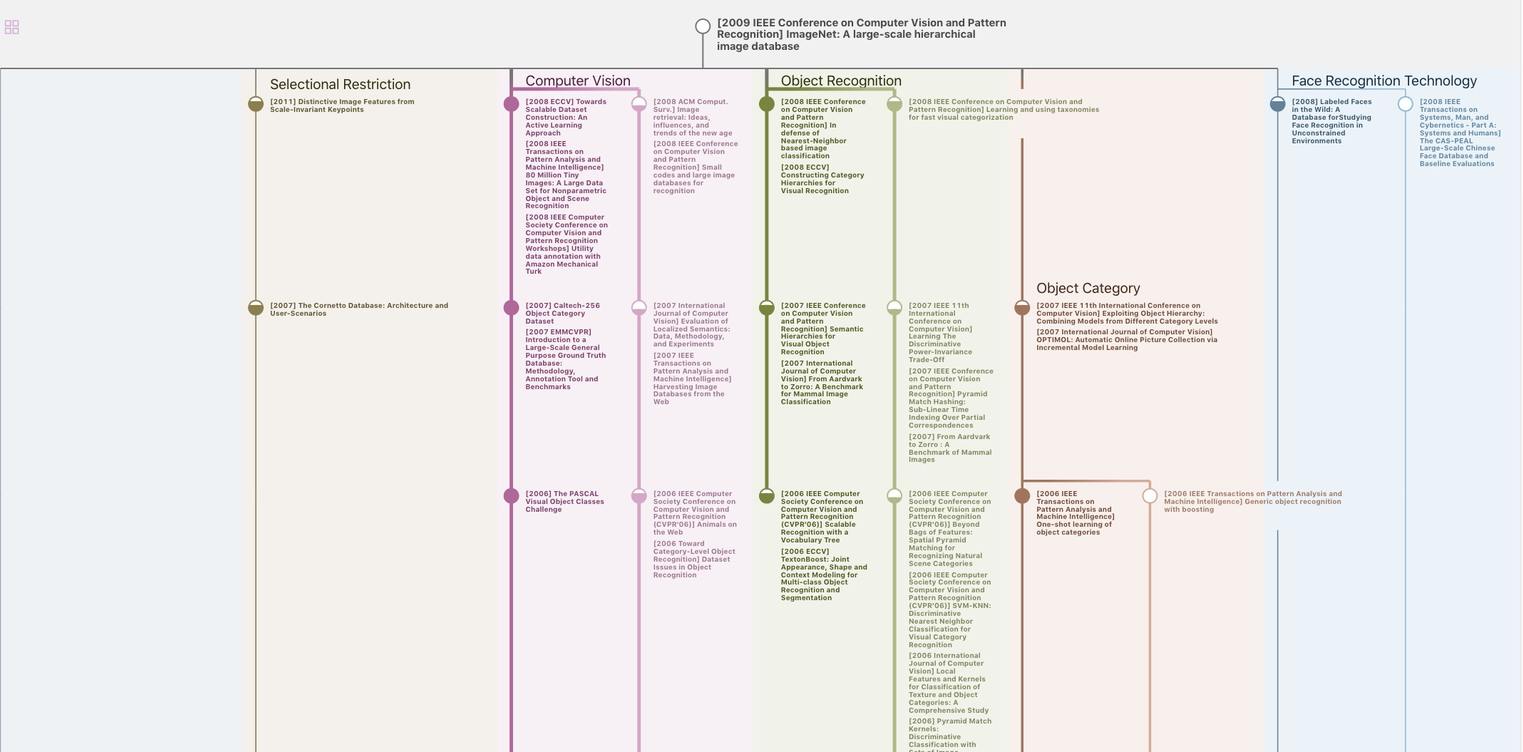
Generate MRT to find the research sequence of this paper
Chat Paper
Summary is being generated by the instructions you defined