Mode standardization: A practical countermeasure against mode collapse of GAN-based signal synthesis
APPLIED SOFT COMPUTING(2024)
摘要
Intelligent fault diagnosis, particularly via data -driven deep learning, is vital for industrial health management. However, it often faces challenges due to unbalanced datasets, with either insufficient fault data or redundant data representing the same condition. For signal -based diagnosis, generative adversarial networks (GANs) provide a countermeasure by generating new signals for data augmentation but can suffer from mode collapse, causing monotonous new signals and further imbalance. Inspired by the conditional mechanism, we propose mode standardization to mitigate mode collapse in GAN-based signal synthesis. This method utilizes part of the original signals as reference input to the generator. As a result, although mode collapse still occurs, and the new signals may still be monotonous, this monotony is confined to their references. In other words, as long as the references are diverse, the generated signals will maintain an acceptable diversity, similar to the original. The negative effects of mode collapse are diminished, decreasing harm to the generation process. Comparative experiments demonstrate mode standardization's effectiveness in diversity and quality on various datasets, affirming it as a practical countermeasure against mode collapse and a suitable strategy for industrial practice.
更多查看译文
关键词
Data augmentation,Deep learning,Generative adversarial networks (GANs),Mode collapse,Signal synthesis
AI 理解论文
溯源树
样例
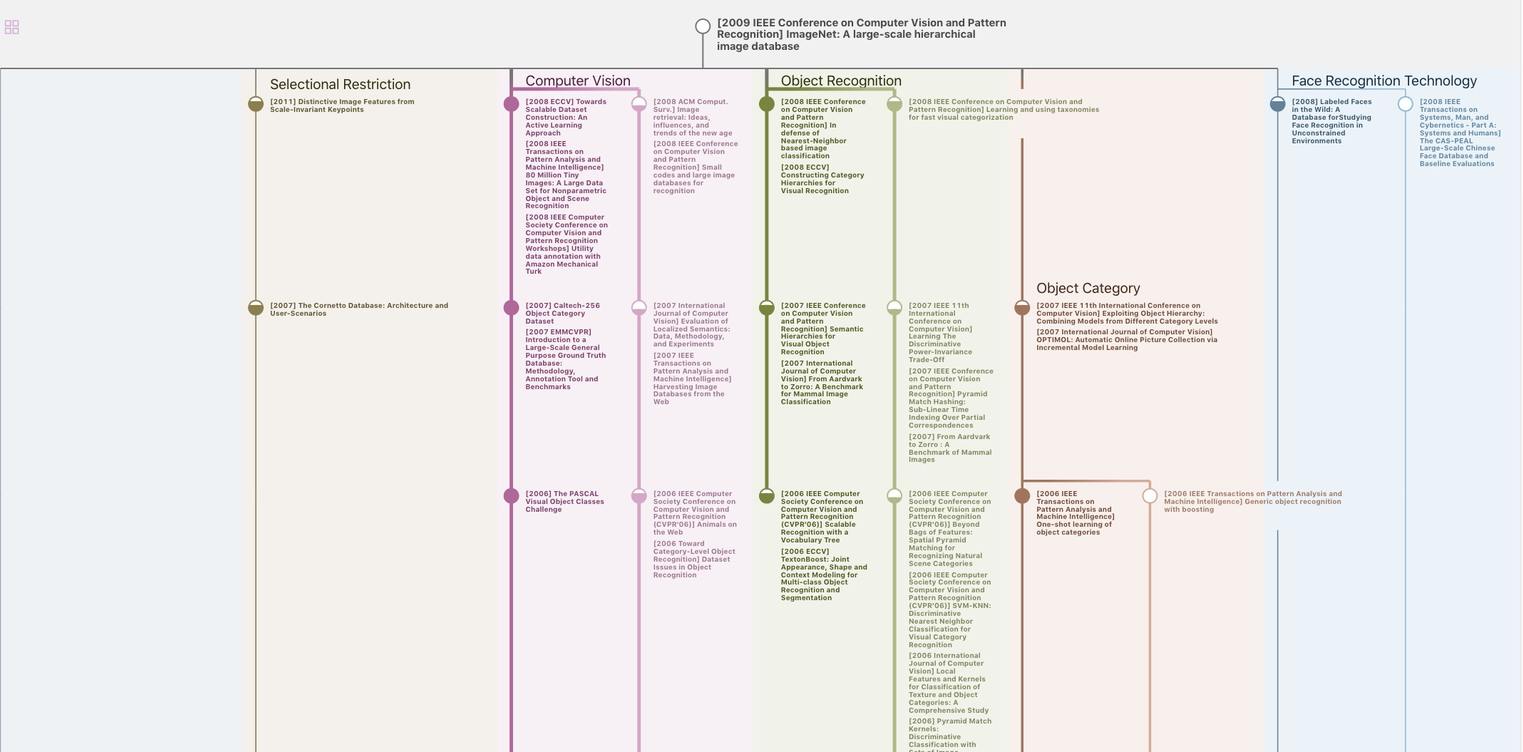
生成溯源树,研究论文发展脉络
Chat Paper
正在生成论文摘要