SynDroid: An adaptive enhanced Android malware classification method based on CTGAN-SVM
COMPUTERS & SECURITY(2024)
摘要
Android mobile phones have the highest market share nowadays, bringing a boom of Android application programming as well as malicious software (malware) issues. Traditional machine learning and deep learning methods are widely used in Android malware detection and classification, both require plenty of application samples to train classifiers. However, the collected samples are always imbalanced, because the distribution of malware categories differs hugely in the real environment. For Android malware samples with high dimension features, and Class Imbalance Ratio coming to more than 100:1, traditional methods become weak. To fill the gap, we propose an Android malware classification model named SynDroid. The core step of SynDroid uses CTGAN-SVM to generate qualified high-dimension samples, and adaptively discards bad results. Besides, we propose KS-CIR test to help the model to determine which classes of data needed to be enhanced most. This new proposed test can measure the data in respect of both samples' quality and quantity. Lastly, Random Forest is taken as a classifier to finish the classification task. The performance of SynDroid is evaluated on CCCS-CIC-AndMal2020 on the accuracy, precision, recall and F1-score. Both longitudinal and horizontal comparison experiments have been done with traditional oversampling methods, cost-sensitive learning, and other complicated methods. The result shows that the proposed method gets 12% more accuracy than the method on the same dataset and alleviates imbalanced data problems.
更多查看译文
关键词
Android malware classification,GAN,Imbalanced dataset,Data augmentation
AI 理解论文
溯源树
样例
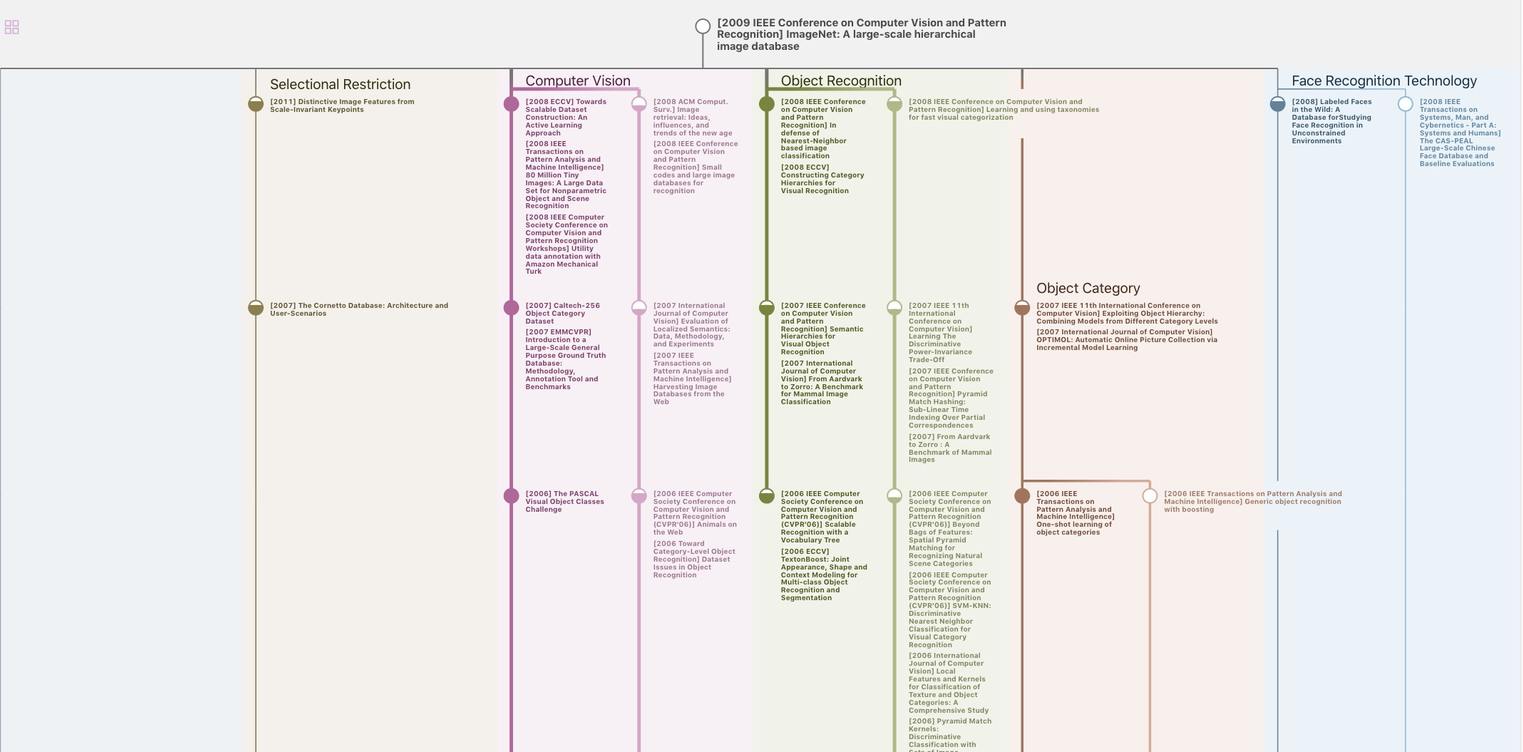
生成溯源树,研究论文发展脉络
Chat Paper
正在生成论文摘要