Learning multiple attention transformer super-resolution method for grape disease recognition
EXPERT SYSTEMS WITH APPLICATIONS(2024)
摘要
Grape leaf disease is a primary factor affecting grape yield and impeding the growth of the industrial economy. Currently, high-quality datasets are required for deep recognition models to effectively identify grape leaf diseases. In reality, the acquired image inevitably has the appearance of being low quality and unclear due to the influence of equipment and environment. The super-resolution method is an effective way to solve the problem. However, due to the complex model structure and learning strategies, existing super-resolution methods are computationally expensive and neglect the balance between global and local feature extraction. In this paper, a novel multiple attention transformer super-resolution (MATSR) method is proposed to solve these problems. Specifically, the method uses convolution to extract low-level features, which are then fed into the multiple attention shuffle transformer (MAST) module. The module consists of the shuffle transformer for global feature extraction, the constructed channel spatial attention block (CSAB) for local feature extraction, and the built reduisal feature block (RFB) for aggregating these two features. Meanwhile, considering the importance of the balance of global and local features for grape leaf disease recognition, a dynamic learning strategy is designed to balance these two features. Further, the abstract feature extraction layer combined the learned features with the low-level features. Finally, these abstracted features are fed into the pixels shuffle layer for super-resolution upsampling. Numerous comparative experiments are carried out on the collected high-resolution grape leaf disease images as well as the apple leaf pathological images. The results demonstrate the proposed method achieved the best PSNR of 38.152 dB and SSIM of 0.96 at scale x2, and 35.896 dB and SSIM of 0.934 at scale x3, respectively. Furthermore, four recognition models are experimented on the super-resolution grape disease dataset, among which ShuffleNet and Inception achieve the best accuracy of 98.28 % and 98.53 %, respectively. Therefore, this study can effectively aid grape growers in accurately identifying diseases and taking proactive measures.
更多查看译文
关键词
Grape disease identification,Image super-resolution,Lightweight,CNN,Transformer networks
AI 理解论文
溯源树
样例
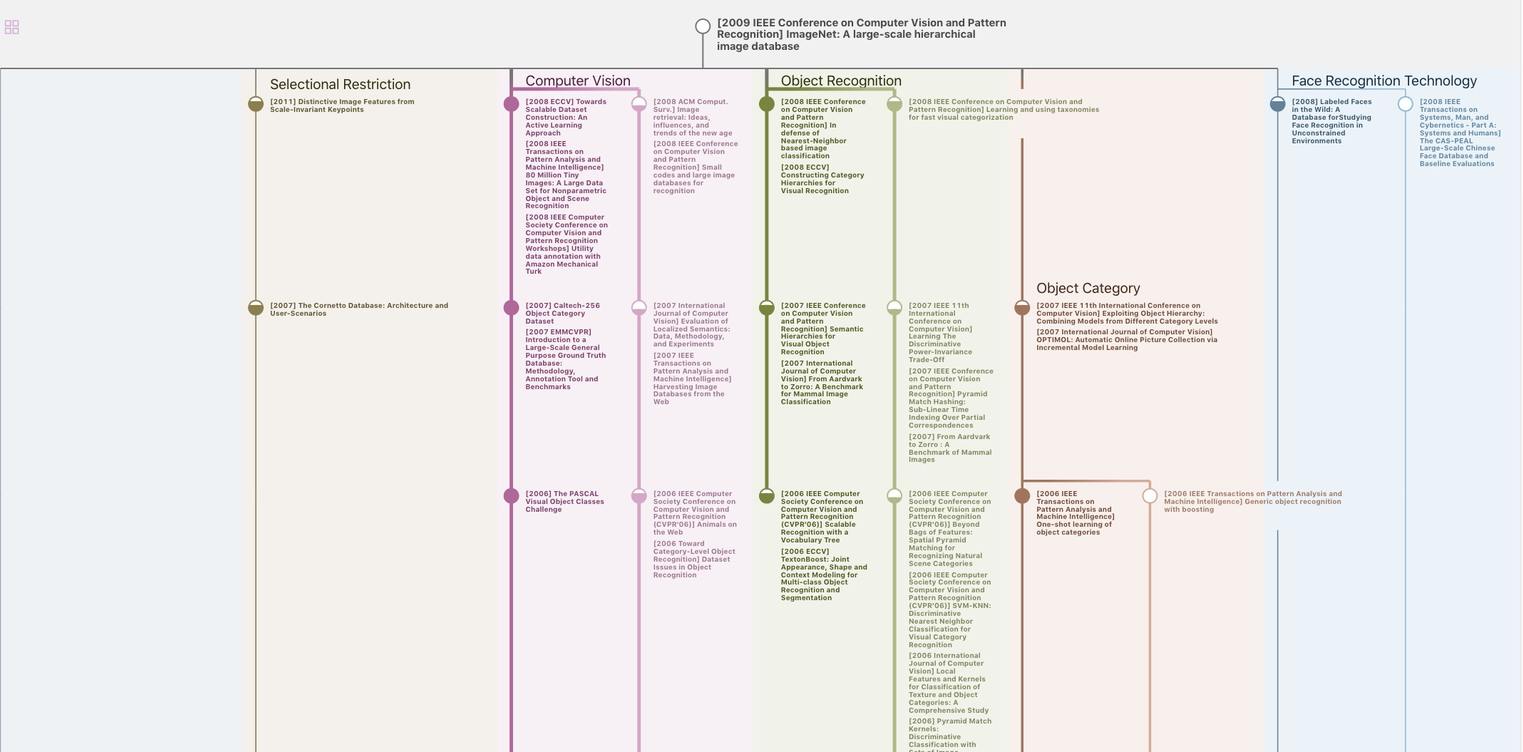
生成溯源树,研究论文发展脉络
Chat Paper
正在生成论文摘要