HN3S: A Federated AutoEncoder framework for Collaborative Filtering via Hybrid Negative Sampling and Secret Sharing
INFORMATION PROCESSING & MANAGEMENT(2024)
摘要
Federated recommender systems can serve users with suitable item recommendations while preserving their privacy, but most current works cannot serve non-participant users. We consider that Federated AutoEncoder for Collaborative Filtering (FAE-CF) is expected to solve this problem. However, FAE-CF encounters privacy protection concerns and high communication overhead issues. In this paper, we investigate FAE-CF's privacy protection capabilities and communication volume, unveiling its potential privacy security issues and inferior practicality. By analyzing the FAE-CF gradient, we propose an effective attack that could recover most user data, with all recovery metrics above 95.53% in our simulation experiments. To address these challenges, we propose Hybrid Negative Sampling and Secret Sharing (HN3S), a privacy preserving method designed for FAE-CF. HN3S uses hybrid negative sampling to reduce communication overhead and secret sharing to protect user data. Experiments conducted on various public datasets with multiple AE-CFs have shown that HN3S can effectively protect the privacy of FAE-CF, reducing the communication overhead by up to 60.44%, while maintaining the performance reduction within 10.78%. Our research reveals significant privacy risks and poor utility inherent in current FAE-CFs, and provides a feasible solution to protect user privacy and balance communication overhead with algorithm performance.
更多查看译文
关键词
Federated recommendation,Collaborative filtering,Variational autoencoder,Negative sampling,Secret sharing
AI 理解论文
溯源树
样例
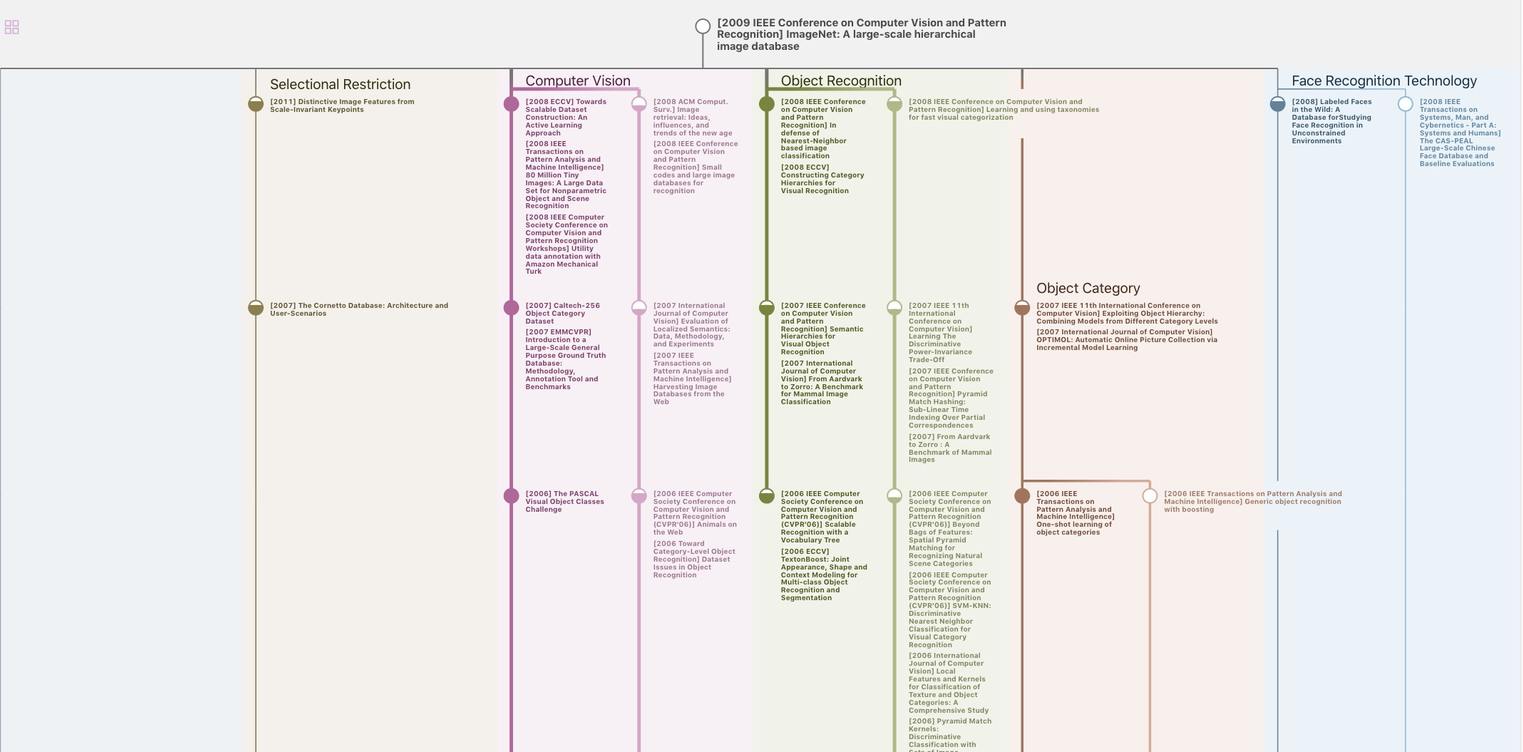
生成溯源树,研究论文发展脉络
Chat Paper
正在生成论文摘要