FACILE: A capsule network with fewer capsules and richer hierarchical information for malware image classification
COMPUTERS & SECURITY(2024)
摘要
The struggle between security researchers and malware perpetuates an endless arms race. Recent studies indicate that converting malware into grayscale images and using convolutional neural network or capsule network to classify and identify them is a promising approach. Nonetheless, training convolutional neural networks demands a significant amount of data and parameters, posing a challenge to obtaining enough training samples for malware detection tasks, particularly for new malware families. Additionally, a high number of parameters translates to low classification efficiency. Capsule networks offer a promising approach to achieve higher-level feature representations through dynamic routing between capsules, but research on capsule networks is still nascent, and there are two major challenges for malware classification tasks: the huge number of parameters, which makes model training difficult, and capsules shedding, which results in information loss during routing. To address these issues, we propose a capsule network called FACILE that uses fewer capsules and richer hierarchical information. In the initial feature extraction stage, we perform multi-level feature extraction and fusion using dynamic convolution. In the routing phase, we introduce balance coefficients to enhance the model's representational power and stabilize the training process. We conducted experiments on the VIRUS-MNIST, MalImg, and BIG2015 datasets and found that FACILE requires only 8.1% of the capsules and 1.8%-3.3% of the parameters compared to the original CapsNet, while reducing the error rate to 8.087%, 1.149%, and 2.797%, respectively. Furthermore, FACILE demonstrates competitive performance with a small number of parameters as the training samples decrease compared to convolutional neural network-based models such as ResNet, EfficientNet, and VGG.
更多查看译文
关键词
Malware classification,Capsule network,Feature extraction,Dynamic convolution,Dynamic routing
AI 理解论文
溯源树
样例
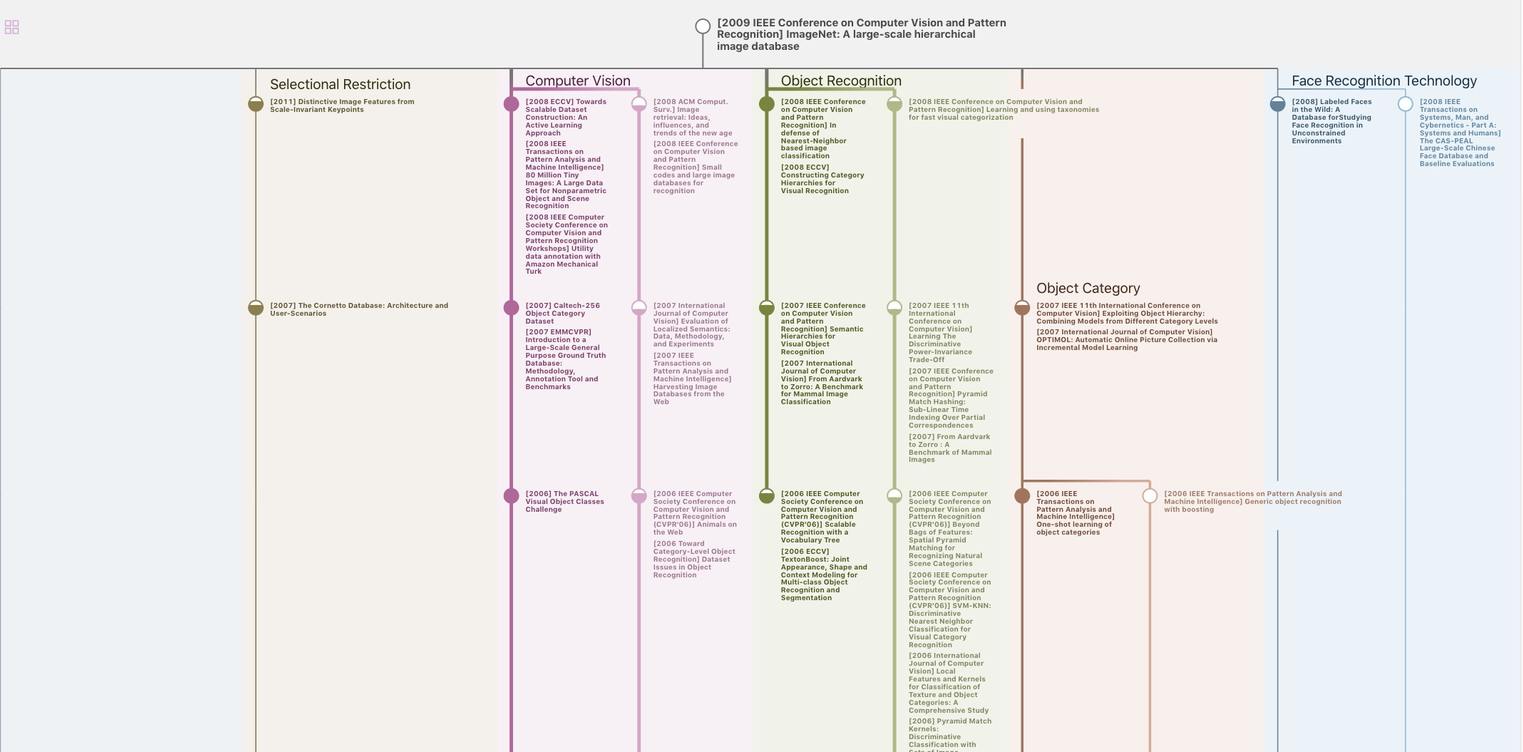
生成溯源树,研究论文发展脉络
Chat Paper
正在生成论文摘要