Prediction of dissolved organic nitrogen via spectroscopic fingerprint in the shallow riverbed sediments of effluent-dominated rivers: A case study in Xi'an, northwest China
JOURNAL OF HYDROLOGY(2024)
摘要
An evaluation on the impacts of effluent discharge to receiving rivers is in demand. Discharged dissolved organic nitrogen (DON) with high eutrophication potentials tends to accumulate in the shallow riverbed sediments especially for effluent-dominated rivers. Since DON characterization requires for advanced analytical tools, there is thereby a need but it is still a challenge to evaluate their distribution and transformation in the riverbed sediments. Considering the fact that DON is an important part of dissolved organic matter (DOM), which has plentiful spectral information, the authors hypothesize if it is feasible to predict DON variations in riverbed sediments via the DOM properties in surface water or sediment porewater? Here, the spatiotemporal spectral characteristics of DOM in river water and sediment porewater of two effluent-dominated rivers were investigated. Compared to surface water, more abundant relationships among fluorephores and spectra-derived parameters in porewater were observed. The relatively high DON contents in both surface water (0.16-0.47 mg-N/ L) and sediment porewater (0.35-5.02 mg-N/L) compared to those reported in other natural waters highlighted the importance in predicting DON in effluent-dominated rivers. The DON in the oxic zone from winter samples carried more humus-like signals, while the fractions in summer samples recognized protein-like and humus-like signals. Two machine learning methods, i.e., random forest (RF) and extreme gradient boosting (XGBoost) were employed to provide evidence-based information on how DON contents and properties differed at variable depths. RF (R2: 0.920, 0.475) outperformed XGBoost (R2: 0.488, 0.316) in predicting DON in winter-oxic zone and winter-suboxic zone. While XGboot (R2: 0.515, 0.787) is superior than RF (R2: 0.317, 0.567) in summer-oxic zone and summer-suboxic zone. Fluorophore of C1 served as the most important variable for predicting DON in winter samples in both models. SUVA260 and SUVA280 were verified most impressive predictor for samples from suboxic zone in summer in both models. HIX represented the most important variable predicting DON in summer samples in XGBoost model. These findings are critical for understanding the transformation processes of DON in the shallow riverbed sediments in receiving rivers, and could provide important implications to improve wastewater discharge management and river health.
更多查看译文
关键词
Effluent-dominated rivers,Shallow riverbed sediments,Spectral fingerprint,Dissolved organic nitrogen,Machine learning
AI 理解论文
溯源树
样例
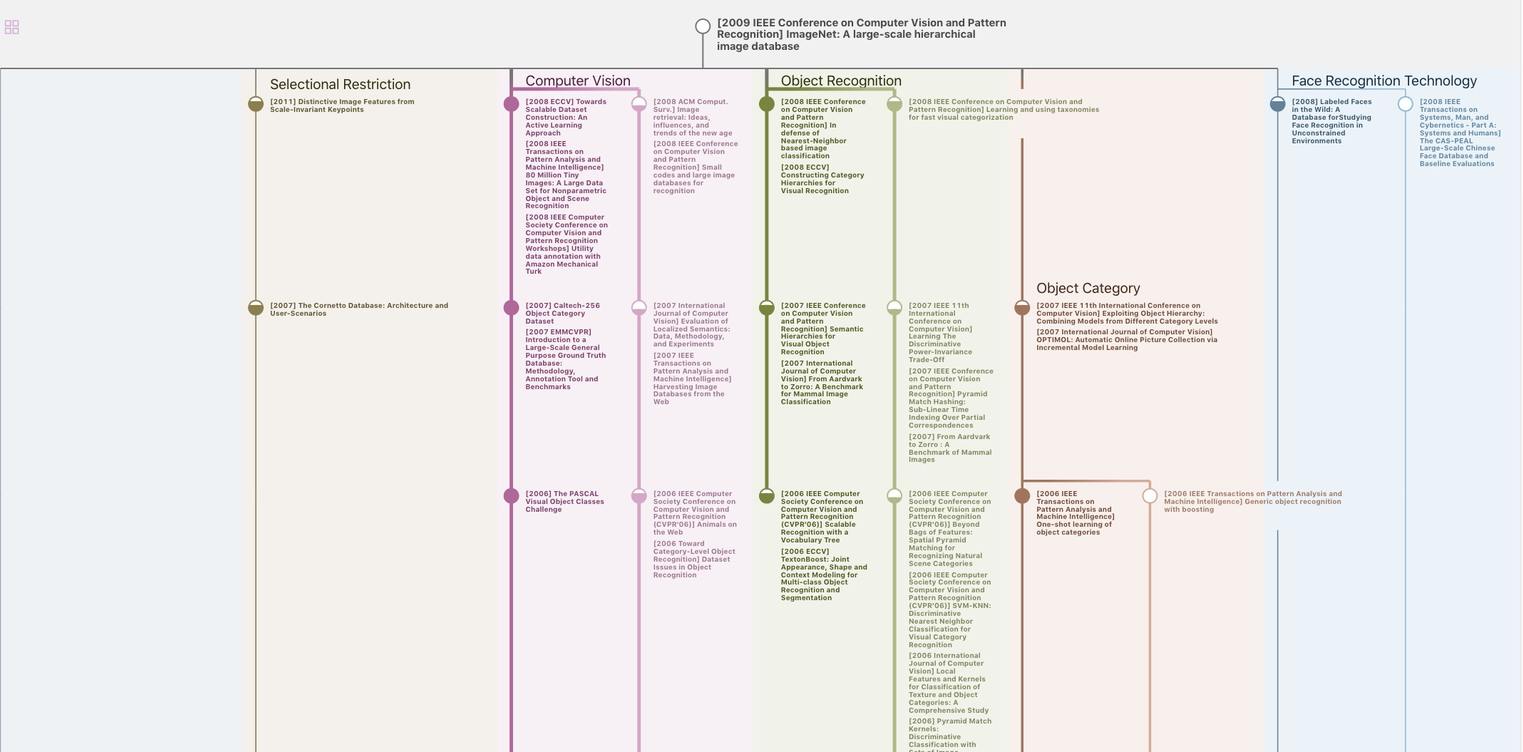
生成溯源树,研究论文发展脉络
Chat Paper
正在生成论文摘要