A data-driven operating improvement method for the thermal power unit with frequent load changes
APPLIED ENERGY(2024)
摘要
In the near and medium term, thermal power generation will still play an important peak-shaving role in providing grid connection to volatile renewable energy. Thermal power units need to adjust from the current load to the given load within a given time period, which provides space for operational improvement. In this paper, we propose an operating improvement method for the thermal power unit based on real-time monitoring data by observation dividing, feature construction and selection, clustering, and machine learning. The unit operation data is categorized into three feature subsets based on domain knowledge, which is used to distinguish different functions of historical data. Subsequently, the optimal feature subset and observations are selected for building regression models, including linear regression (LR), ensemble tree regression (ETR), neural network regression (NNR), and support vector regression (SVR). R-squared (R-2), root mean square error (RMSE), and mean absolute error (MAE) are adopted to test the performance of the proposed regression model on the realtime monitoring data of a thermal unit, which has 80,000 observations of 36 different variables. Compared with benchmark methods, the proposed method has lower regression error in the numerical experiment. Thus, we can thereby improve the efficiency of operational management based on the built learning model. Furthermore, in response to peak shaving requirements, the proposed method in this article considers operational optimization over time periods containing multiple time points compared to the traditional operational optimization perspective. With the continuous arrival of monitoring data, the above method can be updated in a timely manner based on the new database to address model adjustments caused by unit aging and other factors.
更多查看译文
关键词
Clustering,Data mining,Feature construction,Feature selection,Operating improvement,Thermal power unit
AI 理解论文
溯源树
样例
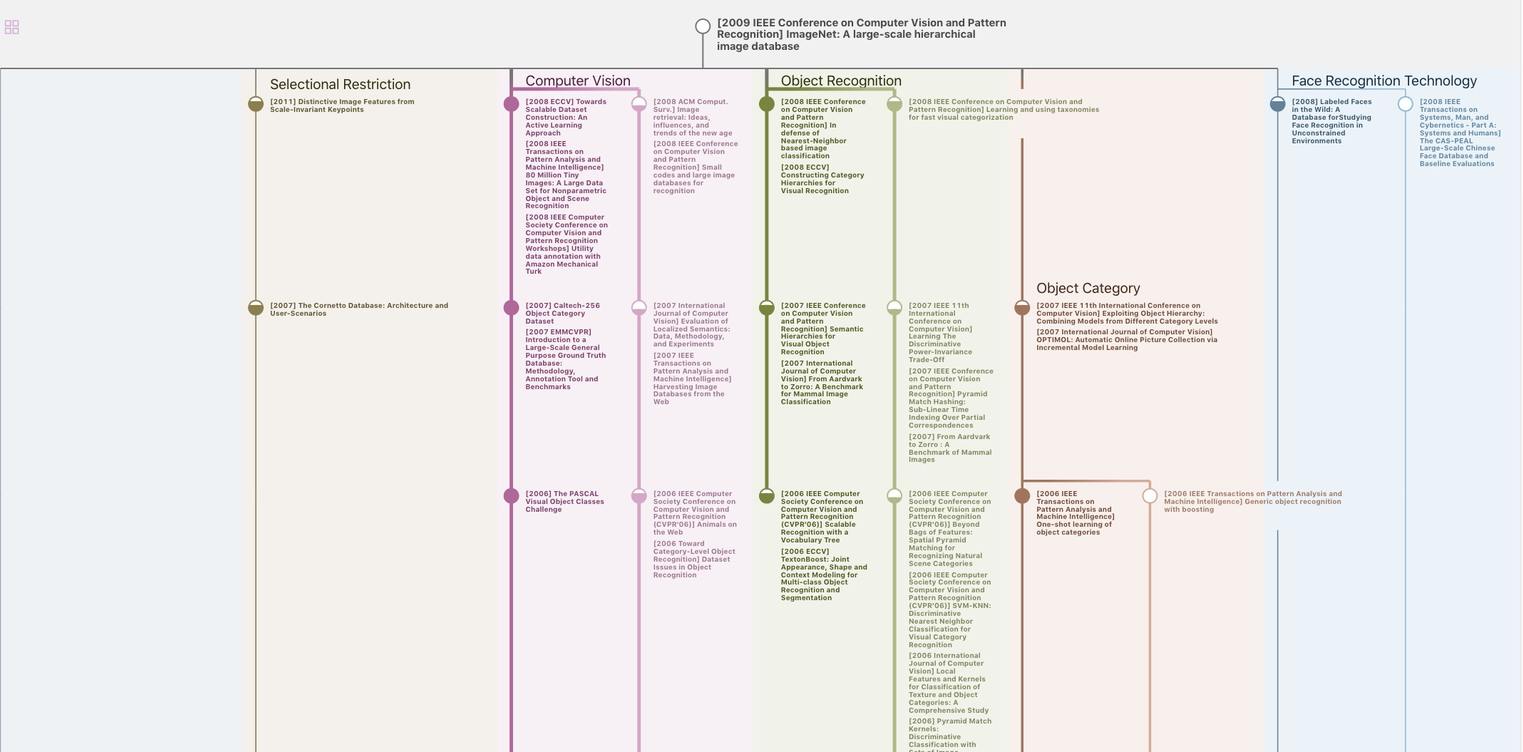
生成溯源树,研究论文发展脉络
Chat Paper
正在生成论文摘要