Reshaping Model Predictive Control Penalties for an Automated Insulin Delivery System in Type 1 Diabetes
IFAC PAPERSONLINE(2023)
摘要
Model Predictive Control ( MPC) is one of the most popular control algorithms for Automated Insulin Delivery (AID) in Type 1 Diabetes ( T1D). So far, several MPC formulations of varying complexity have been proposed, but there is still no general consensus on how to define their cost weights. In this work, we evaluate the performance impact of reshaping the error weight (Q(z)) of RocketAP, a previously developed MPC-based AID system that has been successfully tested in in-silico and in-vivo trials. We analyzed two strategies: (i) a constant Q(z) and (ii) a Q(z) that is a function of the estimated Insulin on Board (IOB), Q(z)(IOB). In both cases, function parameters are determined via grid search with a cost function computed from glycemic outcome metrics. Performance is assessed on the 100-adult cohort of the FDA-accepted UVA/Padova metabolic simulator by clamping the time spent below 70 mg/dL (TBR), and comparing the achievable time spent between 70-180 mg/dL (TIR) and time spent above 250 mg/dL (TAR) in two different 48-hour scenarios. Results indicate that detuning the controller as a function of IOB, Q(z)(IOB), leads to significantly better (p0.001, t-test) glycemic control ( TIR = 78 +/- 10.6%) compared to using a constant error weight in the MPC formulation ( TIR = 70 +/- 9.3%). Copyright (c) 2023 The Authors.
更多查看译文
关键词
Optimal control,Model predictive control,Artificial Pancreas system,Type 1,diabetes,time-varying costs.
AI 理解论文
溯源树
样例
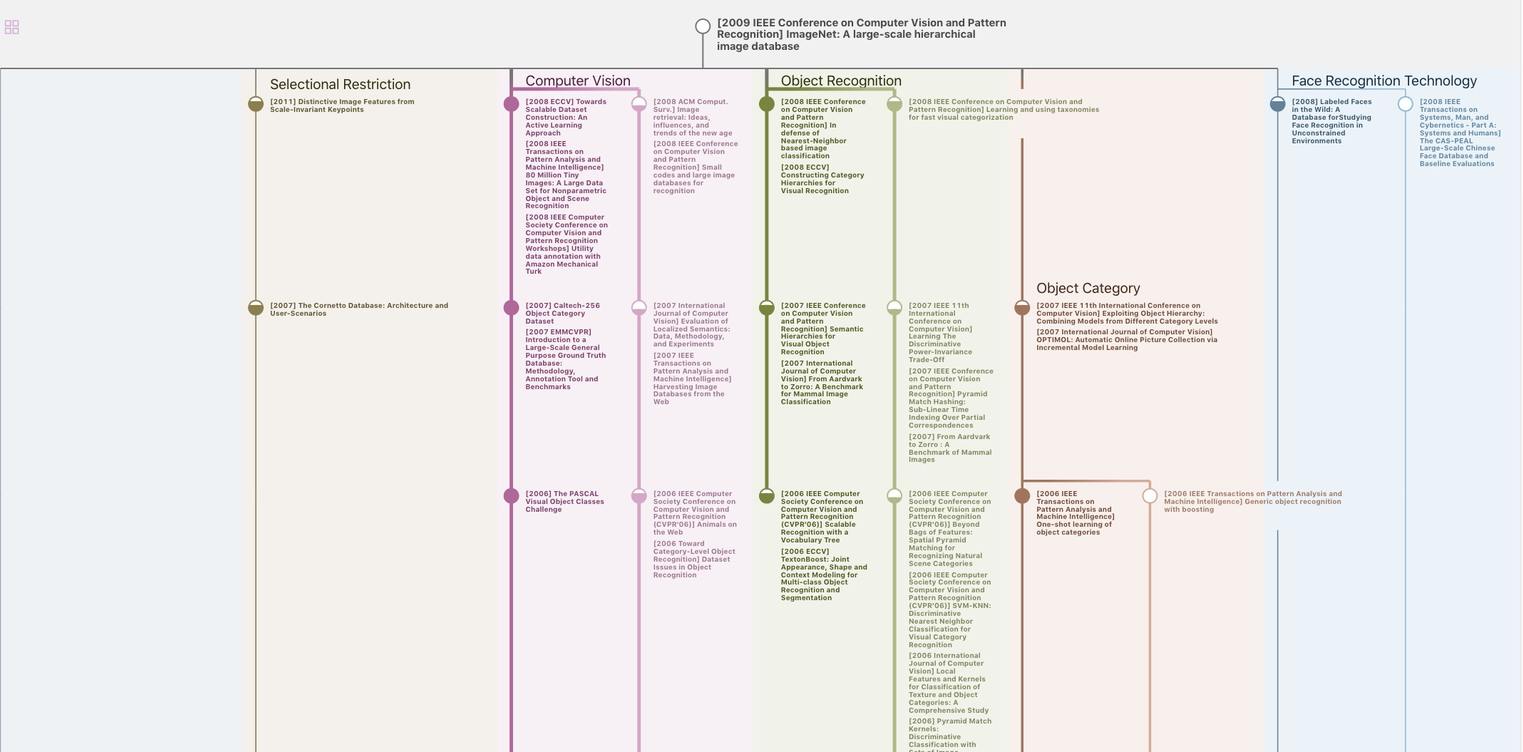
生成溯源树,研究论文发展脉络
Chat Paper
正在生成论文摘要