Sampling methods based on expected traffic-volume information for long-term rotation-based bridge SHM in resource-constrained environments
MECHANICAL SYSTEMS AND SIGNAL PROCESSING(2024)
Abstract
Effective bridge structural health monitoring systems offer a route to assist in the safe and economic operation of bridges, but have seen limited adoption onto the short and medium-span bridges which represent the majority of our bridge stock. This lack of adoption is in part due to the practical challenges of deploying bridge SHM systems in resource-constrained environments, especially where long-term monitoring at transport network-scales is considered. This paper tackles the problem of implementing an effective sampling method to facilitate long-term, bridge rotation-based damage detection, particularly within the confines of a resource-constrained setting. We demonstrate that leveraging readily available expected traffic-volume information enables the creation of more effective bridge SHM sampling methods by capturing more vehicle crossings in the same time. Furthermore, we introduce an empirically tuned, temperature-capable bridge finite-element model for long-term simulations, incorporating a stochastic vehicle arrival model and a feature error model to allow for the presence of any residual noise/dynamics due to imperfect filtering or inaccuracies in the feature extraction stages. This allowed us to simulate 60 years worth of bridge rotation monitoring data with varying damage severities to evaluate the impact of environmental and operational variations on our proposed expected traffic volume based sampling methods. Our results show that sampling only the peak traffic hours was the most effective for the same reduced 'on' time, i.e. for an 8x reduction in 'on' time only a 1.5x increase in the minimum detectable damage was observed versus a 2x increase for naive random sampling.
MoreTranslated text
Key words
Bridge structural health monitoring,Rotation-based damage detection,Long-term monitoring,Sampling methods,Expected traffic volume based sampling
AI Read Science
Must-Reading Tree
Example
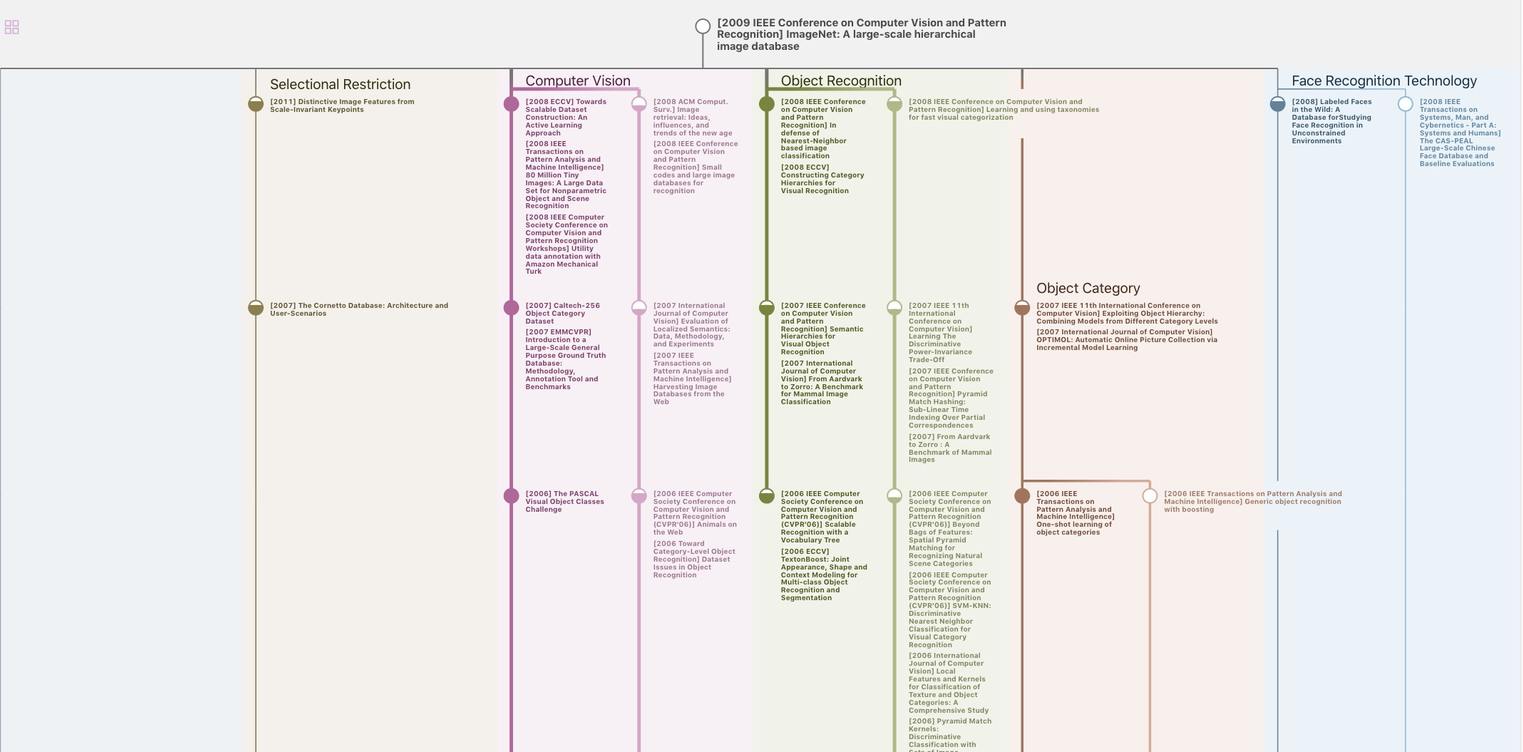
Generate MRT to find the research sequence of this paper
Chat Paper
Summary is being generated by the instructions you defined