Surrogate grid model of an atmospheric pollutant spread
IFAC PAPERSONLINE(2023)
摘要
This paper presents a method for developing computationally-efficient surrogate models for the spread of air pollution. Mitigating the pollution of Seveso-type accidents and designing evacuation scenarios require long-term prediction, which is obtained with numerical simulations of the spread of air pollution. Sophisticated simulation programs frequently possess high computational load and are not suitable for real-time computational studies and experiments. Data-driven surrogate models that are computationally fast are used for such investigations. We propose a grid of independent dynamical Gaussian-process models (GPGIM) to simulate the spread of atmospheric pollution. This is demonstrated using a realistic example of limited complexity based on a thermal power plant in S. o.stanj, Slovenia. The results show an acceptable behaviour match between the surrogate and original models, with a tenfold decrease in computational load. This confirms the feasibility of the proposed method and makes the resulting surrogate model suitable for further experiments. Copyright (c) 2023 The Authors.
更多查看译文
关键词
Machine learning for environmental applications,model reduction and dynamic,emulation,modelling and identification of environmental systems
AI 理解论文
溯源树
样例
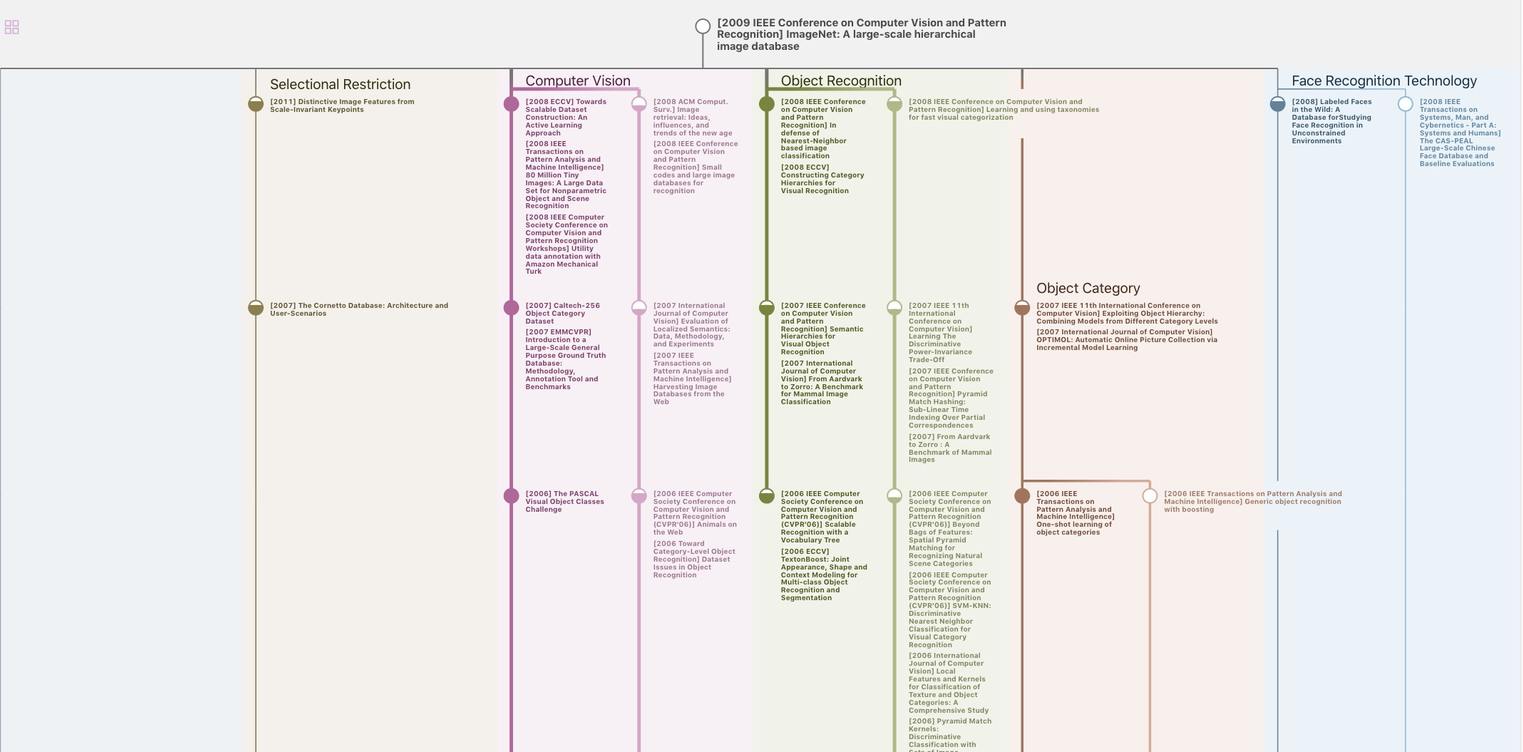
生成溯源树,研究论文发展脉络
Chat Paper
正在生成论文摘要