Entropy Rate Maximization of Markov Decision Processes for Surveillance Tasks
IFAC PAPERSONLINE(2023)
摘要
We consider the problem of synthesizing optimal policies for Markov decision processes (MDP) for both utility objective and security constraint. Specifically, our goal is to maximize the entropy rate of the MDP while achieving a surveillance task in the sense that a given region of interest is visited infinitely often with probability one (w.p.1). Such a policy is of our interest since it guarantees both the completion of tasks and maximizes the unpredictability of limit behavior of the system. Existing works either focus on the total entropy or do not consider the surveillance tasks which are not suitable for surveillance tasks with infinite horizon. We provide a complete solution to this problem. Specifically, we present an algorithm for synthesizing entropy rate maximizing policies for communicating MDPs. Then based on a new state classification method, we show the entropy rate maximization problem under surveillance task can be effectively solved in polynomial-time. We illustrate the proposed algorithm based on a case study of robot planning scenario. Copyright (c) 2023 The Authors.
更多查看译文
关键词
Markov Decision Processes,Entropy Rate,Surveillance Task,Security
AI 理解论文
溯源树
样例
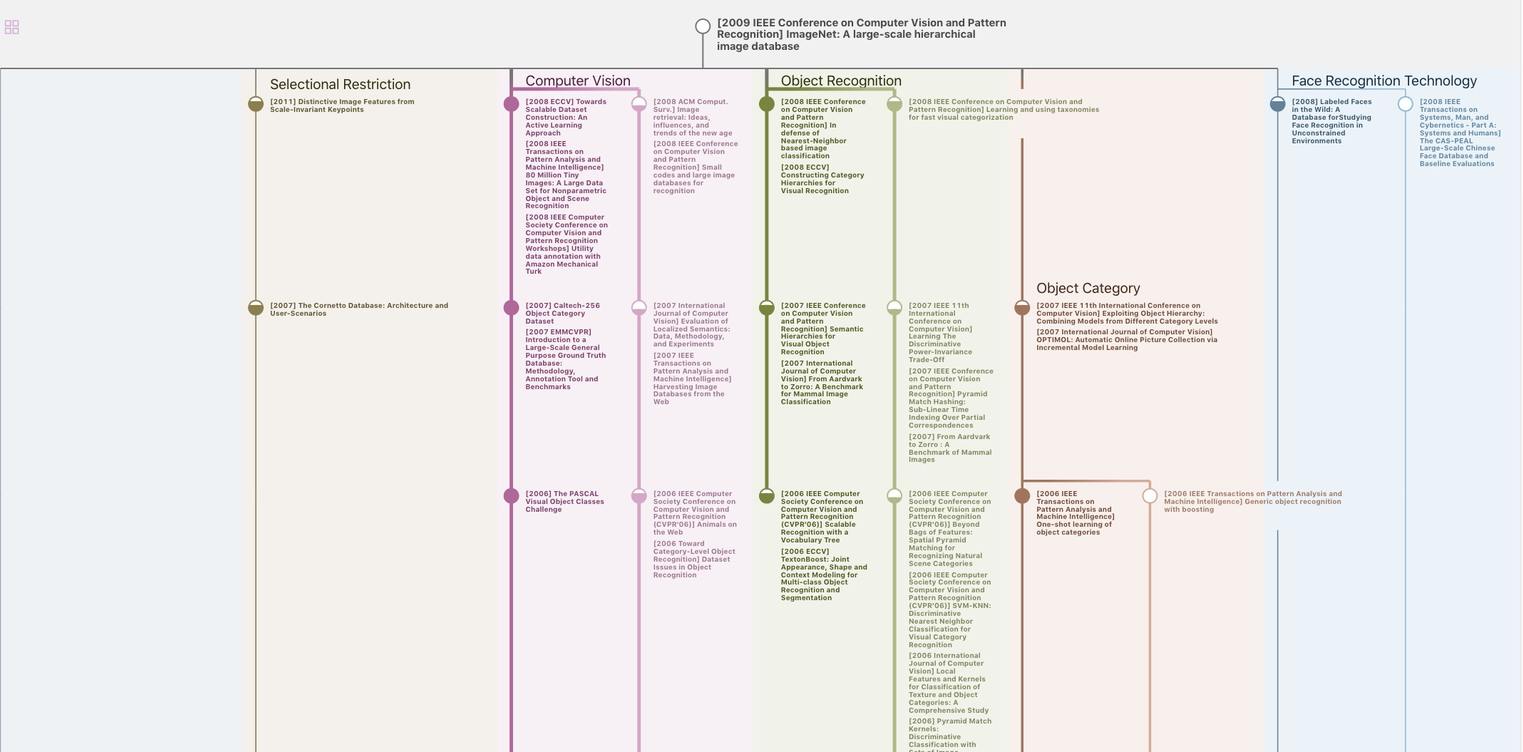
生成溯源树,研究论文发展脉络
Chat Paper
正在生成论文摘要