A Deep Reinforcement Learning Approach for Optimal Scheduling of Heavy-haul Railway
IFAC PAPERSONLINE(2023)
摘要
This paper presents an approach based on deep reinforcement learning to solve the combinatorial optimization problem of heavy-haul railway scheduling. The problem is modeled as a Markov decision process. Considering that the problem is characterized by complex process and strong constraints, a heavy-haul railway simulation environment based on discrete event scheduling is established, in which the optimization objective calculation and complex constraints can be achieved naturally. A reinforcement learning algorithm based on two-stage action sampling is proposed to alleviate the curse of dimensionality, in which invalid actions are masked out through staged action masking. The effectiveness of the approach is verified by the experimental results.
更多查看译文
关键词
deep reinforcement learning,discrete event simulation,combinatorial optimization,heavy-haul railway scheduling
AI 理解论文
溯源树
样例
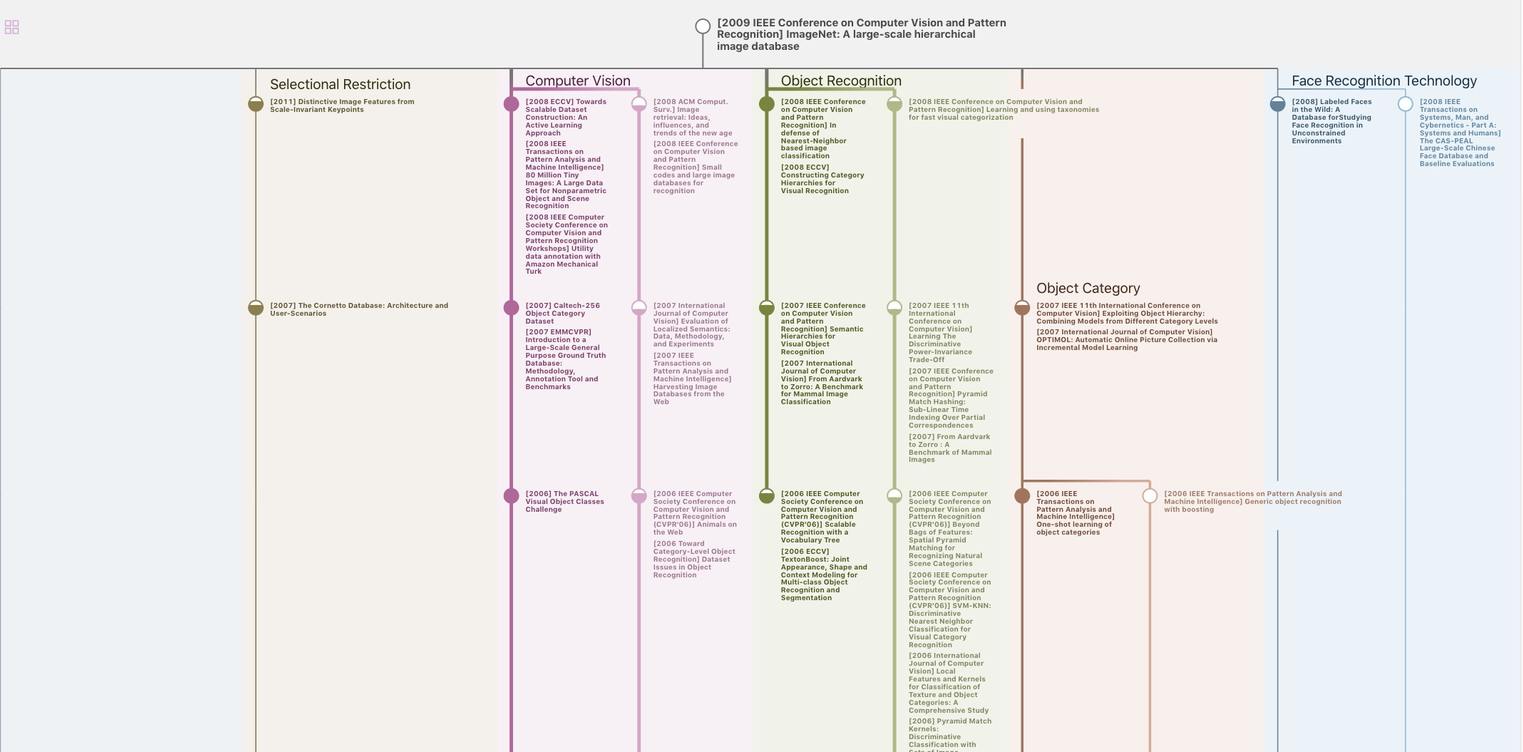
生成溯源树,研究论文发展脉络
Chat Paper
正在生成论文摘要