Bayesian model updating for bridge engineering applications based on DREAMKZS algorithm and Kriging model
STRUCTURES(2023)
摘要
The Differential Evolution Adaptive Metropolis (DREAM) based Bayesian model updating suffers from low convergence speed and high computational cost. This study proposes a Bayesian updating framework based on the DREAM and KZS strategies (DREAMKZS) assisted with the Kriging model to enhance the algorithm's convergence speed and computational efficiency. The KZS strategies represent the Kalman-inspired proposal distribution, sampling from the past states, and snooker updates. The model updating of a numerical beam and a full-scale bridge were employed to test the DREAMKZS algorithm's performance. The results demonstrated that DREAMKZS accelerates the chain exploration in high-dimensional target distributions and improves the convergence speed. The DREAMKZS outperforms the DREAM in achieving rapid convergence of the Markov chains while maintaining the precision of the posterior distribution. In the two case studies, the efficiency of model evaluation is improved by 3.66 times and 1.72 times, respectively. Compared with the traditional Bayesian approach without a surrogate model, the computational cost is reduced by 80.04% and 95.37%, respectively.
更多查看译文
关键词
Bayesian model updating,Bayesian estimation,DREAM KZS algorithm,Kriging model,Kalman-inspired proposal distribution
AI 理解论文
溯源树
样例
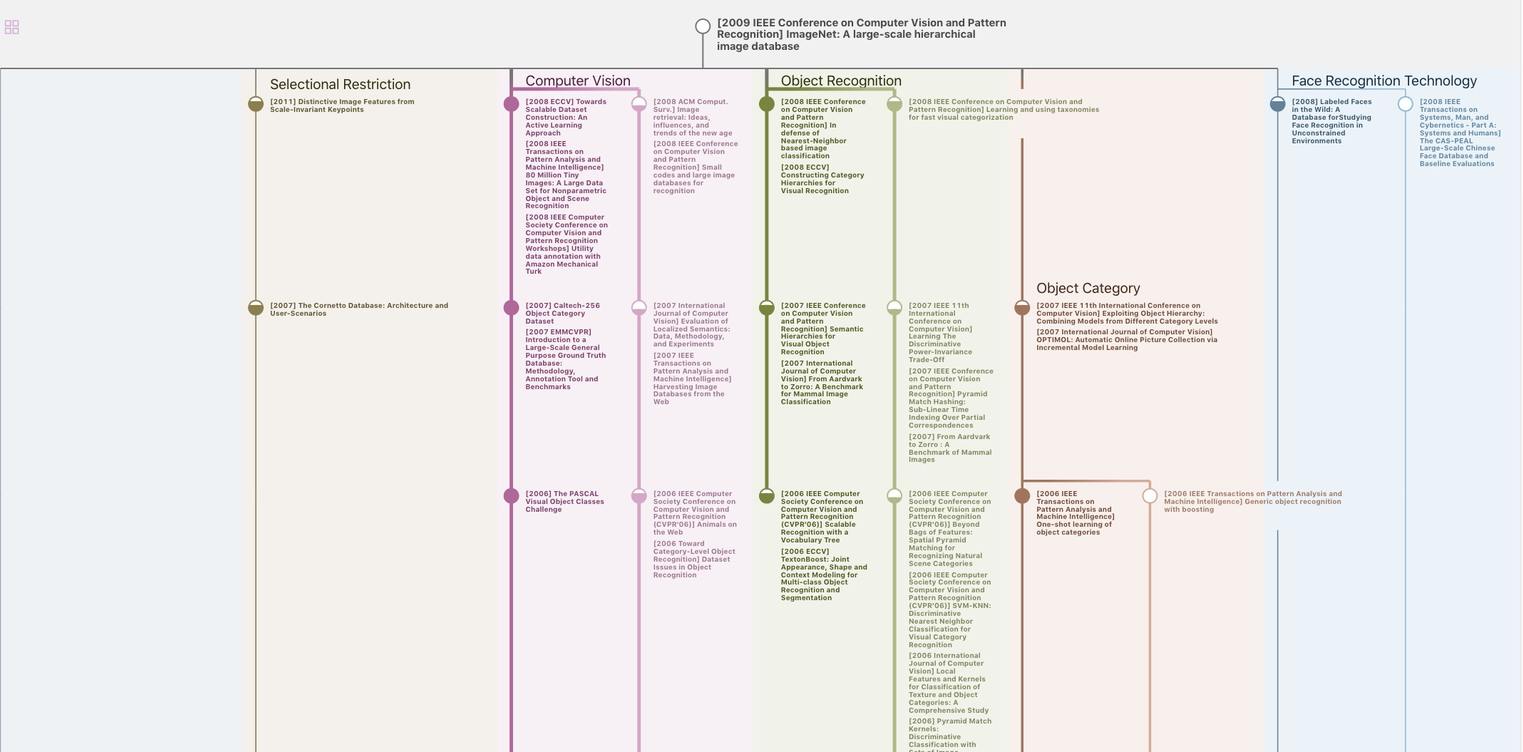
生成溯源树,研究论文发展脉络
Chat Paper
正在生成论文摘要