A unified and efficient semi-supervised learning framework for stereo matching
PATTERN RECOGNITION(2024)
摘要
Recently, stereo matching algorithms have made tremendous progress in terms of both accuracy and efficiency. However, it remains a great challenge to train a practical model due to the scarcity of ground truth disparity. In this paper, we propose a Semi-supervised Stereo Matching Framework (SSMF), i.e., a continuous self-training pipeline involving both teacher model and student model. The proposed framework combines the consistency regularization with the entropy minimization to effectively utilize the unlabeled data in large quantities. To enhance the quality of pseudo disparities: (1) we introduce an additional branch in stereo models to regress confidence map and perform adaptive selection; (2) we inject strong data augmentations on unlabeled data to achieve the prediction consistency; (3) we propose a novel loss function to utilize pseudo disparities of different confidence levels. Comprehensive experimental results show that the proposed framework enables to largely improve the disparity accuracy and robustness. Moreover, it also demonstrates competitive performance in cross-domain scenarios. Among all published methods as of August 2023, it achieves 1st on KITTI 2012 benchmark and 4th on KITTI 2015 benchmark. Code is available at https: //github.com/Twil-7/semi-supervised-stereo-matching.
更多查看译文
关键词
Stereo matching,Semi-supervised learning,Pseudo labeling
AI 理解论文
溯源树
样例
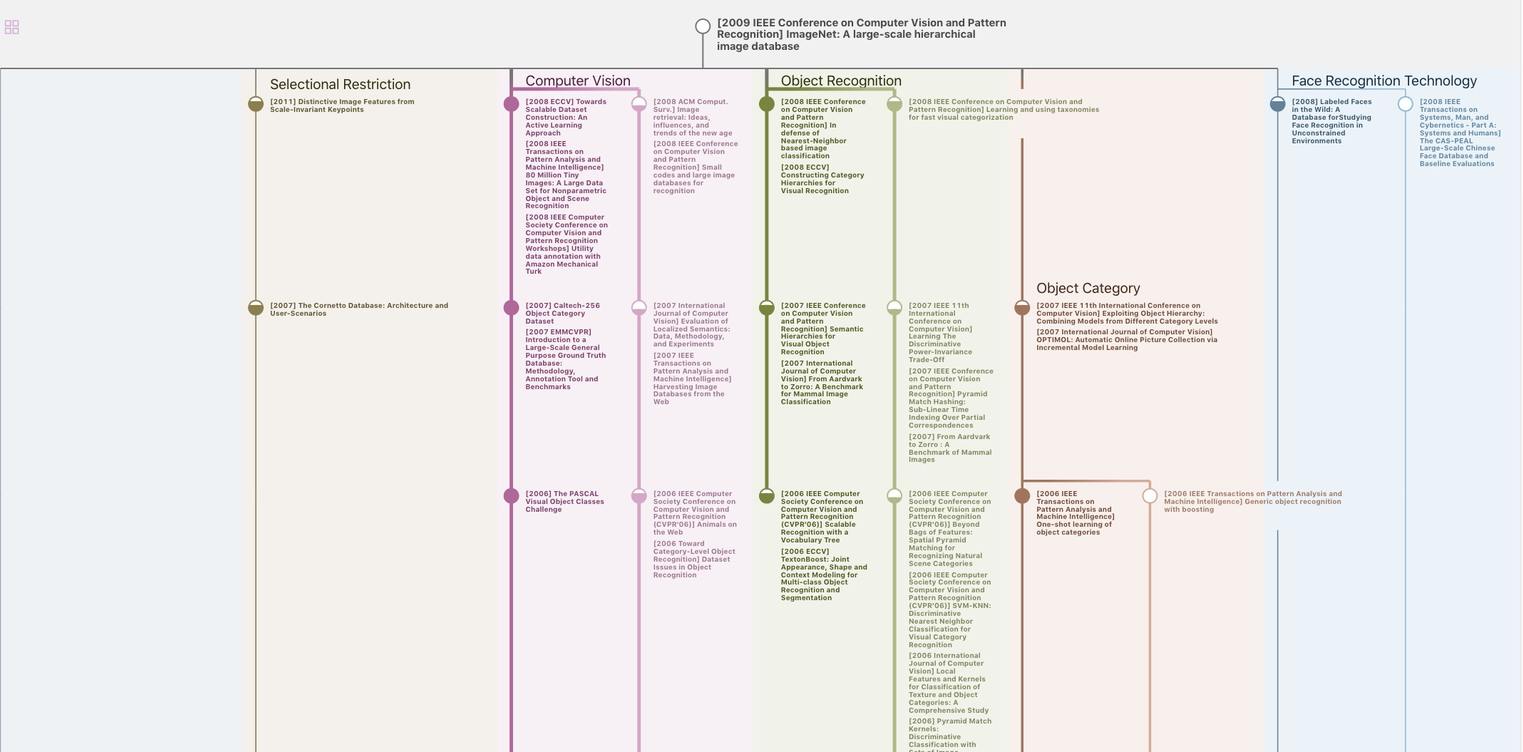
生成溯源树,研究论文发展脉络
Chat Paper
正在生成论文摘要