WSNMF: Weighted Symmetric Nonnegative Matrix Factorization for attributed graph clustering
NEUROCOMPUTING(2024)
摘要
In recent times, Symmetric Nonnegative Matrix Factorization (SNMF), a derivative of Nonnegative Matrix Factorization (NMF), has surfaced as a promising technique for graph clustering. Nevertheless, when applied to attributed graph clustering, it confronts notable challenges. These include the disregard for attributed information, the oversight of geometric data point structures, and the inability to discriminate irrelevant features and data outliers. In response, we introduce an innovative extension of SNMF termed Weighted Symmetric Nonnegative Matrix Factorization (WSNMF). This method introduces node attribute similarity to compute a weight matrix, effectively bridging the gap for attributed graph clustering. Our approach incorporates graph regularization and sparsity constraints to uphold the geometric structure of data points and discern irrelevant features and data outliers. Additionally, we present an updating rule to address optimization complexities and validate algorithmic convergence. Rigorous experimentation on real-world and synthetic networks, employing well-established metrics including F-measure, RI, Modularity, Density, and entropy, substantiates the performance enhancement offered by WSNMF.
更多查看译文
关键词
Attributed networks,Attributed graph clustering,Nonnegative matrix factorization,Symmetric Nonnegative Matrix Factorization
AI 理解论文
溯源树
样例
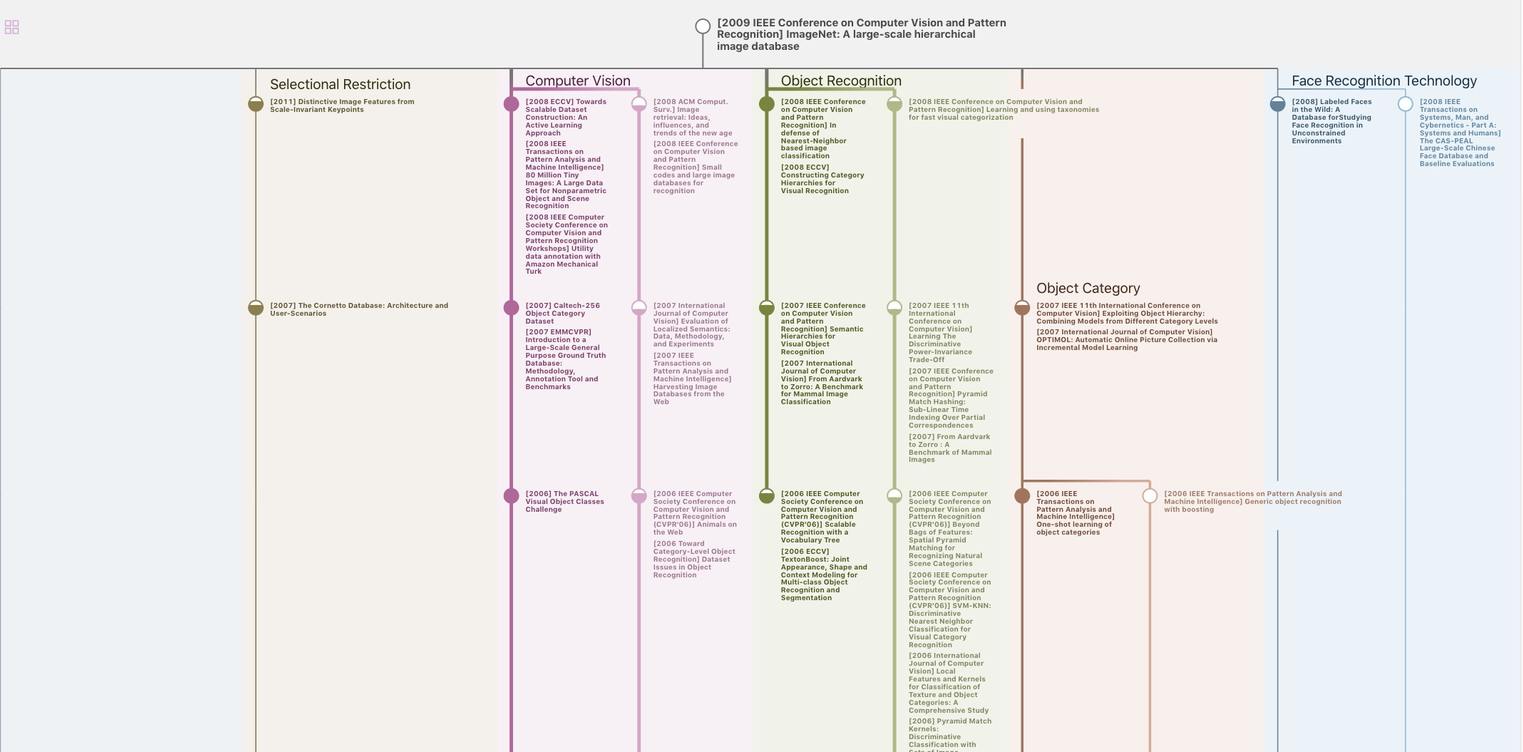
生成溯源树,研究论文发展脉络
Chat Paper
正在生成论文摘要