Combating alert fatigue with AlertPro: Context-aware alert prioritization using reinforcement learning for multi-step attack detection
COMPUTERS & SECURITY(2024)
摘要
Alert fatigue problems can have serious consequences for the enterprise security. When analysts become overwhelmed by the sheer number of alerts, high-risk alerts may go unnoticed or receive delayed responses, exposing the organization to potential cyber threats or data breaches. While current research on alert triage primarily concentrates on reducing false positives, analysts still face a shortage of resources to investigate all true alerts. The key to resolving this issue lies in the prioritization of alerts based on their potential severity, allowing analysts to allocate their efforts effectively. This paper introduces AlertPro, an alert prioritization framework that facilitates the alert triage and validation stage of typical SOC workflow. The AlertPro framework extracts context features from alert sequences and history features from alerts previously investigated by analysts, besides basic features from raw alert data. By presenting analysts with only the top-ranked potentially high-risk alerts in each query and continually updating these rankings based on feedback, AlertPro significantly streamlines the alert investigation process. To evaluate AlertPro, we conducted experiments on five datasets that are chosen or prepared specifically because they all include multi-step attacks. The results reveal that AlertPro is able to discover a previously undisclosed attack concealed within the public dataset iscx, illustrating its potential in enhancing security posture. We also evaluate the feature importance in anomaly detection and conclude that employing context features yields better performance over basic features. The paper also explores the effectiveness of incorporating history features in active learning, achieving an average improvement of 30% in attack discovery rates. The processing time of AlertPro for re-ranking and selecting high-risk alerts is within 0.5 seconds, indicating that AlertPro can effectively work in real-time scenarios. AlertPro is limited to only using partial feedback and can be improved by incorporating richer feedback from experts. Overall, AlertPro mitigates alert fatigue, enabling security analysts to concentrate their efforts on high-priority threats.
更多查看译文
关键词
Alert fatigue,Multi-step attack,Intrusion detection,Reinforcement learning,Expert feedback,Active learning
AI 理解论文
溯源树
样例
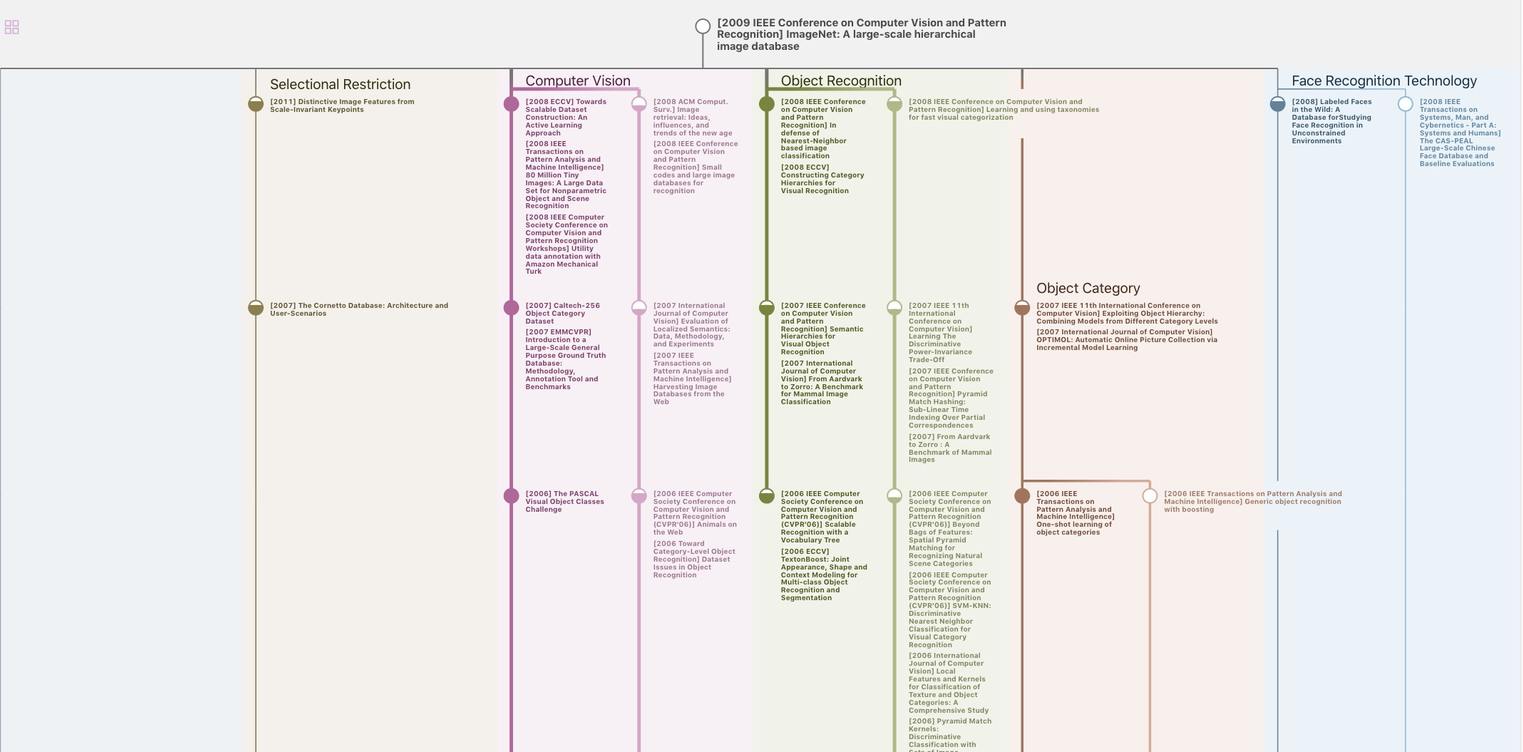
生成溯源树,研究论文发展脉络
Chat Paper
正在生成论文摘要