Enhanced non-contrast computed tomography images for early acute stroke detection using machine learning approach
EXPERT SYSTEMS WITH APPLICATIONS(2024)
摘要
Early identification of acute stroke lowers the fatality rate since clinicians can quickly decide on a quick decision of therapy. Brain computed tomography (CT) was one of the imaging techniques that were testified to be of utmost value in the evaluation of acute stroke, apart from unenhanced CT for emergency circumstances. The non -contrast image based treatment causes improper treatment of the patient. To overcome these issues, a novel hybrid image enhancement technique with an optimal feature selection based acute stroke approach was pro-posed. An advanced machine learning (ML) model was utilised to detect the stroke from the enhanced CT image. Hybrid preprocessing approaches were used to improve the contrast of collected CT images. Then, the enhanced images were segmented by using a modified active contour model, which was further proceeded for the feature extraction approach. Here, three various feature extraction approaches, local binary pattern, Gabor filter and discrete wavelet transform, were utilised to extract the features from the segmented image. Through the uti-lisation of a novel algorithm, the optimal features from the extracted images were selected. These optimal features were given to the ML model to predict the acute stroke appropriately. In order to reduce complexity and enhance the performance of a classifier, optimisation based regularisation parameters were chosen. The proposed XGBoost acute stroke detection approach provides 0.97 accuracy, 0.94 sensitivity and 0.015 false positive rate. Further, the proposed model outcome was contrasted with present models like support vector machine (SVM), artificial neural network (ANN), and naive Bayes (NB). The advanced XGBoost model provides a better contrast enhancement from the raw CT image as well as predicts acute stroke effectively.
更多查看译文
关键词
Computed tomography (CT),Optimised machine learning classifier,Hybrid preprocessing approach,Optimal features selection
AI 理解论文
溯源树
样例
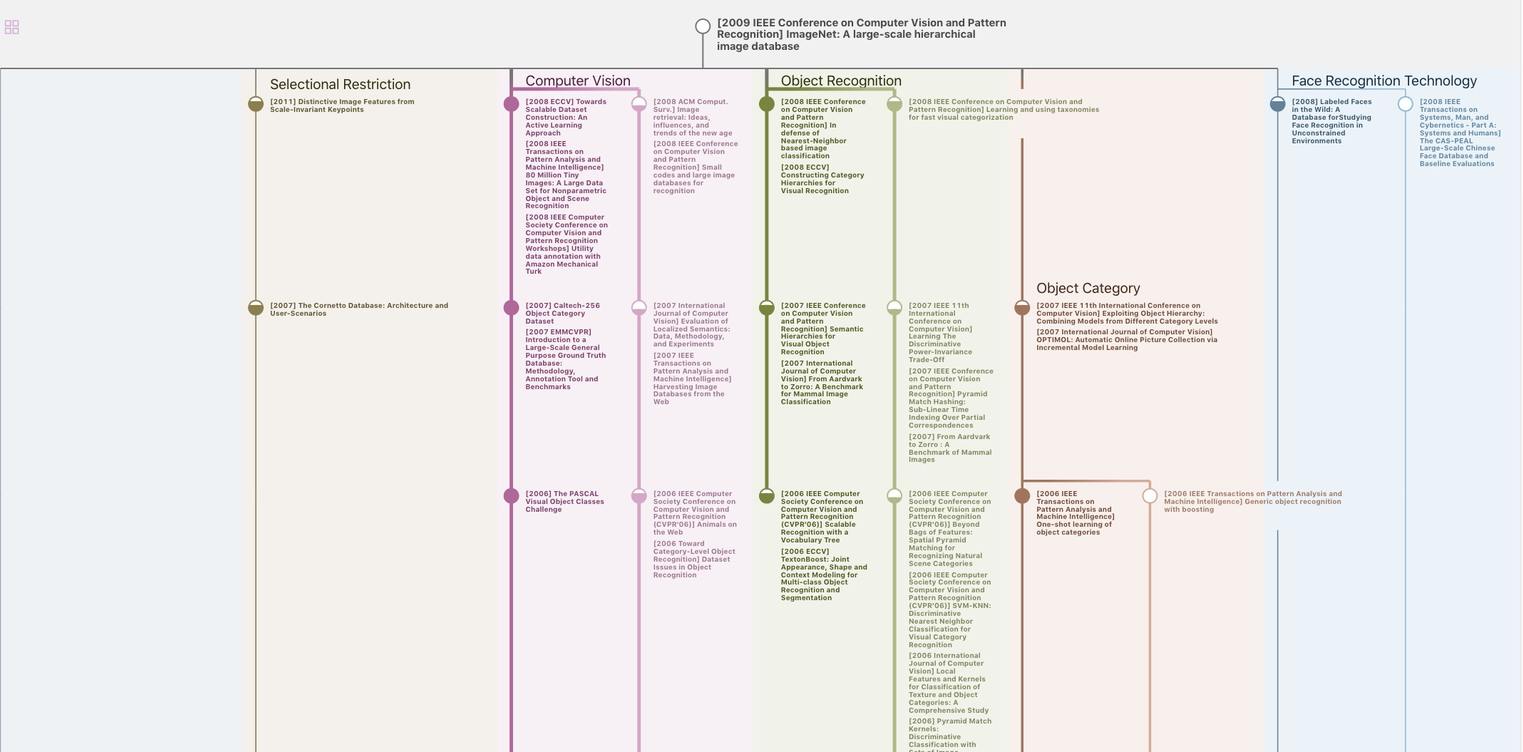
生成溯源树,研究论文发展脉络
Chat Paper
正在生成论文摘要