Neural networks informed by physics for modeling mass flow rate in a production wellbore
ENGINEERING APPLICATIONS OF ARTIFICIAL INTELLIGENCE(2024)
摘要
Optimal control strategies may efficiently manage and operate assets in contemporary oil and gas fields, lowering costs and maximizing yield. However, during this process, we encounter a traditional modeling challenge: obtaining an appropriate system representation. Concerning this task, the current research introduces a new hybrid model for simulating multi-phase mass flow in an offshore oil and gas production field. We investigate a concept based on Physics-Informed Neural Networks (PINN) to characterize the mass flow dynamics in a production wellbore. PINN models have been demonstrated as a feasible alternative for training neural networks using a small number of training data samples and estimating the parameters of physics equations that guide the underlying dynamics. The concept might provide alternatives to modeling and balancing the task of learning from physics and data in oil-gas systems. This research analyzes the performance of neural networks inspired by physics in a synthetic database constructed from the physics laws of mass conservation and conservation of momentum. After solidifying the principles, the study assessed the power of model assimilation in a real-world oilfield situation.
更多查看译文
关键词
Hybrid modeling,Physics informed neural networks,Mass flow rate
AI 理解论文
溯源树
样例
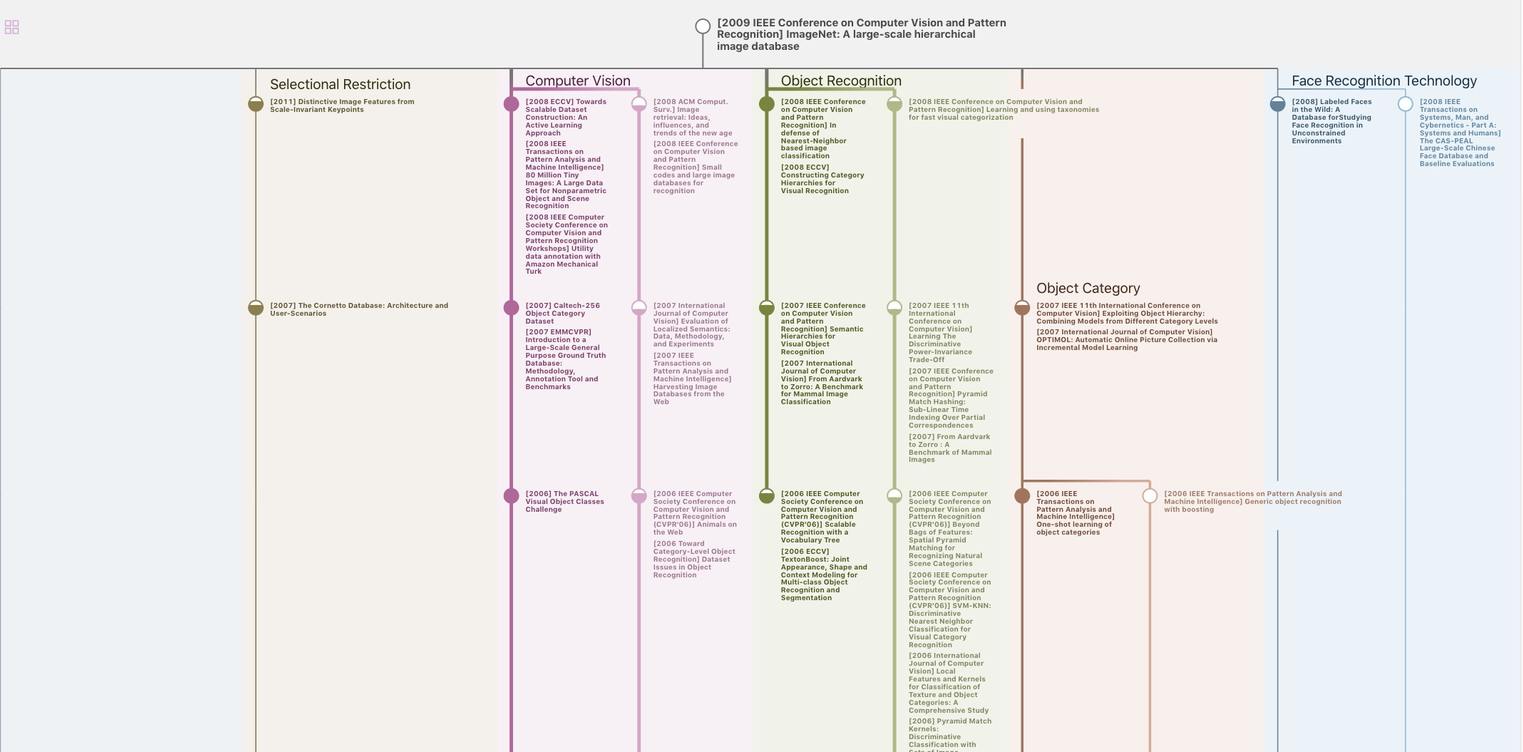
生成溯源树,研究论文发展脉络
Chat Paper
正在生成论文摘要