Machine learning-based optimization of segmented thermoelectric power generators using temperature-dependent performance properties
Applied Energy(2024)
摘要
Segmented thermoelectric generators (STEGs) provide an excellent platform for thermal energy harvesting de-vices because they improve power generation performance across a broad range of operating temperatures.Despite the benefit of direct thermal energy-to-electricity conversion, conventional STEG optimization approaches are unable to provide a systematic method for selecting the optimal multiple stacks of p-and-n-type thermoelectric materials (TEs) legs from a set of numerous TE materials. In this study, we propose a systematic optimization method based on machine learning to find the optimal STEG for performance maximization. A deep neural network (DNN) is trained using an initial dataset generated via the Finite Element Method (FEM), with inputs including the temperature-dependent properties of p-and n-type TE materials, the lengths of each segment, and external loads, as well as the corresponding per-formance outputs. The trained DNN captures the inherent nonlinear relationship between these inputs and outputs. The combination of a trained DNN and a genetic algorithm (GA) efficiently navigates a vast design space using 88 p-type and 70 n-type TE materials along with device factors. It formulates four stacked segment pairs in p-and n-leg TEGs, targeting new superior designs with enhanced power, efficiency, or both. The DNN is iteratively refined via active learning (AL) by incorporating new superior STEG designs to enhance prediction ac-curacy. The optimized STEGs exhibit power and efficiency that are 1.91 and 1.5 times higher, respectively, than the top designs from the initial training dataset composed of 157.916 STEGs. Furthermore, compared to conventional TEG designs without segmentation, our method discovered numerous high-performing STEG designs.
更多查看译文
关键词
Thermoelectric,COMSOL-Multiphysics,Deep neural network,Genetic algorithm active learning
AI 理解论文
溯源树
样例
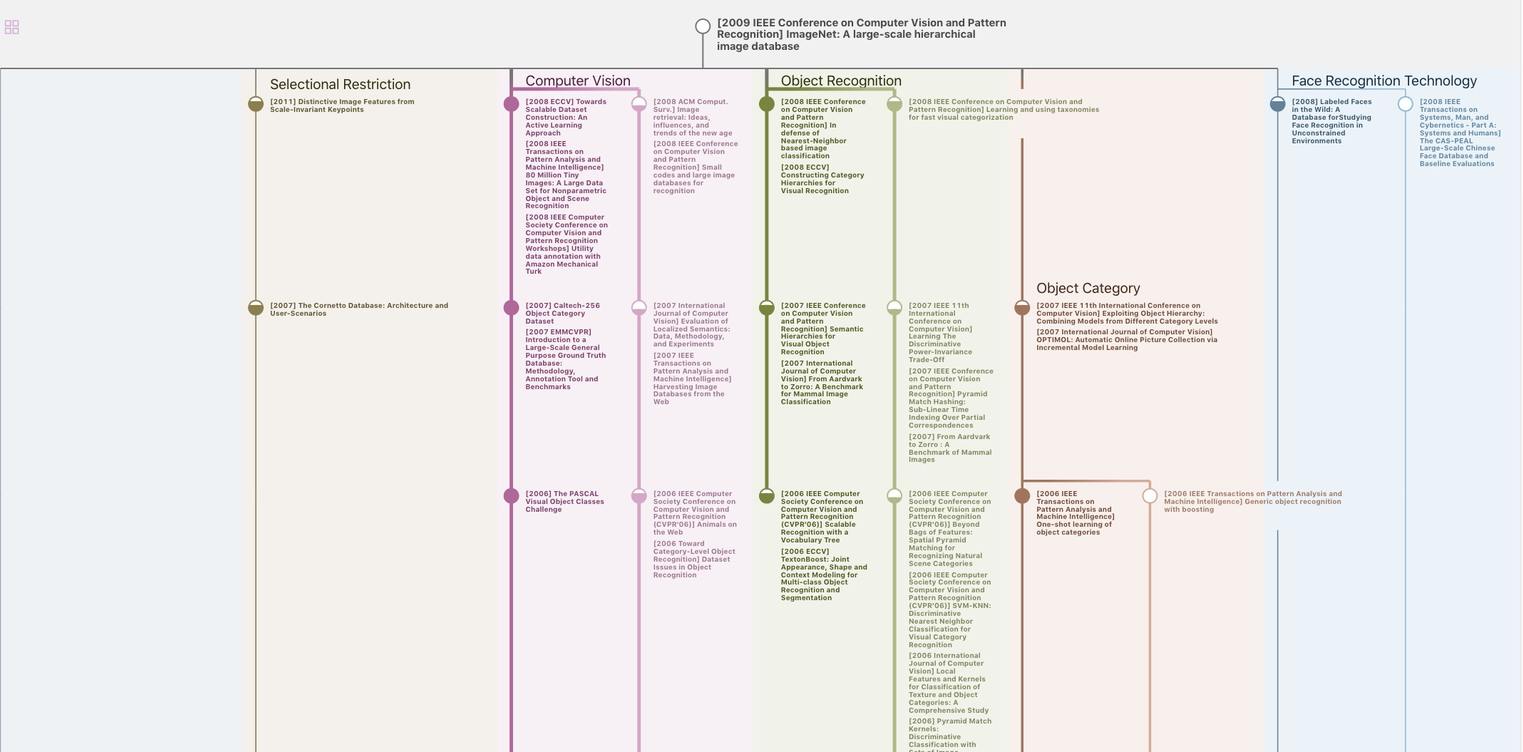
生成溯源树,研究论文发展脉络
Chat Paper
正在生成论文摘要