Attention Cycle-consistent universal network for More Universal Domain Adaptation
PATTERN RECOGNITION(2024)
摘要
Existing Universal Domain Adaptation (UniDA) approaches can handle various domain adaptation (DA) tasks, which need no prior information about the category overlap across target and source domains. However, traditional UniDA scenario cannot fully cover every DA scenario, e.g., Multi-Source DA is absent. Therefore, aiming to simultaneously handle more DA scenarios in nature, we propose the More Universal Domain Adaptation (MUniDA) task. There are three challenges in MUniDA: (i) Category shift between source and target domains; (ii) Domain shift, especially the domain shift among multiple modalities in the source, which is ignored by the current UniDA approaches; (iii) How to recognize common categories across domains? We propose a more universally applicable DA approach that can tackle above challenges without any modification called Attention Cycle-consistent Universal Network (A-CycleUN). We show through extensive experiments on several benchmarks that A-CycleUN works stably and outperforms baselines across different MUniDA settings.
更多查看译文
关键词
Domain adaptation,RGB-D data,Visual categorization,Unequal category
AI 理解论文
溯源树
样例
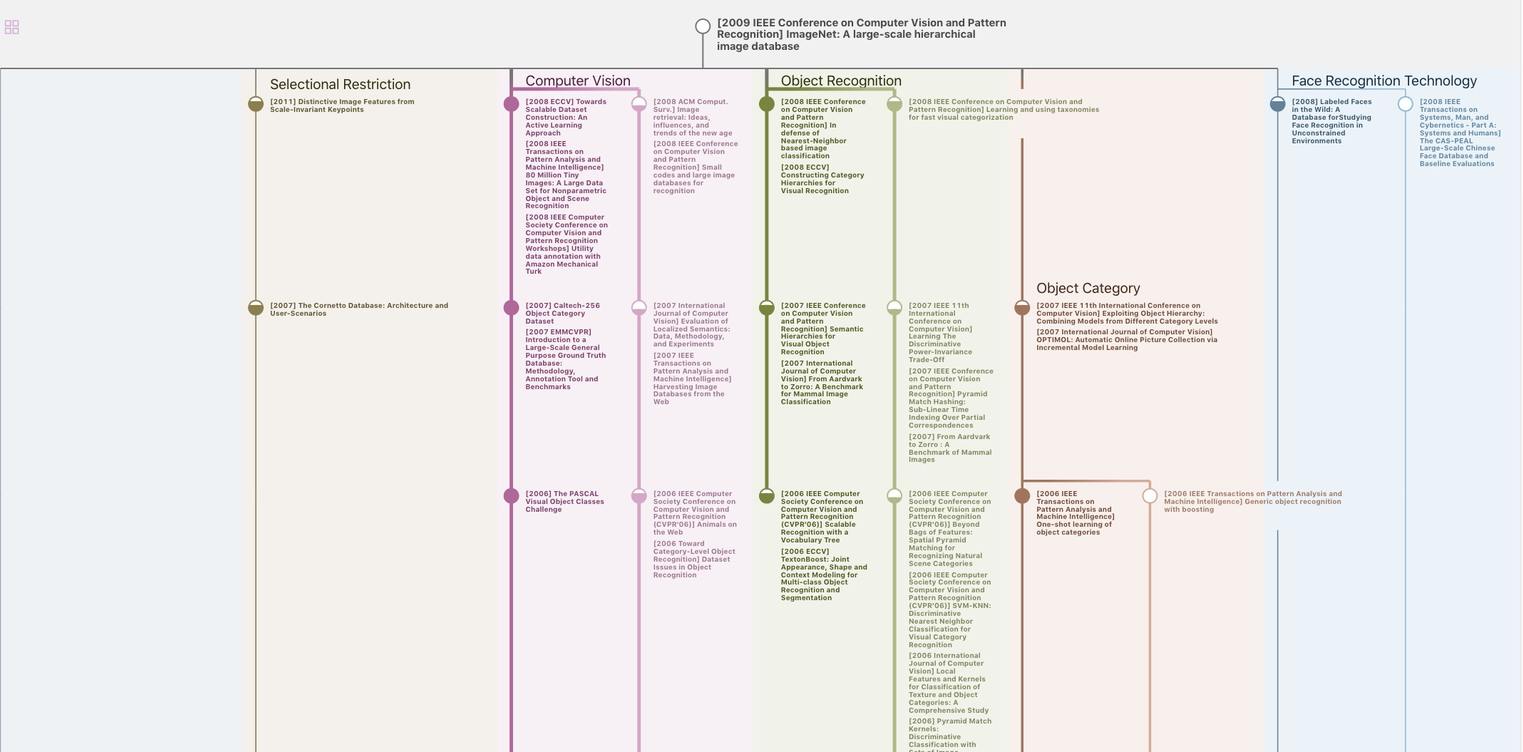
生成溯源树,研究论文发展脉络
Chat Paper
正在生成论文摘要