Dynamic building defect categorization through enhanced unsupervised text classification with domain-specific corpus embedding methods
AUTOMATION IN CONSTRUCTION(2024)
摘要
Large amounts of data are often categorized using different systems. In such cases, few-shot and unsupervised text classification are the two main approaches for dynamically classifying text into a single classification. Unsupervised text classification typically exhibits lower performance but requires significantly less data preparation effort and computing resources than the few-shot approach. This study proposes two methods to enhance unsupervised text classification for domain-specific non-English text using improved domain corpus embedding: 1) weighted embedding-based anchor word clustering (wean-Clustering), and 2) cosine-similarity-based classification using a defect corpus that is vectorized by fine-tuned pretrained language models (sim-ClassificationftPLM). The proposed methods were tested on 40,765 Korean building defect complaints and achieved F1 scores of 89.12% and 84.66% respectively, outperforming the state-of-the-art zero-shot (53.79%) and few-shot (72.63%) text classification methods, with minimal data preparation effort and computing resources.
更多查看译文
关键词
Dynamic text classification,Unsupervised text classification,Domain corpus embedding,Clustering,Text similarity,Few-shot learning,Zero-shot learning,Building defect management
AI 理解论文
溯源树
样例
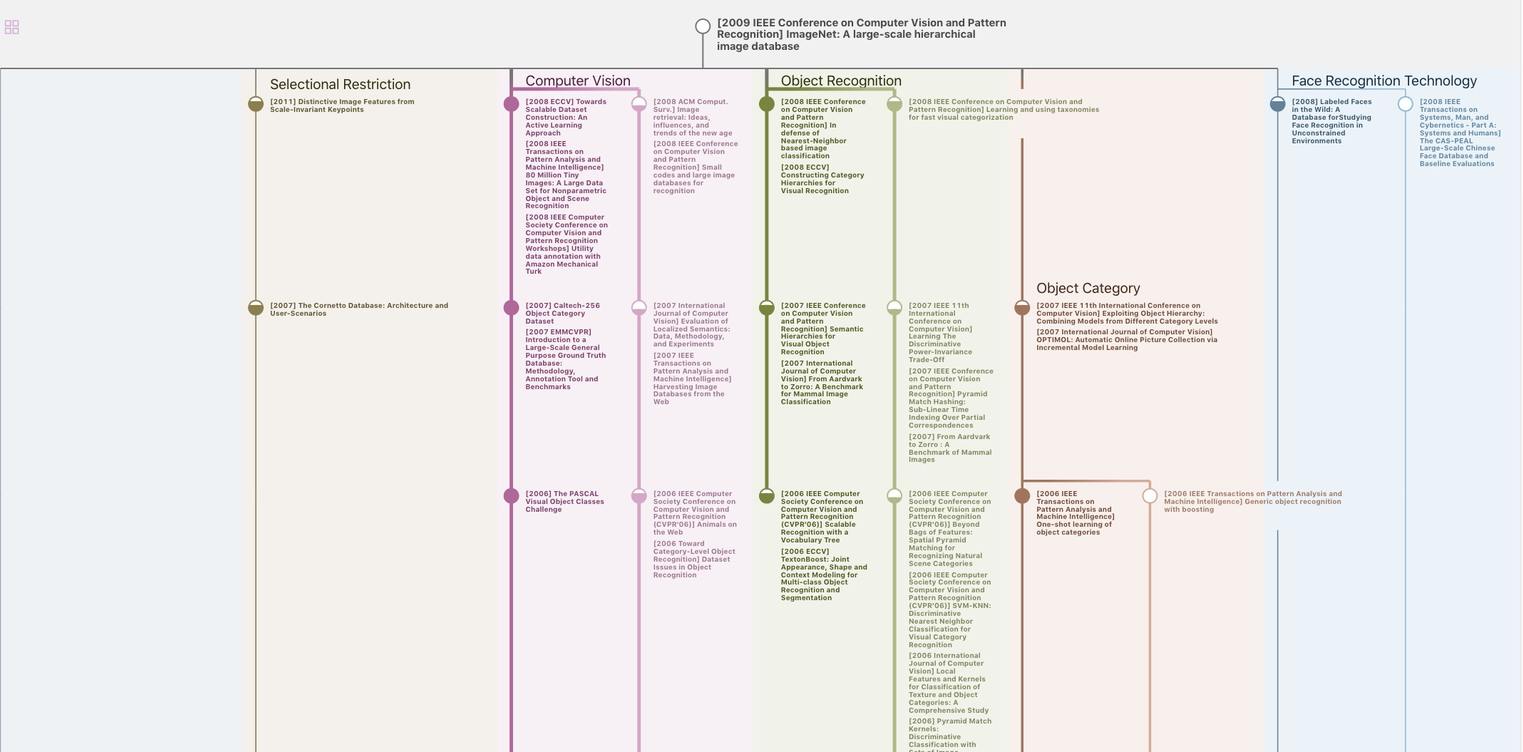
生成溯源树,研究论文发展脉络
Chat Paper
正在生成论文摘要