Monocular depth estimation using self-supervised learning with more effective geometric constraints
ENGINEERING APPLICATIONS OF ARTIFICIAL INTELLIGENCE(2024)
摘要
Self -supervised learning -based depth estimation from monocular videos is a challenging yet promising way for 3D environment perception. Existing methods that use photometric consistency as supervision are often fragile in the case of textureless environments or illumination variations. In this paper, we adopt geometric constraints to improve the reliability and accuracy of monocular depth estimation. We introduce an equation derived from the two -view imaging geometry, from which we also develop a novel geometric loss function that is shown to be more effective than the epipolar constraint in self -supervised learning. In addition, we design a depth reconstruction loss by explicitly aligning the depth scale of the consecutive estimated depth maps to keep the depth scale consistent. Finally, a new self -supervised learning framework is formulated by incorporating the proposed geometric constraints into depth, pose, and optical flow estimation models. Extensive experiments on KITTI, Make3D, and NYU Depth v2 datasets show that, by adding our geometric constraints, the depth estimation model compares favorably with state-of-the-art self -supervised learning methods, and each sub -task has a significant improvement.
更多查看译文
关键词
Monocular depth estimation,Self-supervised learning,Geometric constraints,Two-view imaging geometry,Pure rotation
AI 理解论文
溯源树
样例
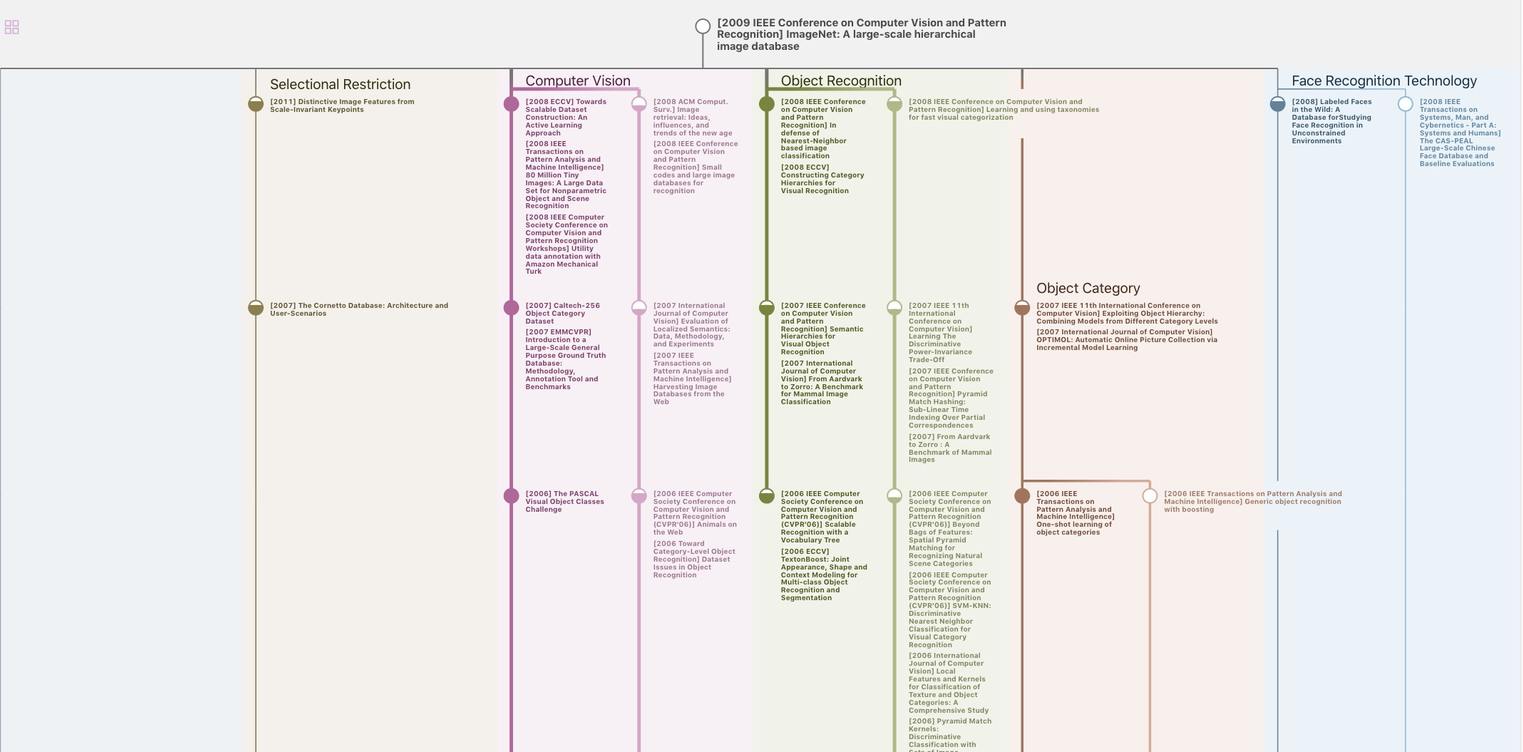
生成溯源树,研究论文发展脉络
Chat Paper
正在生成论文摘要