Time-aware personalized graph convolutional network for multivariate time series forecasting
EXPERT SYSTEMS WITH APPLICATIONS(2024)
摘要
The use of graph structures to model variable dependencies in multivariate time series data holds promise. Probabilistic graph models, sampling discrete graphs from neural network-parameterized distributions, have shown efficacy. However, the current approaches ignore that dependencies are dynamic and constrained by heterogeneous information. For instance, interactions among traffic data's roads are constrained by traffic volume and network topology. Furthermore, the existing spatio-temporal graph propagation mechanism has failed to account for the impact of the evolutionary patterns of the nodes themselves on future outcomes. To address these limitations, this research introduces a novel approach: a time-aware personalized graph convolutional network (TPGCN) consisting of time-aware discrete graph structure estimation (TADG) and dynamic personalized graph convolutional (DPGC) components. The TADG module confronts the challenge of graph learning by framing it as an inference issue within a heterogeneous information landscape. Through dynamic inputs, it deduces graph structures that are influenced by the varying nature of interactions. DPGC, drawing inspiration from personalized PageRank, models variable effects while considering evolutionary patterns and external factors. By dynamically fusing these two types of information at every information aggregation, our approach outperforms existing state-of-the-art methods, as demonstrated on eight benchmark datasets.
更多查看译文
关键词
Multivariate time series forecasting,Probabilistic graph models,Heterogeneous information,Dynamic graph learning,Personalized pageRank
AI 理解论文
溯源树
样例
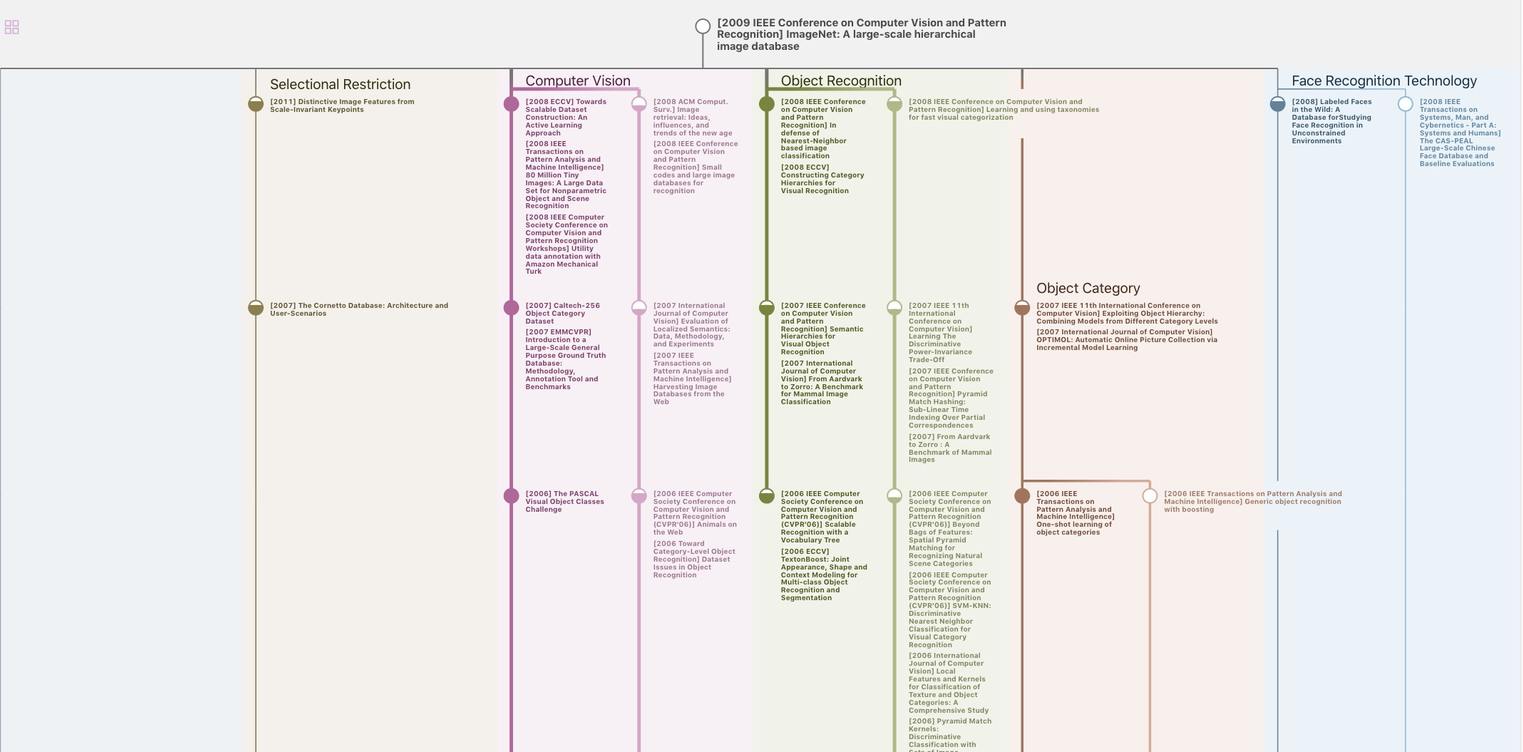
生成溯源树,研究论文发展脉络
Chat Paper
正在生成论文摘要