Correlated differential privacy based logistic regression for supplier data protection
COMPUTERS & SECURITY(2024)
摘要
As a crucial participant in the supply chain, the supplier's every action affects the supply chain's status, making predictions about whether a supplier will be listed or not essential. However, the large amount of sensitive data used in machine learning generates the problem of privacy leakage. Due to the data relevance, traditional differential privacy is prone to leakage of information of correlated data. To effectively tackle this problem, in the scenario of supplier listing prediction, we introduce the correlated differential privacy mechanism for the logistic regression model, propose the feature selection scheme DC-FBFS, and further explore different noise addition methods. The experiments show that the proposed scheme can improve the utility of data, increase the prediction accuracy, and reduce the error in data query while effectively protecting data.
更多查看译文
关键词
Correlated differential privacy,Feature selection,Logistic regression,Supplier data,Privacy-preservation
AI 理解论文
溯源树
样例
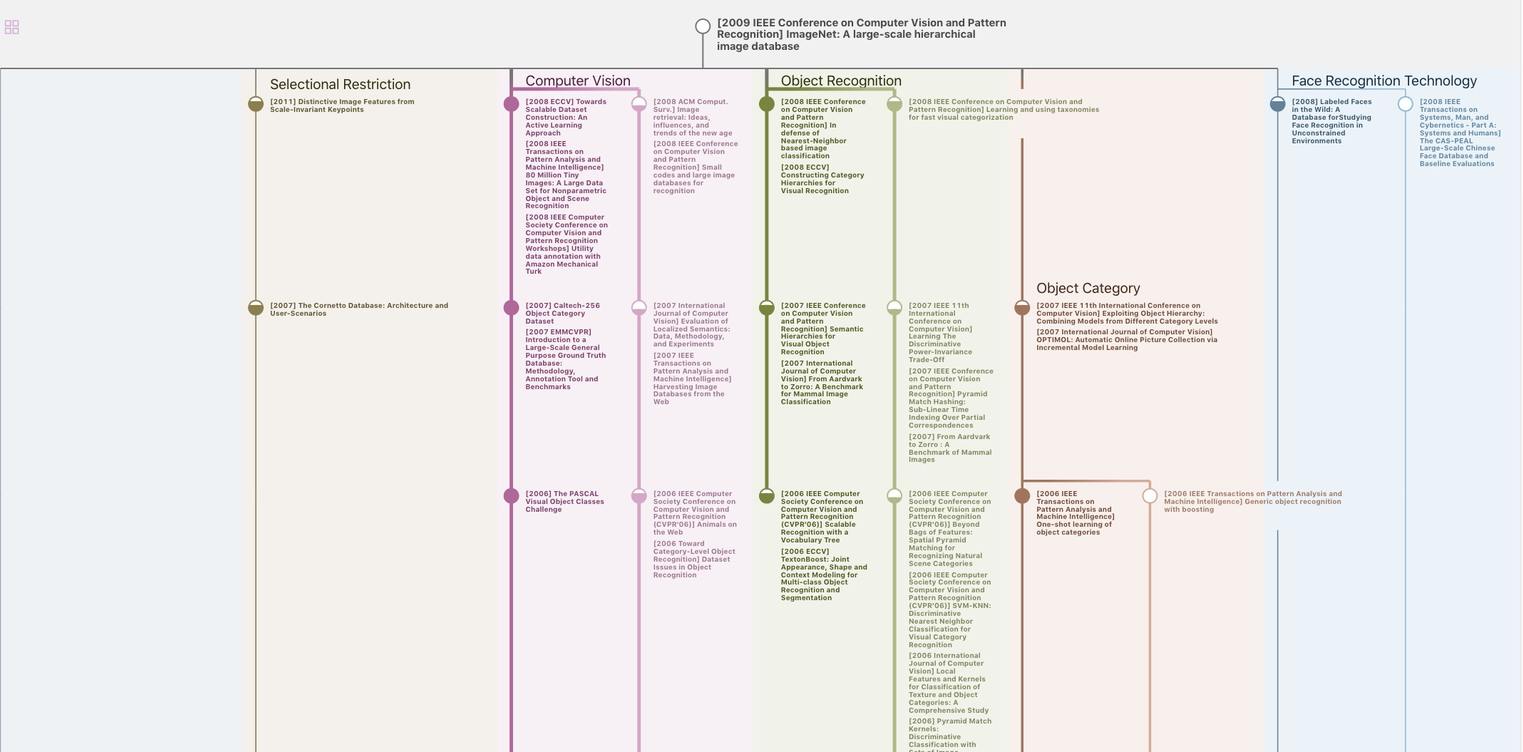
生成溯源树,研究论文发展脉络
Chat Paper
正在生成论文摘要