Branch-cut phase unwrapping method based on deep learning
Optik(2023)
摘要
As a typical representative of the phase unwrapping algorithm, the branch-cut method has good anti-noise performance in the phase unwrapping process. However, the branch-cut generated by the traditional branch-cut method cannot accurately block error propagation. This study proposes a branch-cut phase unwrapping method based on deep learning, which transforms the traditional problem of connecting residual points into a semantic segmentation learning problem, to improve the blocking effect of the branch-cut to the error. The article analyzes the performance of modulation and residue maps in representing phase errors and finds that residue maps have a poor perception of error information in shadow areas in the wrapped phase map. Therefore, a new branch-cut connection criterion is proposed that combines modulation maps and sets the bound of shadow and non-shadow areas as part of branch-cut. Positive and negative residual points are connected by curves along the path of poor modulation, and their polarities are accumulated to maintain branch-cut balance. By using the new branch-cut connection criterion to provide learning samples and using the U-Net++ network structure as the deployment network of branch-cut, the network generates a branch-cut for phase unwrapping, which phase unwrapping path bypasses the branch-cut. The experimental results show that the deployment of branch-cut using the U-Net++ network structure can achieve the expected training effect, and the branch-cut generated based on the new connection principle can more effectively block the propagation of errors during phase unwrapping.
更多查看译文
关键词
deep learning,phase,branch-cut
AI 理解论文
溯源树
样例
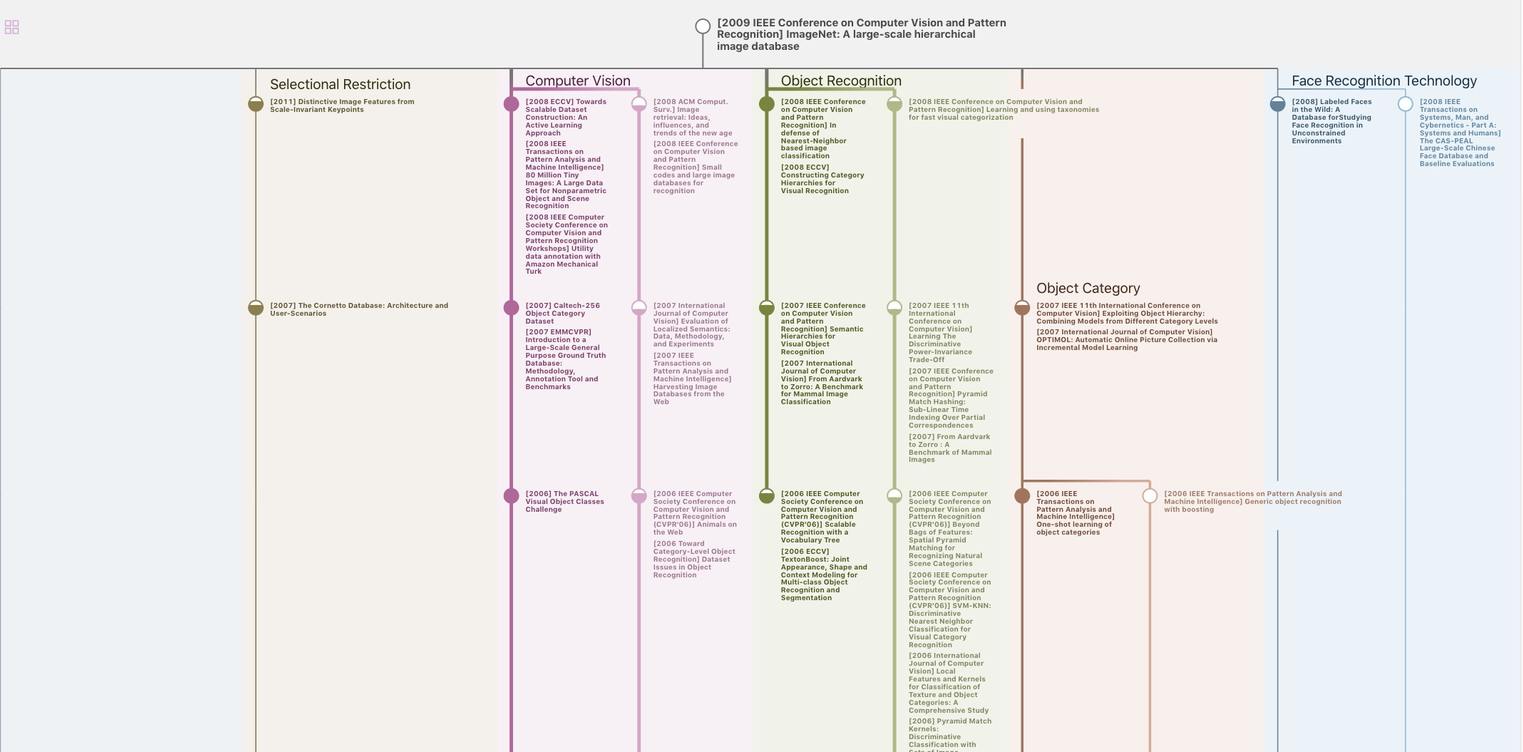
生成溯源树,研究论文发展脉络
Chat Paper
正在生成论文摘要