Dynamic hypergraph convolutional network for multimodal sentiment analysis
NEUROCOMPUTING(2024)
摘要
Multimodal sentiment analysis (MSA) aims to detect the sentiments from language (text), audio, and visual (facial expressions) modalities. The main challenge in MSA is how to efficiently model intra-modality and inter-modality dynamics. With the advent of graph convolution network (GCN), graph-based models are proposed to solve the challenge. However, general graphs contain only two nodes per edge, which limits the exploitation of high-order interactions. Moreover, current graph-based models mainly aggregate the features of each node during fusion, while the features of connected edges are not well mined. In this paper, we introduce dynamic hypergraph convolution networks to MSA for the first time and propose a Multimodal Dynamic Hypergraph Network (MDH) to learn intra-and inter-modality dynamics. Hypergraphs provide a natural approach to capture transcendental pairwise relations, and their potential for MSA remains unexplored. MDH mainly consists of three components: Unimodal Encoder, Dynamic Hypergraph Enhancement Network (DHEN), and HyperFusion module. Specifically, DHEN is composed of Cross-modal Affine, Hypergraph Construction, and Hypergraph Aggregation modules. As for the intra-modality dynamics, MDH utilizes Hypergraph Construction and Aggregation modules to model the interactions within time steps for each modality. As for the inter-modality dynamics, MDH implements Cross-modal Affine and HyperFusion modules to learn the relationships of the modalities. In addition, multi-task learning has been implemented to optimize the learning process for multimodal tasks. Experiments show that MDH outperforms graph-based models on CMU-MOSI and CMU-MOSEI datasets, as well as obtains new state-of-the-art results on CH-SIMS dataset. Furthermore, we conduct external experiments to explore the effectiveness of MDH and the effect of model depth with different graph networks.
更多查看译文
关键词
Multimodal sentiment analysis,Dynamic hypergraph network,Multi-task learning
AI 理解论文
溯源树
样例
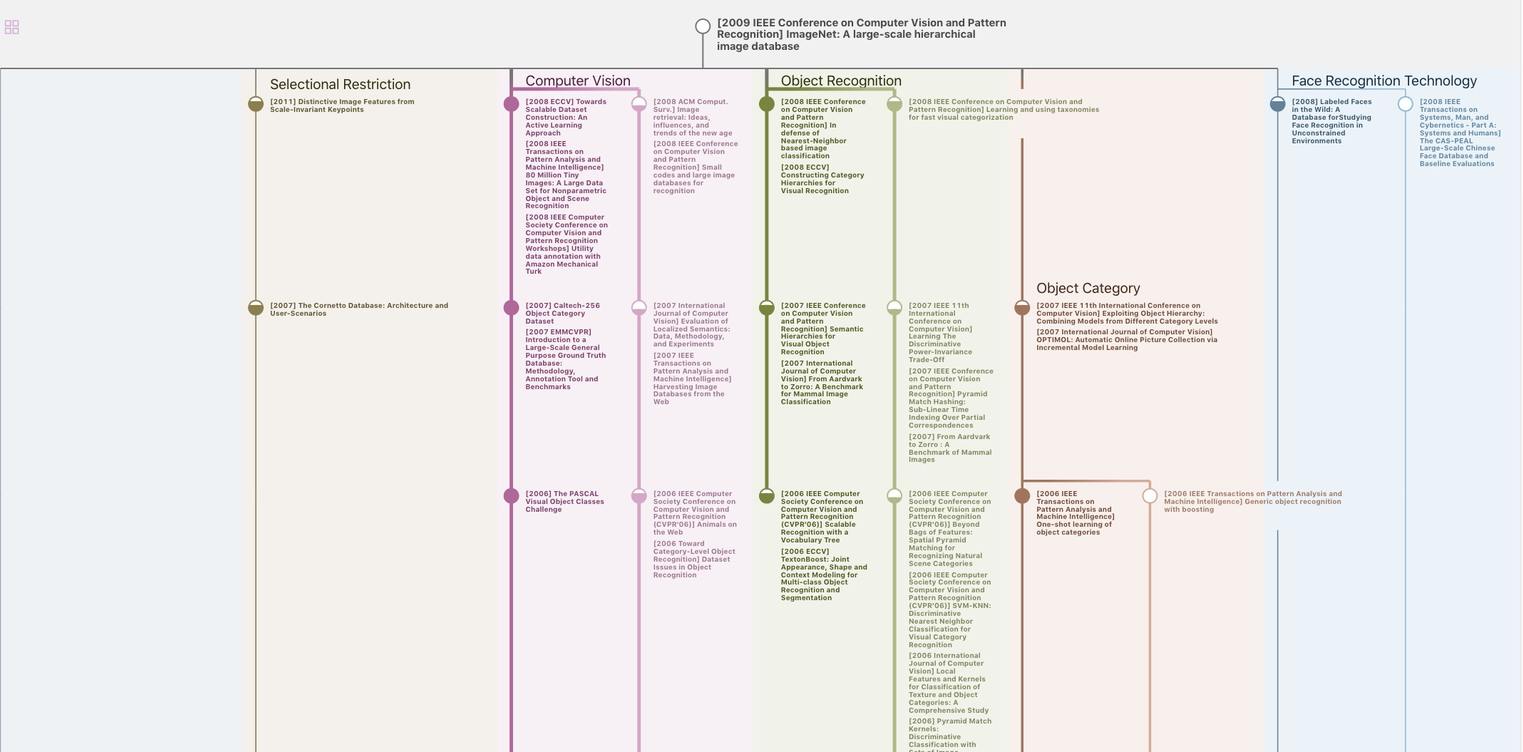
生成溯源树,研究论文发展脉络
Chat Paper
正在生成论文摘要