Defect detection of MicroLED with low distinction based on deep learning
OPTICS AND LASERS IN ENGINEERING(2024)
摘要
MicroLED has great prospects in visible light communication, optical detection and other fields. To solve limited efficiency and reliability issues caused by current manual quality control processes in MicroLED production environments, we propose a micro-vision-based automatic scanning system (MVASS) used to capture the MicroLED defect images and performing defect prediction inference. Since MicroLED defects are weak, they are very similar to normal MicroLEDs with low distinction, and the large number of MicroLEDs in an image, making a poor detection effect for the existing method. In this paper, a real-time detection method named AMS-YOLO base on lightweight deep convolutional neural network for MicroLED defects with low-distinction is proposed, utilizing the attention residual module (ARM) to extract multi-level effective features by focusing on the key features in the defect images by self-attention, and introducing the multi-scale feature fast fusion module (M3FM) to fuses the multi-level feature effectively and quickly to better capture the global information of the defect image, and using the selective feature pyramid module (SFPM) to selectively fuse the extracted features to enhance the network's classification and positioning capabilities and ultimately achieve precise MicroLED classification and detection. Experimental results on the MicroLED dataset show that AMS-YOLO demonstrates a better performance, with the mAP, Precision, Recall and speed increasing by 3.0 %, 3.5 %, 6.0 % and 4.3 % respectively, compared to the YOLOv5s.
更多查看译文
关键词
Low-distinction defect detection,Visual system,MicroLED,Deep learning,Self-attention
AI 理解论文
溯源树
样例
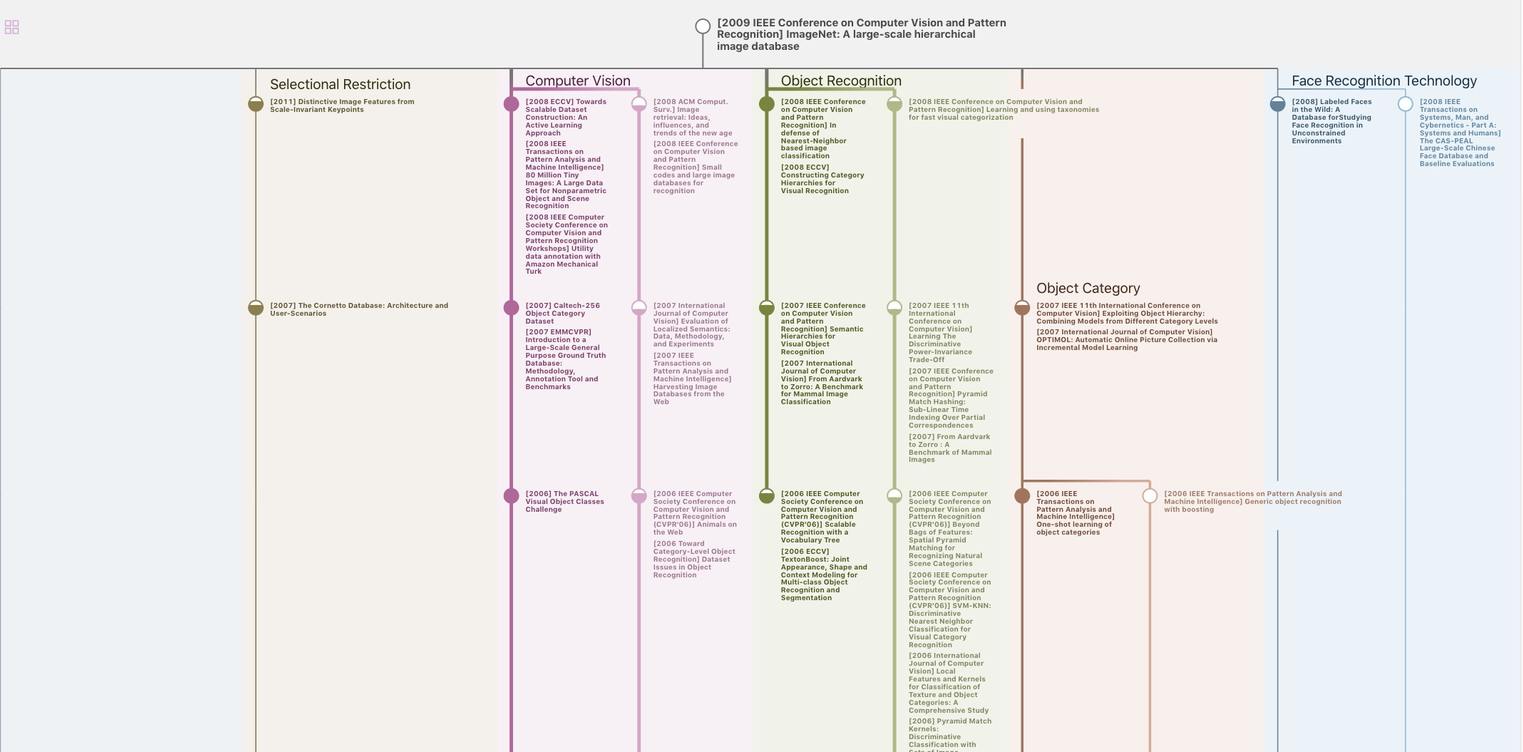
生成溯源树,研究论文发展脉络
Chat Paper
正在生成论文摘要