Bayesian averaging-enabled transfer learning method for probabilistic wind power forecasting of newly built wind farms
APPLIED ENERGY(2024)
摘要
This paper proposes a technique for the probabilistic wind power forecasting (WPF) of a newly built wind farm (NWF) using a limited amount of historical data. First, the state-of-the-art Transformer network is employed to capture the power generation pattern of different wind farms (WFs) based on abundant historical training samples. Then, the Bayesian averaging regression method is applied to transfer the learned power generation pattern to the NWF by assigning proper weights to the WPF results of different WFs. This enables the proposed method to yield accurate NWF power predictions utilizing a limited amount of historical data. The Bayesian characteristics further enable the quantification of multiple uncertainties in forecasting results that may be essential for the NWF operator when the input is uncertain. Comprehensive tests were also performed by employing other deterministic and probabilistic WPF methods using field data. By comparing the results, the proposed method is demonstrated to produce accurate forecasting results with sparse historical data. Moreover, the uncertainties of outcomes are quantified, and acceptable performance is achieved.
更多查看译文
关键词
Probabilistic wind power forecasting,Newly built wind farm,Transformer network,Bayesian averaging regression
AI 理解论文
溯源树
样例
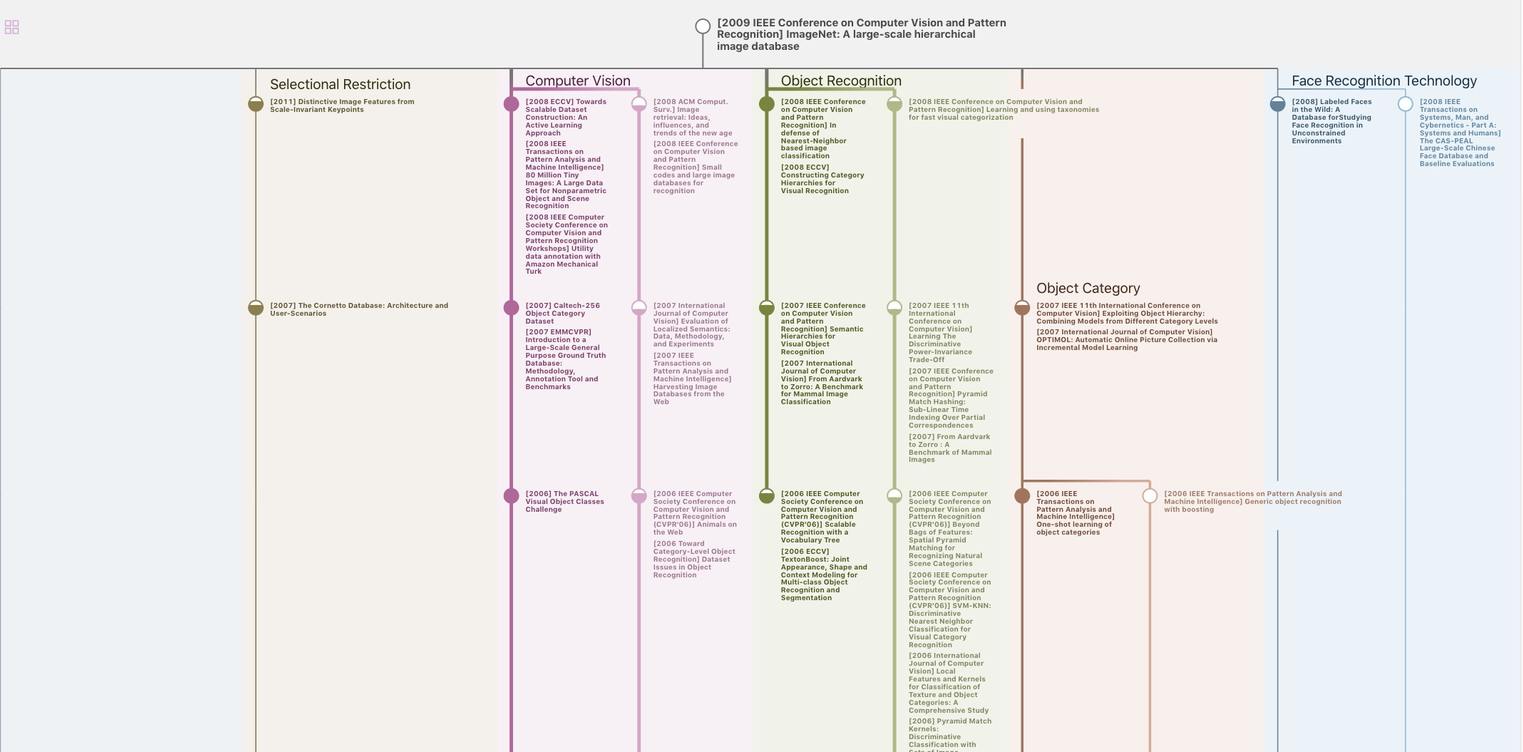
生成溯源树,研究论文发展脉络
Chat Paper
正在生成论文摘要