TE process fault diagnosis based on KPCA-RF
2023 35TH CHINESE CONTROL AND DECISION CONFERENCE, CCDC(2023)
摘要
For the safety of automatic industry processing, the prediction and analysis of abnormal conditions of field equipment fast and accurately is of great significance. The KPCA-RF fault diagnosis approach, which is based on the kernel principal component analysis (KPCA) and the random forest (RF), is developed in this study to address the issues with the mainstream fault diagnosis method in the Tennessee-Eastman (TE) process. High-dimensional raw data must be feature extracted with KPCA before time series based nonlinear feature data can be obtained. The RF method is then used to determine the fault type of the feature data. The accuracy of this diagnosis method has been proved through comparative experiments.
更多查看译文
关键词
Kernel principal component analysis,Random forest,Tennessee-Eastman process,Fault diagnosis
AI 理解论文
溯源树
样例
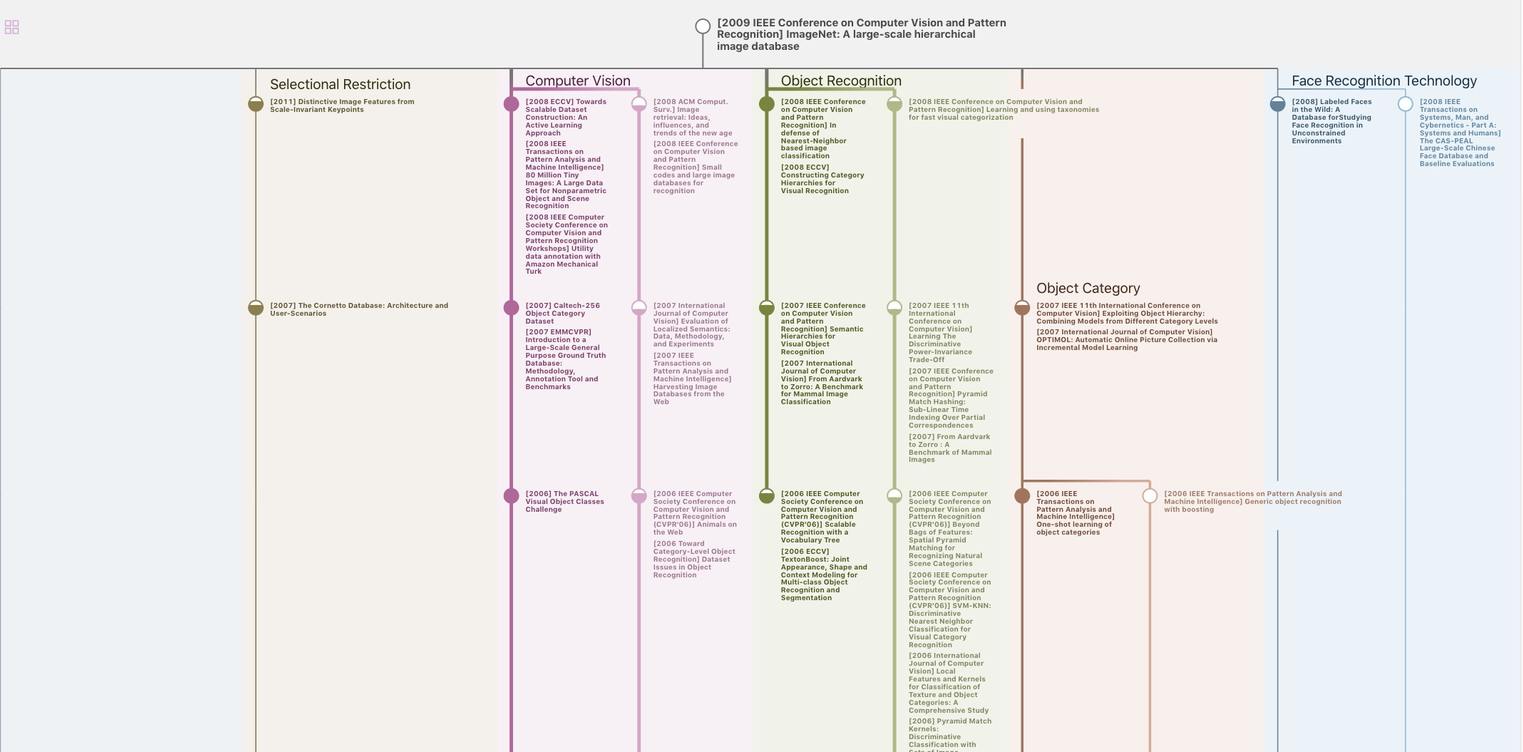
生成溯源树,研究论文发展脉络
Chat Paper
正在生成论文摘要