Dynamic flexible job shop scheduling algorithm based on deep reinforcement learning
2023 35TH CHINESE CONTROL AND DECISION CONFERENCE, CCDC(2023)
Abstract
The dynamic scheduling problem is a hot topic of current research. To solve the dynamic flexible job shop scheduling problem, an improved composite scheduling rule algorithm based on proximal policy optimization is proposed with the objective of minimizing the total delay time. The algorithm uses seven state features to represent the scheduling environment and designs six custom composite scheduling rules and a reward function. The algorithm is able to continuously interact with the environment to accumulate data and update the neural network parameters using Adam's algorithm through offline learning. Simulation results show that the algorithm can achieve better performance metrics by using a combination of single scheduling rules, and the results are better compared to classical scheduling algorithms.
MoreTranslated text
Key words
proximal policy optimization algorithm,deep reinforcement learning,dispatching rules,flexible job shop scheduling
AI Read Science
Must-Reading Tree
Example
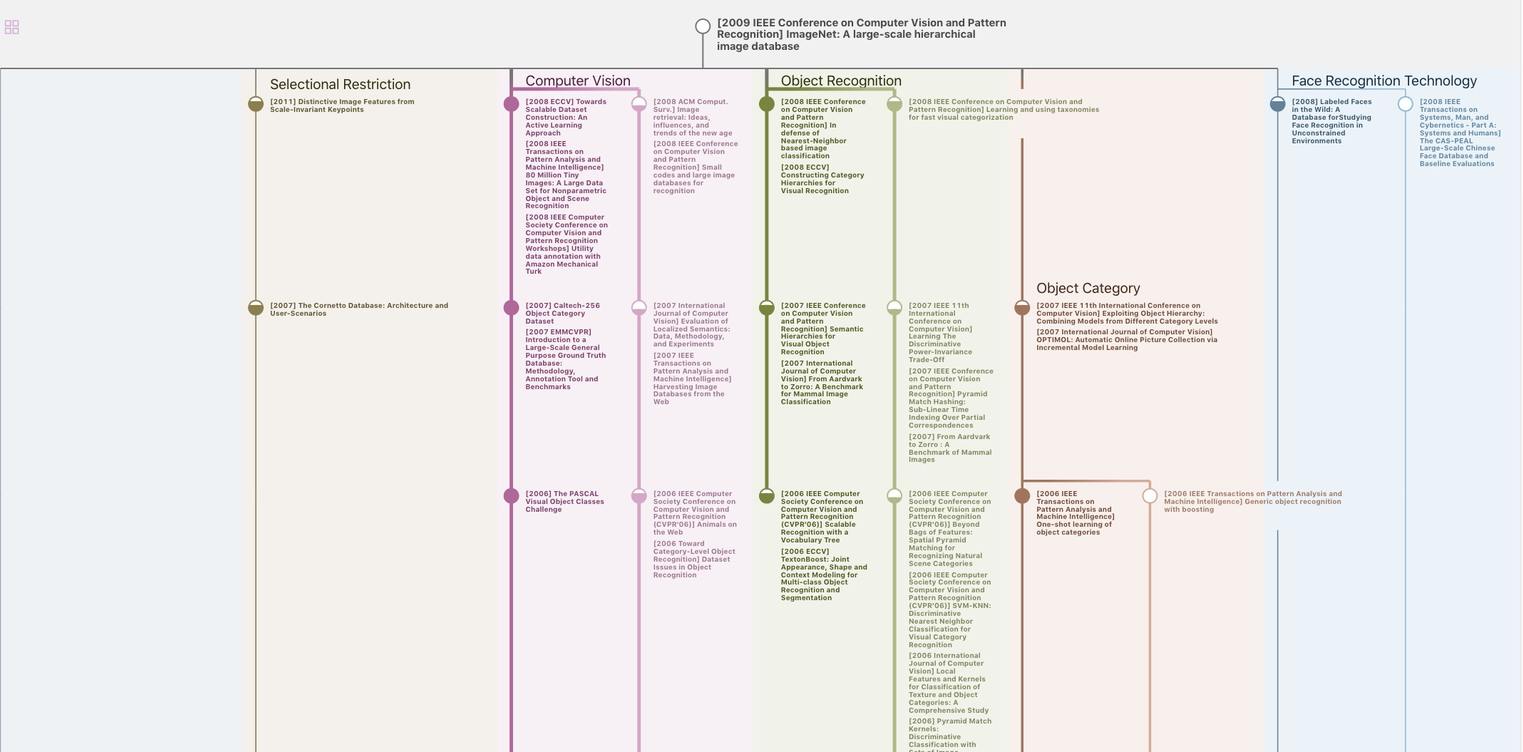
Generate MRT to find the research sequence of this paper
Chat Paper
Summary is being generated by the instructions you defined