Detecting Fraudulent Wallets in Ethereum Blockchain Combining Supervised and Unsupervised Techniques - Using Autoencoders and XGboost.
BLOCKCHAIN(2023)
关键词
Blockchain,Boosting,Fraud Detection
AI 理解论文
溯源树
样例
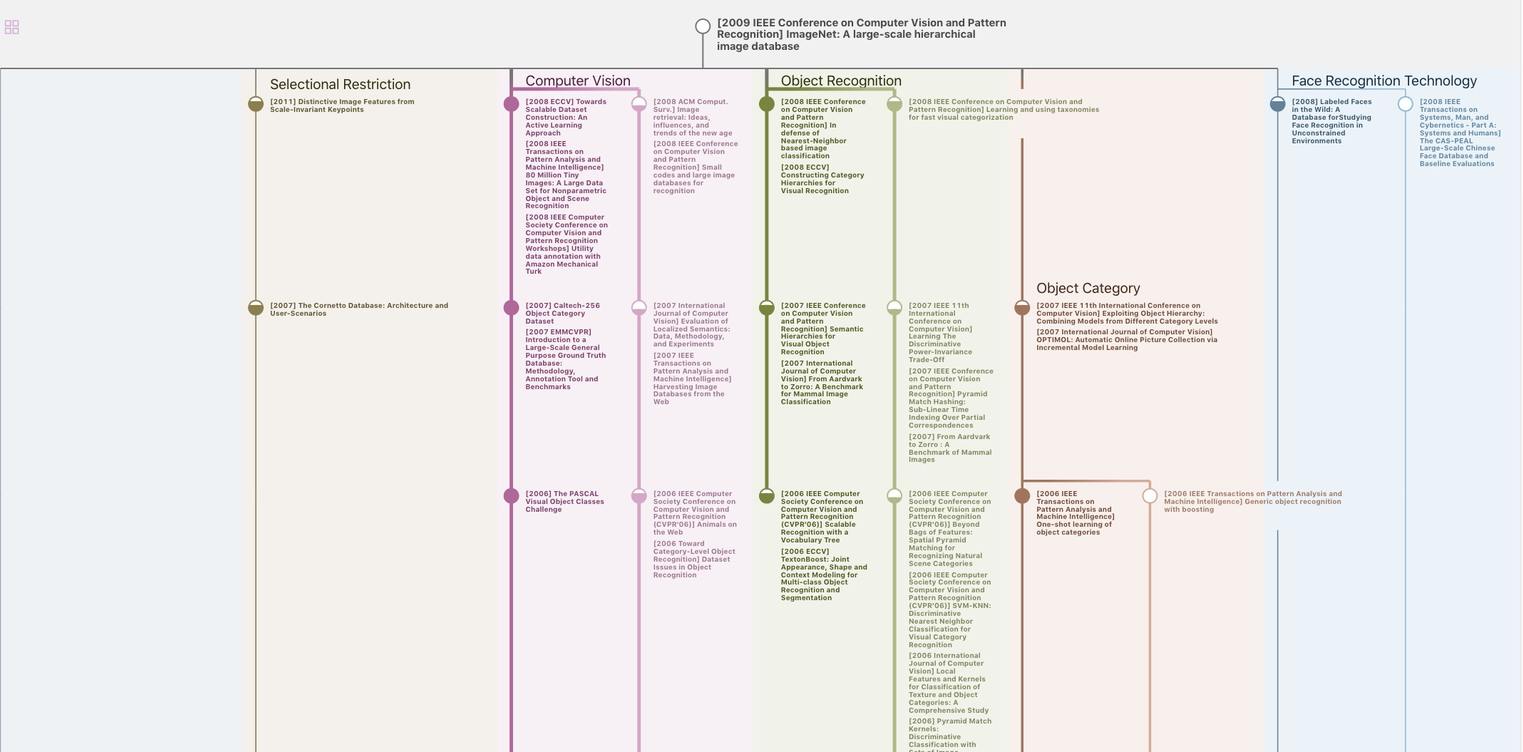
生成溯源树,研究论文发展脉络
Chat Paper
正在生成论文摘要
BLOCKCHAIN(2023)