Explaining a Staff Rostering Problem by Mining Trajectory Variance Structures
ARTIFICIAL INTELLIGENCE XL, AI 2023(2023)
摘要
The use of Artificial Intelligence-driven solutions in domains involving end-user interaction and cooperation has been continually growing. This has also lead to an increasing need to communicate crucial information to end-users about algorithm behaviour and the quality of solutions. In this paper, we apply our method of search trajectory mining through decomposition to the solutions created by a Genetic Algorithma non-deterministic, population-based metaheuristic. We complement this method with the use of One-Way ANOVA statistical testing to help identify explanatory features found in the search trajectories-subsets of the set of optimization variables having both high and low influence on the search behaviour of the GA and solution quality. This allows us to highlight these to an end-user to allow for greater flexibility in solution selection. We demonstrate the techniques on a real-world staff rostering problem and show how, together, they identify the personnel who are critical to the optimality of the rosters being created.
更多查看译文
关键词
Evolutionary Algorithms,PCA,Explainability,Population Diversity
AI 理解论文
溯源树
样例
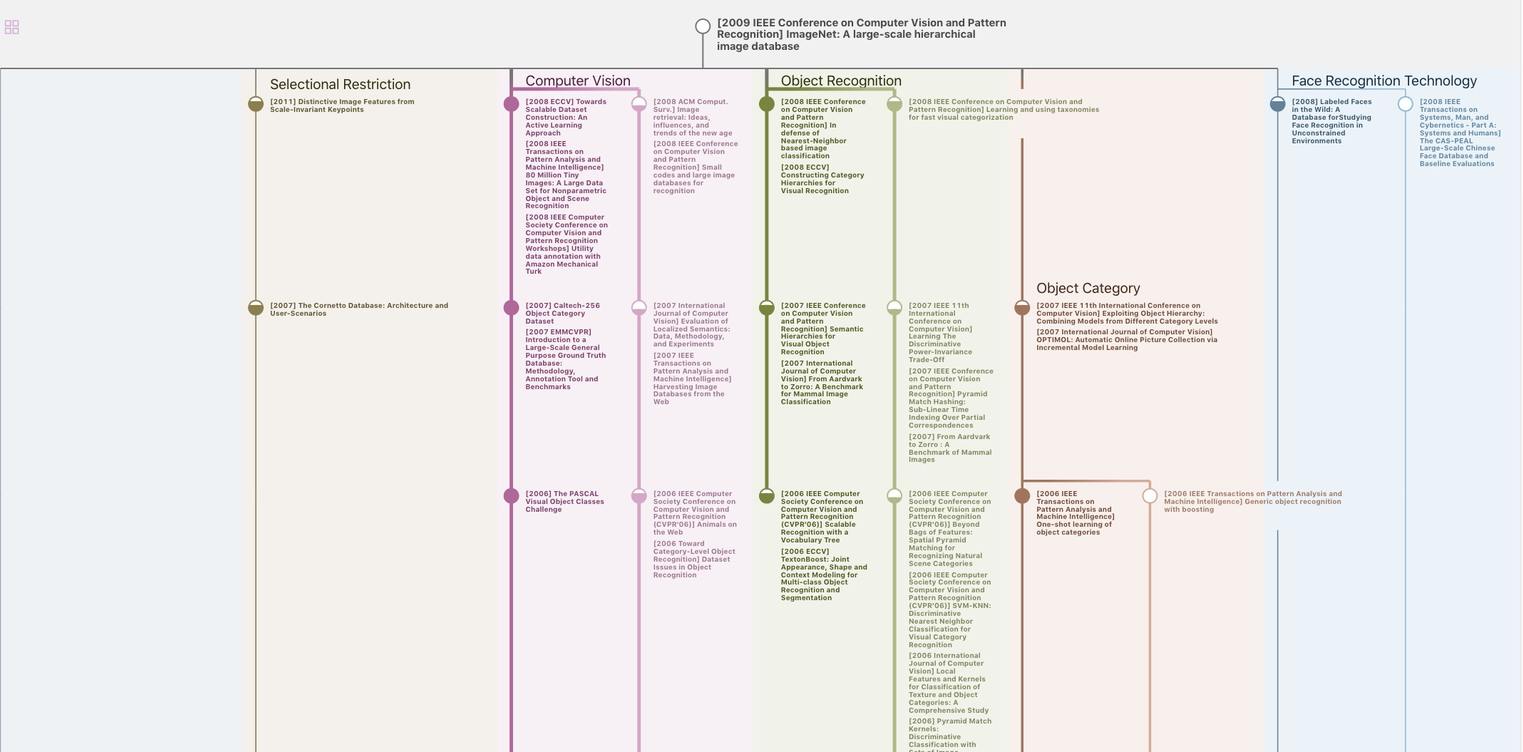
生成溯源树,研究论文发展脉络
Chat Paper
正在生成论文摘要