Enhancing Credit Risk Reports Generation using LLMs: An Integration of Bayesian Networks and Labeled Guide Prompting
PROCEEDINGS OF THE 4TH ACM INTERNATIONAL CONFERENCE ON AI IN FINANCE, ICAIF 2023(2023)
摘要
Credit risk analysis is a process that involves a wide range of complex cognitive abilities. Automating the credit risk analysis process using Large Language Models can bring transformative changes to the finance industry, but not without appropriate measures to ensure trustworthy responses. In this work, we propose a novel prompt-engineering method that enhances the ability of Large Language Models to generate reliable credit risk reports - Labeled Guide Prompting (LGP). LGP consists of: (1) providing annotated few-shot examples to the LLM that denote sets of tokens in an exemplary prompt that are of greater importance when generating sets of tokens in the exemplary response and (2) providing text in the prompt that describes the direction, pathways and interactions between variables from a Bayesian network used for credit risk assessment, thus promoting abductive reasoning. Using data from 100 credit applications, we demonstrate that LGP enables LLMs to generate credit risk reports that are preferred by human credit analysts (in 60-90% of cases) over alternative credit risk reports created by their peers in a blind review. Additionally, we found a statistically significant improvement (p-value < 10(-10)) in the insightfulness of the responses generated using LGP when compared to identical prompts without LGP components. We conclude that Labeled Guide Prompting can enhance LLM performance in complex problem-solving tasks, achieving a level of competency comparable to or exceeding human experts.
更多查看译文
关键词
GPT-4,prompt engineering,credit risk report,Bayesian network,labeled guide prompting
AI 理解论文
溯源树
样例
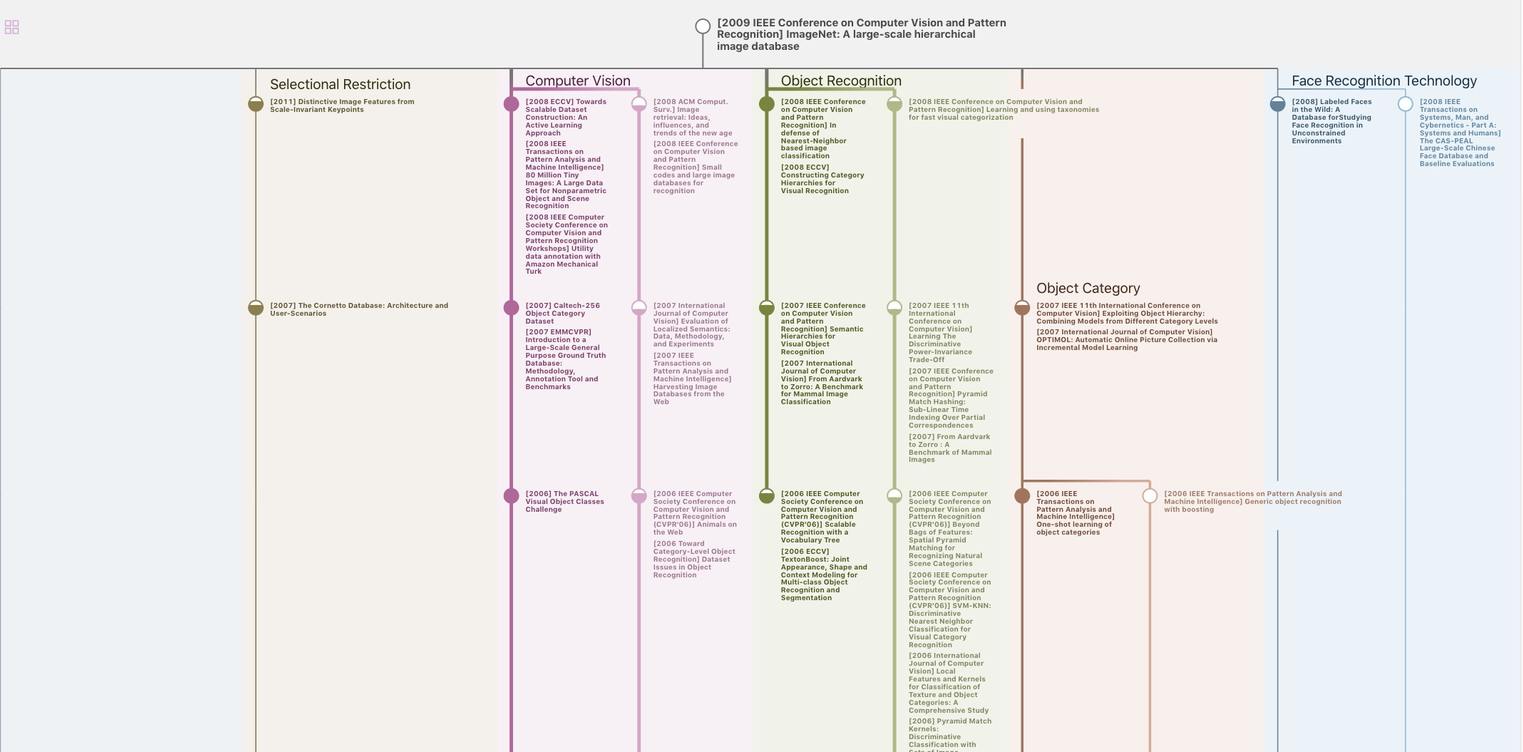
生成溯源树,研究论文发展脉络
Chat Paper
正在生成论文摘要