Dynamic Covariance Estimation under Structural Assumptions via a Joint Optimization Approach
PROCEEDINGS OF THE 4TH ACM INTERNATIONAL CONFERENCE ON AI IN FINANCE, ICAIF 2023(2023)
摘要
Dynamic covariance estimation is a problem of fundamental importance in statistics, econometrics, with important applications in finance, especially portfolio optimization. While there is a large body of work on static covariance estimation, the current literature on dynamic covariance estimation is somewhat limited in comparison. We propose a flexible optimization framework to simultaneously learn covariance matrices across different time periods under suitable structural assumptions on the period-specific covariance matrices and time-varying regularizers. We propose a novel efficient joint optimization algorithm to learn the covariance matrices simultaneously. Our numerical experiments demonstrate the computation improvements of our algorithm over both off-the-shelf solvers and other dynamic covariance estimation methods. We also see notable gains in terms of test MSE and downstream portfolio optimization tasks on both synthetic and real datasets.
更多查看译文
关键词
dynamic covariance matrix,joint optimization,time varying,factor model,sparsity,portfolio optimization
AI 理解论文
溯源树
样例
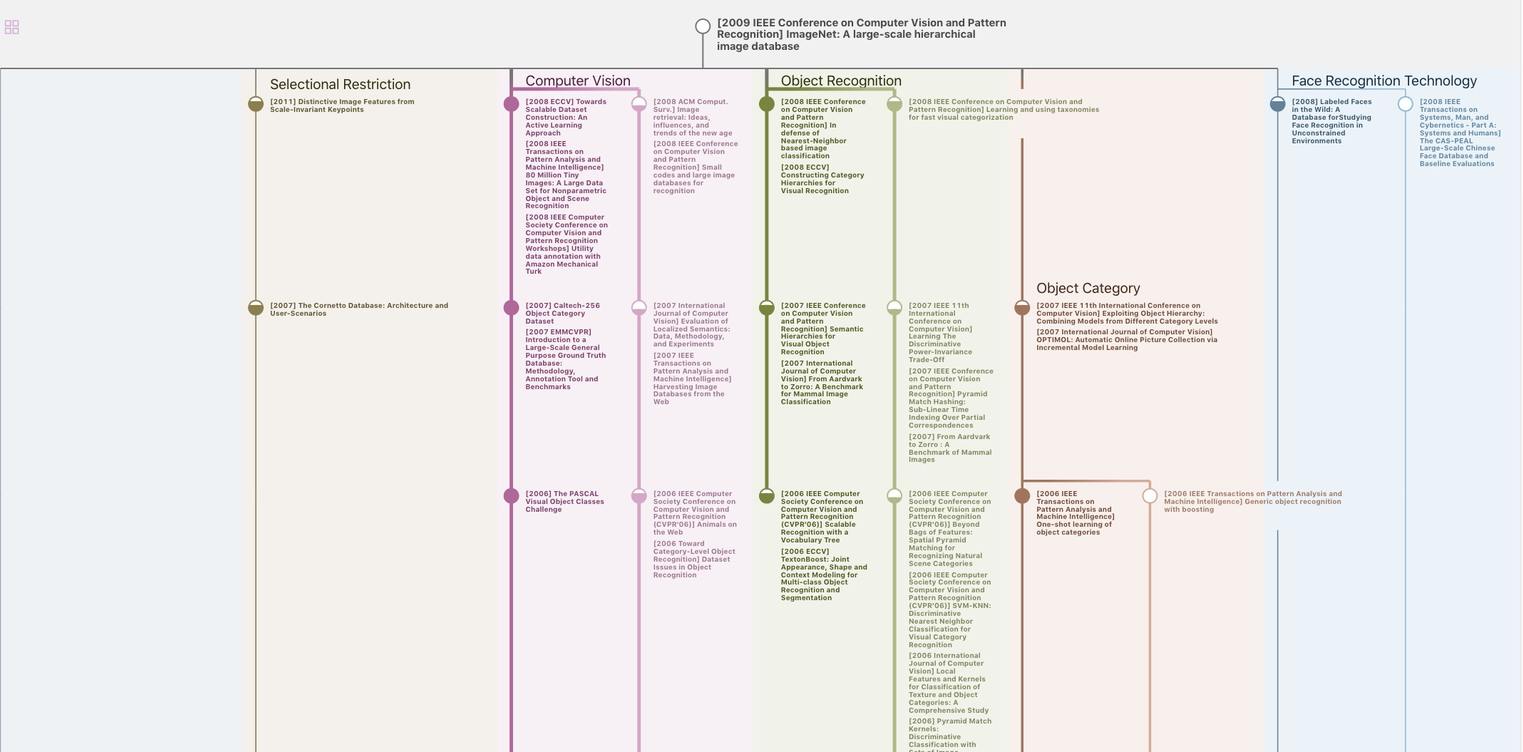
生成溯源树,研究论文发展脉络
Chat Paper
正在生成论文摘要