Feature Fool Exploitation for Lightweight Anomaly Detection in Respiratory Sound.
FDSE(2023)
摘要
Respiratory sound auscultation with digital stethoscopes is a common technique for identifying lung disorders, however, it requires a qualified medical expert to interpret the sounds, and inter-listener variability results from subjectivity in interpretations. To improve diagnostic accuracy and enhance patient treatment, there is a growing need for automated detection of lung diseases. Deep neural networks (DNNs) have demonstrated substantial potential in addressing such challenges. However, DNNs demand a significant amount of data, and the largest available respiratory dataset, ICBHI, comprises only 6898 breathing cycles, which is insufficient to train a satisfactory DNN model. To address the issue, we propose a robust and lightweight model that employs a feature fool exploitation technique to identify respiratory anomalies. Next, we deploy two evaluation approaches to evaluate its performance: random 60/40 splitting and 5-fold cross-validation, against state-of-the-art methods, using the ICBHI dataset. Remarkably, our scheme outperforms existing approaches up to 18.26%, achieving impressive accuracy rates of 72.36% and 89.46%, using the two respective train/test splitting methods. The results show a significant improvement from our method over existing approaches, suggesting its promise for future respiratory healthcare technology research.
更多查看译文
AI 理解论文
溯源树
样例
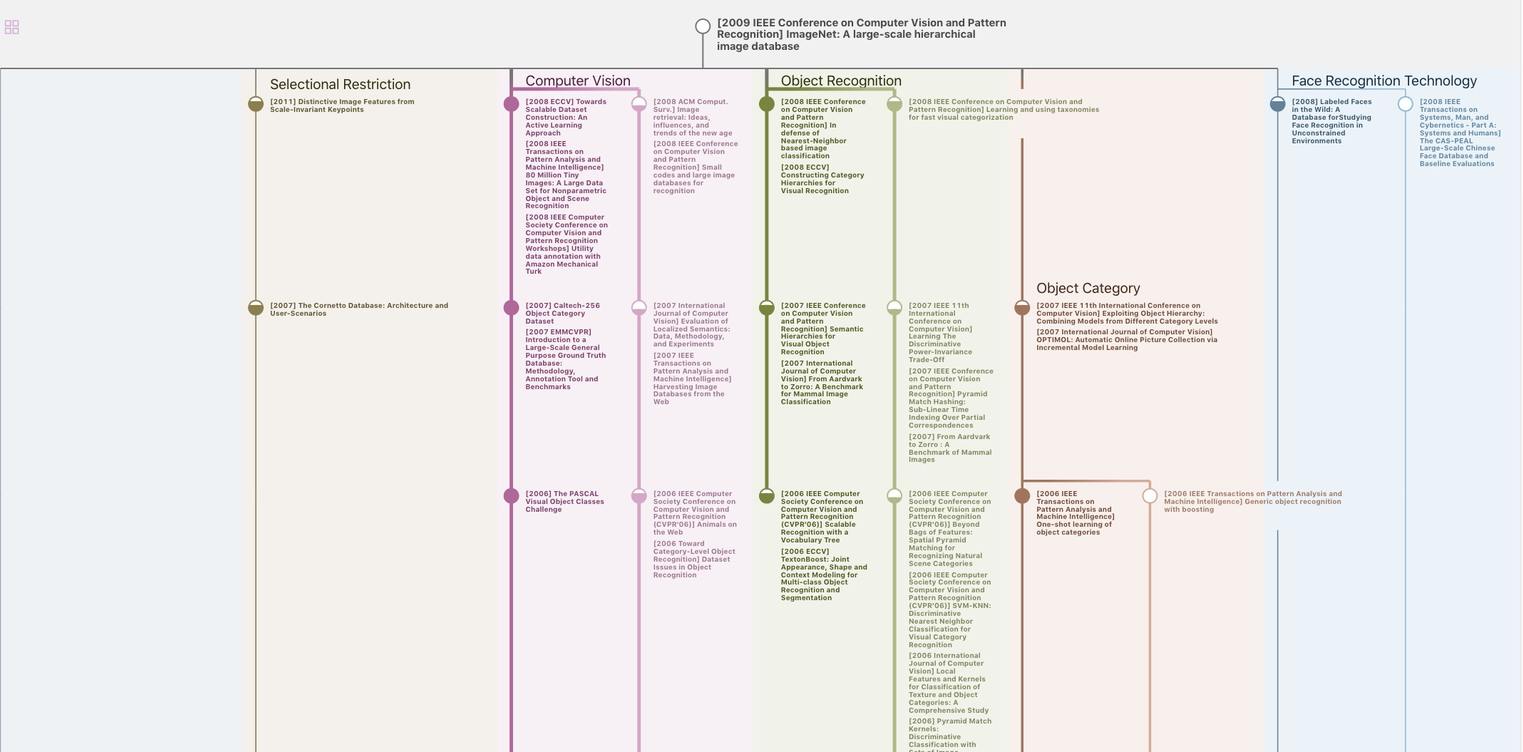
生成溯源树,研究论文发展脉络
Chat Paper
正在生成论文摘要