Ensemble Learning Based Employment Recommendation Under Interaction Sparsity for College Students.
ADMA (2)(2023)
摘要
Recommendation systems play a crucial role in helping college students find job opportunities. However, the sparsity of interactions in employment recommendation for college students poses a challenge for models based on historical user preferences. To address this issue, we propose a novel model called Ensemble Learning based Employment Recommendation under Interaction Sparsity for College Students (EERIS). The model comprises two components: a similarity information component that uses pooled users to determine the nearest neighbor in user similarity measurement, and a global interaction component that uses interaction vectors of user groups to enhance interactions. To evaluate the missing interactions, we propose a loss function called CellLoss. These components are combined based on ensemble learning to improve the model’s generalization and scalability. Our experiments on two real-world datasets demonstrate the superior performance of the EERIS model. Ablation experiments further confirm that each component positively contributes to the model’s performance. Additionally, we design a revised metric for better model testing. Overall, the proposed EERIS model effectively addresses the interaction sparsity in employment recommendation for college students and provides satisfactory recommendations to students.
更多查看译文
关键词
ensemble,interaction sparsity,employment,college students,learning
AI 理解论文
溯源树
样例
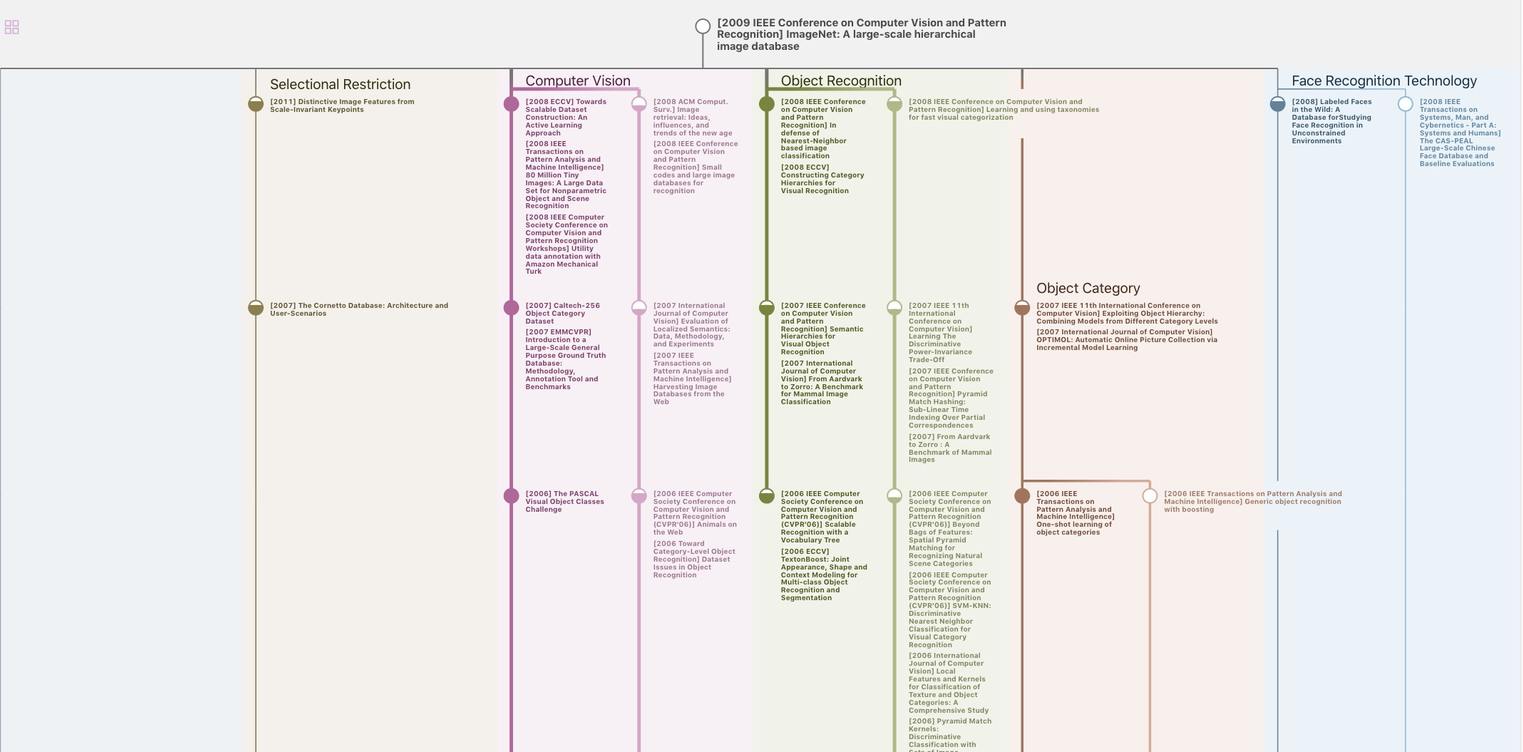
生成溯源树,研究论文发展脉络
Chat Paper
正在生成论文摘要